PART TWO—
HUMANS AND MACHINES
Seven—
Introduction
James J. Sheehan
There has always been a metaphorical connection between technology and nature. People have often looked to the natural world for images to help them understand social, political, and cultural developments, just as they have often imposed on nature images taken from human affairs. The character of natural and mechanical metaphors has changed over time. The boundary between nature and machine has sometimes been redrawn. But it is only recently that we have been able to imagine machines complex enough to be like humans and humans predictable enough to be like machines.
In the ancient and medieval worlds, what David Bolter calls the "defining technology" was not a mechanical device but rather some fairly simple form of manual production. When Plato looked for a metaphor to describe the way the universe functions, he spoke of a "spindle of necessity" with which the fates spin human destiny into the world. In the Timaeus , he compared the creator of the universe to a carpenter and a potter. Only in the seventeenth century, with the rise of a new cosmology, did the most important technological metaphors become mechanical rather than manual. The clock replaced the carpenter's lathe or the potter's wheel as a way of understanding how the universe operated. Like a clock, the universe was thought to be at once complex and comprehensible, a mechanism set in motion by a divine maker who, quite unlike the potter at his wheel, gave his creation the power to operate without his constant supervision.[1]
Just as the Greeks sometimes imagined that the gods had made living things from clay, so people in the mechanical age thought that some living things were no more than complex machines. This view was given
its most influential formulation by Descartes, who stands at the intersection of humanity's changing relationship to animals and machines. As we saw in the introduction to Part I, Humans and Animals, Descartes believed that animals were merely automata made of flesh. Even human activities that were not guided by reason could be seen as mechanical: "the beating of the heart, the digestion of our food, nutrition, respiration when we are asleep, and even walking, singing, and similar acts when we are awake, if performed without the mind attending to them." Such opinions would not, Descartes continued, "seem strange to those who know how many different automata or moving machines can be made by the industry of man." But, of course, Descartes did not think that humans were merely mechanical. The possession of reason opened a fundamental gap between humanity and machines—as well as between humanity and animals.[2]
In the eighteenth century, at the same time that some thinkers had begun to wonder if the gap between humans and animals was as fundamental as Descartes insisted, others called into question the basic distinction between humans and machines. The classic expression of this position was L'Homme machine , published by Julien Offray de La Mettrie in 1747. As his title suggests, La Mettrie believed that Humans were no less machinelike than animals. "From animals to men," he wrote, "the transition is not extraordinary." In blunt, provocative language, La Mettrie argued that mind and body were equally mechanical: "the brain has muscles to think, as the legs have to walk." But he did not have much to say about how mental mechanisms actually worked. His epistemology, which emphasized the power of imagination, had little connection with his notion of brain muscles. In Effect. L'Homme machine was what one scholar has called "a picturable analogy" of the mind, a "modus cognoscendi , desiged to promote scientific inquiry, inquiry, rather than any ultimate knowledge about the nature of things."[3]
In the nineteenth century, a great deal was learned about the body's physiological mechanisms. Research on chemistry and biology enabled scientists to understand how the body functioned like a steam engine—the machine that had replaced the clock as the era's "defining technology." Researchers believed that this knowledge of physiological mechanisms was part of a single scientific enterprise that would embrace the study of all matter, living and monliving. "Physilogy," Wilhelm Wundt maintained, "thus appears as a branch of applied physics, its problems being a reduction of vital phenomena to general physical laws, and thus ultimately to the fundamental laws of mechanics." Such laws, some maintained, explained mental no less than physical phenomena. Edward Youmans, for example, an American disciple of Herbert Spencer, re-
garded the First Law of Thermodynamics as extending from the farthest galaxies into the hidden recesses of the mind:
Star and nerve-tissue are parts of the system—stellar and nervous forces are correlated. Nay more; sensation awakens thought and kindles emotion, so that this wondrous dynamic chain binds into living unity the realms of matter and mind through measureless amplitudes of space and time.
The chain of being, once a hierarchy of existence forged by divine decree, thus becomes a unified domain subject to the same physical laws.[4]
Within certain limits, the nineteenth century's mechnical metaphors were useful tools for understanding physiological phenomena like respiration and digestion. But these metaphors worked much less well when applied to the mind. To be sure, experimental psychologists learned to measure some kind of perceptions and responses, and neurologists did important work on the structure of the brain; but it was difficult to imagine mechanisms complex and versatile enough to approximate mental activities. Nor could anyone come close to building a machine that could perform anything but the most rudimentary calculations. In the 1830s, for example, Charles Babbage failed in the attempt to manufacture an "analytical engine" that would be able to "think" mathematically. Because he did not have the technologicl means to perform the functions he had ingeniously contrived, Babbage remained "a brilliant aberration, a prophet of the electronic age in the heyday of the steam engine."[5]
Until well into the twentieth century, those who believed that the brain functioned mechanically had great difficulty describing just how these mental mechanisms worked. One product of these difficulties we behavioralism, perhaps the dominant school of empirical psychology in the early twentieth century, which denied that the nature of mental states was knowable and concentrated instead on studying behavioral responses to stimuli. In the 1920s, when a new controversy erupted over the concept of "l'homme machine," it was fought out on largely biological rather than psychologicla grounds. Joseph Needham, who took the La Mettriean position in this debate, acknowledged that this mechanistic view of human nature was no more than a "methodological fiction," even though he believed that "in science, man is a machine; or if he is not, then he is nothing at all." Given the sort of machines that Needham could imagine in 1928, it is not surprising that he could not find one that much resembled the mental world of human beings.[6]
All this changed with the coming of the computer. The theoretical and technological basis for he computer was laid in the 1930s by Alan Turing and others, but it was only after the Second World War that these machines moved from the realm of highly technical speculation to the
center of both scientific research and popular culture. Computers, unlike the crude calculating machines of the past, seemed fast, complex, and supple neough to approximate real thought. The gap between mind and machine seemed to be narrowing. For example, in 1949, Norbert Wiener published his influential work on "Cybernetics," which he defined as "the entire field of control and communication theory, whether in the machine or in the animal." That same year, John von Neumann, who had built a rudimentary computer in Princeton, pointed out the similarities between the computer and the brain. Two years later, in an essay entitled "Computing Machinery and Intelligence," Turing predicted tht within fifty years there would be machines that could perfectly imitate human intelligence.[7]
So swiftly did the use of computers spread that by the 1960s, they had become our "defining technology," as fundamental to the way we view ourselves and our worlds as Plato's crafts or Descartes's clockwork mechanisms had been to theirs. We now live in the era of "Turing's Man," whose relationship with machines Bolter summarizes in the following telling phrase: "By making a machine think as a man, man re-creates himself, defines himself as a machine."[8]
Computers played an essential role in the formulation of cognitive science, which Howard Gardner defines as the "empirically based effort to answer long-standin epistemological questions—particularly those concerned with the nature of knowledge, its components, its sources, its development, and its deployment." In the last two decades, this way of looking at the mind has tended to replace behavioralism as the most vigorous branch of empirical psychology. In the light of what computer scientists claimed about the way their machines worked, the behavioralists' self-imposed refusal to talk about mental states no longer seemed either necessary or desirable. As George Miller, who observed the shift away from behavioralism, recalled,
The engineers showed us how to build a machine that has memory, a machine that has purpose, a machine that plays chess, a machine that can detect signals in the presence of noise, and so on. if they can do that, then the kind of things they say about machines, a psychologist should be permitted to say about a human being.
Cognitive scientists insisted that they could open the so-called black box, which behavioralists believed concealed the mental connection between stimulus and response Within this box, many were now convinced, they would find something very much like a computer.[9]
Research on what came to be known as "artificial intelligence" began during the exciting early days of the computer revolution, when the technology's potential seemed without limit. The following statement,
from an article published in 1958 by Herbert Simon and Allen Newell, provides a good example of the style and tone with which the new field was announced:
It is not my aim to surprise or shock you. . . . But the simplest way I can summarize is to say that there are now in the world machines that think, that learn and that create. Moreover, thier ability to do these things is going to increase rapidly until—in a visible future—the range of problems they can handle will be coextensive with the range to which the human mind has been applied.
La Mettrie had declared that humans were machines but could say little about how these machiens worked. Nineteenth-century physiologists could imagine the human body as a "hea engine," which converted fuel into growth and activities. To the advocates of artificial intelligence, the computer finally provided a mechanism comparable to the mind: to them, "intelligent beings are semantic engines— in other words, automatic formal systems with interpretations under which they consistently make sense." Marvin Minsky put the matter much less elegantly when, in what would become an infamous phrase, he defined the mind as "meat machine."[10]
Artificial intelligence draws on several disciplines and contains a variety of different elements. It is, in the words of one of its practitioners, "a field renowned for its lack of consensus on fundamental issues." For our purposes, two intradisciplinary divisions seem especially important. The first is the difference between "strong" and "weak" artificial intelligence. The former (vigorously examplified by Newell's essay in this volume) argues for the potential identity of mind and machine; the latter is content with seeing computers as models or metaphors for certain kinds of mental activities. The second division is rather more complex since it involves two different ways of thinking about and building intelligent machines. The one (and again, Newell is a fine example) seeks to define a sequential set of rules through which the computer can approximate—or duplicate—the workings of the mind. This perspective, which draws its philosophical inspiration from Descartes's belief in the possibility of defining certain rules of thought, builds on concepts first developed by Turing and von neumann. Sherry Turkle calls the other approach to artificial intelligence "emergent AI," which includes "connectionist" models as well as models based on the idea of a "society of mind." Modeled on assumptions about neural operations in the brain, this approach emphasizes parallel operations rather than sequences, the storing and manipulation of individual pieces of information rather than the formulation of general rules. Whiel both branches of artificial intelligence developed at about the same time, the former became dominant in the 1970s and
early 1980s, while the latter seems to have become increasingly influential after 1984.[11]
Like sociobiology, artificial intelligence has been attacked from several directions—as philosophically naive, methodologically careless, and politically dangerous. Terry Winograd's essay suggests some of these criticisms, to which we will return in the conclusion. Critics like Winograd argue that fulfilling the promises made during the early days of cognitive science has turned out to be much more difficult than Turing and his contemporaries had believed. Changing the scale and broadening the scope of what a computer cna do have involved conceptual nd technological problems few of the pioneers envisioned. Nevertheless, no one doubts that computer scientists have made great progress on a number of fronts. Moreover, given the unpredictable course of scientific progress, it is hard to say what is and what is not possible. After all, as two scientists have recently written, computer experts have "the whole future" in which to show that intelligent mchines can be created. Doubts about the feasibility of artificial intelligence, therefore, can lead to skepticism, not categorical rejection.[12]
A categorical denial that it will ever be possible to build machines that can think like humans can rest on two foundations. The first requires a belief in some spiritual attribute that separates humans from machines—and in Western culture at least, from animals as well. The second is based not on our possession of a soul but rather of a body: according to this line of argument, which is suggested in Stuart Hampshire's concluding remarks to this section, being human is inseparable from the feelings and perceptions that come from our physical existece. Without that knowledge of our own birth and death, a knowledge central to our conceptions of past, present, and future, any intelligence, no matter how skilled at solving certain sorts of problems, must remain essentially and unalterably different from our own. Melvin Konner makes this point by comparing the machines of the future to the gods of ancient Greece: "incredibly powerful and even capable of many human emotions—not because of their immortality, ineligible for admission into that warm circle of sympathy reserved exclusively for humans."[13]
Eight—
The Meaning of the Mechanistic Age
Roger Hahan
The late Eduard J. Dijksterhis of Utrecht coined the term "Mechanization of the World Picture" to characterize the nature of the intellectual revolution that emerged triumphant in the seventeenth century. In his pathbreaking work, written close to forty years ago, he repeatedly refuses to provide a static definition for his central conception, choosing instead to describe it by showing how it emerged from a rediscovery and recombination of certain key strands of Greek natural philosophy in the late Renaissance and how it was centrally shaped by the success of Galileo and Kepler's new astronomy, Descartes's and Huygens's new philosophy of physics, and newton's grand synthesis at the end of the century.
Conceptions of the world picture since the seventeeth century have admittedly never been the same. The Aristotelian universe of essential qualities and goal-directed forms apparently was displaced by the new mechanistic philosophy. For the last forty years, that transformation, known to historians by the shorthand label of the Scientific Revolution, has been chronicled, its origins hve been established, and its character has been the subject of dozens of major monographs. Curiously enough, the issues Dijksterhuis broached but refused to settle have never been fully analyzed from a modern perspective. My concern is to offer some suggestions for finding a meaning for this Mechanistic Age which rests on historically sound grounds. That meaning requires special attention because "mechanistic" is the common epithet hurled at "reductionists." They are often crudely charged with a desire to eliminate the study of living beings by grounding them exclusively on the physical sciences. If we proceed with a historical analysis, we will see that the label "mechanistic" carries with it other substantial implications.
Perhaps the biggest obstacle to arriving at a full appreciation of the
notion is in fact for us to remain captive of too strict a chronologicla view. Historians of science—and I must include myself among the guilty ones—have, through their decades of refining the meaning and arguing about the temporal limits of the Scientific Revolution, locked themselves into a much too narrow identification of the Mechanistic Age with this movement. It behooves us first to clear away these constricting and ultimately misleading views of the Sicnetific Revolution to permit reaching for a more encompassing meaning.
The current notion of the Scientific Revolution, though it has a history that would take us back to the eighteenth century, is in fact the by-product of a set of lectures given at Cambridge in 1948 by the late Sir Herbert Butterfield, eventually published under the title, The Origins of Modern Science . That notion was successfully elaborated by a group of major scholars that includes the aforementioned Dijksterhuis and my teachers and colleagues Alexandre Koyré, Marie Boas, her husband, A. Rupert Hall, Paolo Casini, I. Bernard Cohen, Thomas Kuhn, and most recently, Richard S. Westfall. They and their followers all suffer from a common historic myopia, namely, the unitary identification of the momentous Revolution with the "Mechanical Philosophy" and the conviction that its culmination came in the person of Sir Isaac Newton, whose Philosophiae Naturalis Principia Mathematica is justly hailed. As a consequence of this shortsighted view, historians have too rigidly equated the Scientific Revolution with the advent of a Mechanistic Age and struggled to confine its development to the seventeenth century, principally in an English context appropriate to Sir Isaac.
An oversimplified view of their argument runs somewhat as follows. Following on the almost simulatneous discovery in 1609 by Kepler of the ellipticity of planetary orbits and by Galileo of the physical imperfections of celestial bodies like the Moon and Jupiter seen in his telescope, progressive natural philosophers were forced one by one to abandon Aristotelian and Ptolemaic dicta concerning the cosmos. In the next decades, the old order was shattered beyond repair by a flood of opinions that included a devastating critique of Peripatetic concepts about the closed universe structured around a central point and its attendant terrestrial physics based on the idea that motion was determined by essential qualities residing in substance. The separation of the cosmos into a world of heavenly perfection and earthly imperfection which mirrored Christian beliefs, and of the correspondence between the microcosm of man and the macrocosm of the universe, was recognized as obsolete and jettisoned as misleading. Critical philosophers like Bacon and Descartes ridiculed assumptions abou tthe erroneous grounds on which the older epistemology was based and began to proclaim a new philosophic order.
By the 1630s, its building blocks had been individually assembled: a
new philosophy o mathematical cogency, often coupled with experimental confirmation; the postulation of a corpuscular ontology that focused on quantifiable elements and mechanisms immediately apprehended by the eye and the mind; infinite, isotropic space that was indifferent to the nature of the objects placed in it and hence stripped of hierarchical values taken for granted by traditional religious authorities. By the end of the seventeenth century, so the official line runs, all these pieces were brilliantly assembled into the Newtonian system of the world with its universal laws of motion, its new metaphysic of inert matter, absolute space and time, and the proclamation of a new progressive epistemology of the mathematical and experimental natural philsophy. Moreover, it is pointed out, Newton found it possible to incorporate a modified set of Christian values about the Lord into his mechanical and corpuscular system so that it would continue to reflect the harmony and power of the deity in the cosmos. Sir Isaac returned the favor of his prophetic birth on Christmas Day, 1642, by lending his genius to the Almighty to "save" the cosmos for Christianity. You will surely recall Pope's famous couplet that expresses the blessings Western Civilization received when "Nature and nature's laws hid in night: / God said, let Newton be! and all was Light."
What is wrong with this interpretation from our perspective? It evades larger historic issues by giving a narrow, temporal, and geographic location to the Scientific Revolution, assuming it to coincide with the process of the mechanizationof the cosmos. Ideas as profound and important as mechanisms have both roots and consequences that cannot be confined to seventeenth-century England. In saying this, I do not advocate the fashioning of a counterthesis, merely the decoupling of the concepts. Today aswell as in the seventeenth century, in the world of Western civilization, whether it is found in Israel, Japan, or Merry Old England, we mainly operate with the assumptions of the Mechanistic Age. let me elaborate.
The notion of the mechanical involves quite a few dimensions that, for the sake of analysis, I would like to display singly, keeping in mind that they are hisotirclaly and conceptually interlinked.
First is the machine itself, with its internal workings displayed for all to apprehend. While it was principally articulated after the invention and with the help of printing, the machine was no newcomer to the Renaissance. Every civilization fashions more or less elaborate tools to cope with nature, but few had given them such a special place as did the Hellenistic Greeks and their Roman followers. Engines of war and construction were at first merely described with words and their principles laid down using terminology common to natural philosophers by major figures like Archimedes. Already in the third century B.C., it ws aximomatic that their functioning was based on a geometric undersanding of physical princi-
ples, in which number, proportion, size, and configuration were critical and for which the strength of materials and thermal forces responsible for expansion nd contraction provided an essential explanatory component. Simple laws of leverage and the schemes for multiplying mechanical advantages by the use of the wedge, pulleys, and gears were well understood by engineers like Philo of Byzantium and Hero of Alexandria who used them to make sense of the common machines of antiquity.
But the Greeks also developed mechanical principles to serve quite different ends. They fashioned automata, that is, self-moving objects dressed up to resemble and imiate the operations generally ascribed to willful agents. One finds described singing birds activated by water pressure, temple doors that open in response to the lighting of fires, puppet shows representating shipyard workers, and mixing vessels that seem to be filled mysteriously as they are visibly being emptied. Such elaborate toys, popular in the Byzantine world, built to astound the spectator rather than enlighten him, are a testimony of the genius of ancient crafsmen.[1] But they also testify to the ancients' use of machines to glorify their own ability to penetrate nature's secrets and to mystify the gullible public by the fashioning of mirabilia . They are part of an ancient and tenacious thaumaturgic tradition. All themechanisms to produce these effects are carefully hidden from view so as to increase the sense of the marvelous.
In ancient times, such automata were not built as mechanical models to explain living beings; they existed merely to imitate nature they way that an ancient artist would strive to copy and reproduce natural occurrences. A measure of the prowess of the artisan was his ability to reproduce in the most faithful way possible the natural processes we experience. Prior to the advent of Christianity, nature was worshiped because it encompassed such wonders; man's accomplishments would be measured by his ability to mimic life, not to make it serve his secular purposes. In the rare instances we know about the Greek mechanist was proud to have outdone nature by feats of trickery, often executed by use of mirrors, by which the right and left might be transposed from their natural order or by which small objects might be magnified by placing them under a distorting glass object.
What differentiates this use of the mechanical artifact from the post-Ranaissance version is the emphasis on secret processes, the reveling in the mysterious ways of nature. Mysterious at least to the public! The treatises from which we garner this picture of the Greek notion of machine were meant for the engineers, the few in society adept at understanding and imitating the cosmos. The mechanicians of ancient times were in this sense askin to the alchemists: by the use of secret means, the alchemist had meant to reproduce in the laboratory the complex procedures found in nature to grow metals in the telluric womb. The alchemi-
cal mahatma was the one who could imitate nature and perhaps speed up its natural processes. Man might coax nature along, but he never meant to dominate it.
The picture changes markedly as these ancient treatises are rediscovered and edited in the renaissance, Hero, for example, in 1575. The spellbinding character of the mechanical contrivances is embraced by enthusiastis like Della Porta as part of the magical tradition, known to contemporaries as the secrets of nature. Knowledge about them was shared among the adept as an initiation rite for entrance into his Neapolitan Accademia Secretorum Naturae . But alongside the older approach to machines, a new tradition had already found favor with the other, more practically minded aritsans. Agricola, for example, despite his humanistic training, focused on the actual descriptin of machines employed by miners in his De Re Metallica (1556). Like his contemporaries, Biringuccio and Ercker, he took his mission to be to assemble a handbook of mining based directly on practices he observed in Saxon mines, and he strove to make them immediately accessible, though in the language of Columella and in the accompanying illustrations. He was not alone in this genre. Indeed, so many texts describing the arts of sailing, cartography, architecture, fortifications, gunnery, hydraulics, bridge building, and so on, often presented in the vernacular and accompanied by numerous diagrams, were published in the late sixteenth century that they provoked the compilation of encyclopedic treatises that became an immediate printing success. Jacques Besson's Theatre des instrumens mathematiques et mechaniques (1578), Agostino Ramelli's Le Diverse e artificiose machine (1588), and Vittorio Zonca's Novo teatro di machine et edificii (1607) all have in common the exaltation of machines as human inventions, downplaying the notion of artisans as mere imitators of nature. These compilers share the goal of spreading new knowledge to the multitudes. Evne the illiterate artisans were permitted to profit from them, merely by studying the sumptuous illustrations.
Machines were now directly linked to the expectation of progress through mechanical improvement, a phenomenon that was widespread in the increasingly commercial and urbanized society of early modern Europe.[2] The same mood has continued unabated to our present day and is the most important root of the Mechanistic Age in which we operate.
What was gained through this dimension for the making of the Mechanistic Age is not just publicity and progress and its concomitants, democratization and the material improvement of society. The visual representation of machines forever stripped them of secret recesses and hidden forces.[3] The learned reader now expected to see for himself how things worked, no longer depending solely on classicla authorities for confirma-
tion. In his preface to De Re Metallica , Agricola assures us he personally went into the mine itself. In 1600, William Gilbert similarly dedicated his De Magnete: "to you alone, true philosophizers, honest men, who seek knowledge not from books only but from things themselves."[4]
The new style of philosophizing, preferring things to books and concrete, visually reliable instances to rhetorical systems of thought, was of course at one with the new experimental philosophy embraced by Galileo, Bacon, and even, to some extent, Descartes. Galileo sets the debates between his interlocutors in his Discorsi in the Venetian Arsenal where deeds speak louder than words. In a clear sense, the theoreticians of the Scientific Revolution borrowed a frame of mind from the artisan traditions displayed in the "theatre of machines" literature. Scientists' new preference for the palpable is evident not only in their prefaces but in their defense of demonstrations and experiments as the new arbiter among competing theories. It is no accident that all of the important discussion groups of the seventeenth century, from Gresham College to the Berlin Academy, owned scientific equipment and hired demonstrators. The tone of the new sciences was to displace the occult by the visible, the mysterious by the palpable.
Here once comes to the second important way by which we may analyze the meanings of the Mechanistic Age. It is characteristics of philosophical discussions of the era to reject occult forces whenever possible and to substitute for them self-evident principles much like one sees when watching a simple machine in operation.
According to the new mechanical philosophers, Aristotelian reliance on essential qualities as the central explanatory device in physics was the major weakness blocking the growth of understanding. To explain change by referring to an immaterial form that "informs" matter was quite unproductive. The charge was advanced, for example, that asserting an object falls to the center of the universe because its essence is heaviness is as illuminating as Molière's medical student who explains the powr of opium to put patients to sleep as the result o a virtus dormitiva (opium is a soporific because it contains a dormitive power).[5] Peripatetic strategy seemed to obfuscate by substituting words for real causes. When asked further why earthy matter was heavy or opium soporific, the answer invariably was circular: because it was "in the nature" of the object to act in this fashion. How could progress ensue when playing such a dead-end rhetorical game?
Historians of the Scientific Revolution customarily claim that the introduction of the mechanical philosophy broke this impasse by providing a solution to this philosophic dilemma. If visible, palpable mechanisms of change were substituted for the hidden, occult forms, the future of natural philsophy might be ensured. And none seemed more intelligi-
ble than ction through direct contact. The immediacy of understanding that comes from watching a stationary billiard ball move when struck by another, or how power is transmitted from one gear to another, or even how blood gushes from an opened artery when the heart contracts had a dramatic impact on the mind confused by Peripatetic verbosity. Even the seemingly complicated functions of animate beings were rendered more understandable by assumptions that their members—the hand or arm, for instance—operated as did machines, with levers, gears, pulleys, and gates, following the commands of the mind.[6] In the late seventeenth and early eighteenth centuries, bodily parts were pictured as individual mechanisms. The structural correspondence between man-made manchines and living entities was adopted because it promised a new degree of intelligibility. The new scientists of the seventeenth century could readily give up on the old metaphysics when they juxtaposed the mechanical world of their era with the scholastic auditorium in universities, one vital, illuminating, and progressive, the other stultifying, fruitless, and tautological.
What principles did they propose instead? The new rule adopted was to ground all explanations on the idea of matter in motion. The corpuscular philosopher, who unashamedly borrowed from ancient atomists, found new psychological satisfaction in reducing the complexity of nature to the simplicity of corpuscles capable of motion and causing change by impact only. To the mind troubled by the old verbiage, they were surely preferable to Peripatetic forms and qualities. For Descartes, the principal systematic proponent of this view, matter was the analogue of extension, in the same way space was defined by the objects that occupied it. Common sense as well as logic showed us with undeniable reality that things are composed of matter and that next to impenetrability, matter was principally characterized by its location. Motion, too, was grounded on irrefutable visible existence. Neither was hidden or occult. Both were, as Descartes enjoyed repeating, clear and distinct and, to his mind, ultimately real. The mechanical philosopher reveled in having found an ontologiclaly satisfying basis for apprehending nature, one that was not merely true by virtue of what the senses and the mind perceived but that could also be manipulated by mathematical symbols rather than scholastic terminology. Adopting the mechanical philosophy brought in its wake the ascendancy of mathematical language that purportedly guaranteed that proper logic would be observed and clarity maintained. It seemed to answer all the criticism laid at the door of the ancients and to offer a hope for depening our understanding.
To a considerable extent, the mechanical philosophy provided a much-desired new ideology that brought success to many seventeenth-century natural philosophers. It had all kinds of advantages. For Des-
cartes, as it had for Galileo earlier and was to have later for Locke, it permitted the separation of primary from secondary causes into the fundamental quantitative and the derivative qualitative realm. As a well-taught paradigm, it served to focus generations of scientists on specific riddles of nature—for example, the nature of the vacuum, the production of colors—that required experimental elucidation. But it would be a gross historical simplification to suppose that this new philosophy solved all the old problems and earned Descartes universal accolaim.
Practitioners of the new philosophy realized all too quickly that Descartes had substituted a new ontology for the old one—the quantifiable for the qualitative—but it was itself metaphysical. Instead of relying directly on tangible experience, it proposed principles, different from those of Aristotle but principles nonetheless. Scholastic debate was by no means absent from discussion centers where Descartes's philosophy was taught and applied. Was extension coterminous with matter? How divisible were corpuscles, and how many kinds of matter were there? How was movement transmitted and its total quantity in the universe to be measured? How could one verify by palpable, visual experiments the submicroscopic explanations for the action of the lodestone, or the production of colors? Leaving aside for a moment the immense problems posed by living matter for Descartes, how could one fully understand the motion of the heavenly bodies in elliptical paths? The new mechanical philosophy seemed to have created as many new problems as it resolved. Some of the solutions offered clearly reintroduced into our language the very occult terms that Descartes and his followers had thought to have repudiated. A major group of thinkers, particularly in England and including Sir Isaac, sought the answer to the mechanical philosopher's weak points in the nature of the deity, or by postulating universal gravitation and short-range affinities of an electric, magnetic, or even alchemical sort. It is particularly because of this historic turn of events that I urge a decoupling of that which has led us too casually to equate the Mechanistic Age with the Scientific Revolution. By following the intellectual path chosen by Newton too exclusively, as most of my colleagues have done, they have made us lose sight of the continuing agenda of those who instituted the Mechanistic Age.
A third and very subtle aspect of the Mechanistic Age was the change in emphasis traditionally give to the notion of causality from what Aristotle called formal and final causes to efficient and material ones. Indeed, many who embarked on this path found themselves holding a nominalist position that went so far as to deny causality altogether—witness David Hume's mid-eighteenth-century critique. The detailed history of this change has yet to be written, but its main stages within the history of science are clearly evident and provide a major ground for later criticism
of the mechanistic philosophy.[7] By diminishing the importance of first causes, or even denying the possibility of reaching them, the mechanistic scientist has abandoned a task natural philosophers originally assumed for themselves: to make complete sense out of the world and to provide an explanation for it by attributing a goal for nature and its creator. The Mechanistic Age completed the process of displacing teleology in favor of a search for laws that link phenomena in regular, repeatable patterns of behavior. Note that the historical trajectory that takes us from Plato to the positivists of the nineteenth century passes through the Scientific Revolution but is not restricted by it. Conversely, all the actors of the Scientific Revolution were not equally committed to the abandonment of final causes. Moreover, leaving out Hume, the main proponents of this constituent lived on the Continent and operated next to the mainstream of the traditional Scientific Revolution: Pascal, Malebranche, d'Alembert, Buffon, Laplace, Bernard, and Du Bois-Reymond. There is insufficient space to carefully map this path, so I will confine myself to illustrating it mainly with Laplace, for it was in his hands that this aspect of the Mechanistic Age found its most assertive and pregnant outcome, the principle of strict mechanical determinism.
As is well known, both Plato and Aristotle drew on telos as the central concept for explaining the world. Not only were structures of living beings best understood through their function but all physical change was expressed in terms of a process analogous to substances fulfilling their natural destiny. Aristotle had specifically singled out the atomists for criticisms because their system left events to chance. For him, all satisfactory explanations required an overdetermined set of causes that left no room for fortune. When atomist notions were refurbished in the seventeenth century and were adopted for the establishment of a cogent mechanical philosophy, the charge of impiety was added to the discomfort evidenced over the belief that all could ultimately be made intelligible by the fortuitous interplay of invisible material elements. But there were devout Christians like Pascal and Malebranche who took refuge in the idea that even though the natural philosopher could never divine the real intentions of God, he could at least note nature's regularities and establish the phenomenal laws under which they operated. Like other nominalists, they radically separated God's omniscience from man's limited abilities to reach essences and to fathom purposes.
It is no accident that such developments took root precisely at the time when the scientific community began to organize and define itself against the older natural philosophic tradition. Not only did scientists of the second half of the seventeenth century develop communication networks and produce works collectively sanctioned but they also sought out a rationale for a separate existence which offered them a differential role in
the world of intellectuals. In effect, scientists like pascal and Malebranche acknowledged the existence of a sphere of activity in which scientists had little to contribute, namely, theology, but proclaimed the special ability of colleagues to study nature directly and extract from it ever more precise regularities.
Those familiar with the English tradition in natural religion, in which Sir Isaac Newton participated, will notice what a radical turn this Continental path represents, a point that once more underscores my admonition to stray from the straight and narrow path mapped out by traditional historians of the Scientific Revolution.
Yet in another important sense, the followers of this Continental path shared with their colleagues across the Channel a distruct of essential qualities. At about the same time, but on different occasions, d'Alembert and Buffon, who were each accused of anti-Christian leanings, were annoyed by the continued fascination their contemporaries displayed for claiming to apprehend nature's true being. Buffon argued for an empirical system of natural classification to describe the realm of natural history; d'Alembert argued for a redefinition of the elusive concept of what we now call energy conservation, to avoid having to ground his physics on an essentialist understanding of gravitational forces. Both of them justified their stand by narrowing the territory in which scientists with their current methods could reasonably expect to make headway. Although religious authorities failed to interpret it this way, nominalist scientists retreated from the impenetrable domain of final causes, cheerfully turning it over to the ecclesiastic community, while asserting their authority to speak about the empirically based laws of nature with self-assurance. That position is still fashionable today and continues to constitute a major argument in defense of science's autonomous right to know regardless of its consequences in other domains of culture.
Laplace's major contribution to this component of the Mechanistic Age was to demonstrate with heavy scientific artillery in probability theory, celestial mechanics, and physics that such a specialized, professional approach, far from diminishing the extent and richness of the scientist's domain, helped him focus on what it was reasonable for man to understand and to dream constructively about what might be possible. He did this on several occasions.
In a classic paper on heat written with Lavoisier in 1783, he argued that the ultimate truth of competing theories about its nature (kinetic vs. materialist) was best set aside in favor of the establishment, with careful experiments, of the behavior of thermal phenomena.[8] To that end, Laplace invented the ice calorimeter to measure heat exchanges in combustion and respiration and derived tables pointing to laws for the expansion of metals on heating. For us, it matters little that his efforts were
easily bested by the next generation of physicists. What is noteworthy, considering his great authority in the scientific community, was his explicit disregard for quiddity as long as some progress could be achieved by empirical means. Laplace laid great store in what we call an operational or instrumentalist view of science, one that favors the knowlable mechanisms of nature, studied mathematically, over its deeper and less evident aspects. He preferred the palpable to the speculative. In discussing respiration, for example, Laplace and Lavoisier confined themselves to statements about the oxygen consumed and the heat produced, leaving aside all reflections on the relationship between the nature of life and vital heat, a commonplace subject in their epoch.[9]
We legitimately ask if this conscious choice was merely a stratagem to disarm nettlesome religious or philosophic critics or a conviction about the speical nature of the scientists' occupation.[10] Undoubtedly both. Beyond this, and for personal reasons, Laplace also preferred epistemological certainty to ontological profundity. In one of those paradoxical events that befalls so many in their lieftime, his conventional career choice of the priesthood had been shattered by a conversion experience brought about by the discovery of the beauty and power of the analytic versions of Newtonian astronomy practiced in France. Laplace, moreover, fashioned a new mathematical tool, the a posteriori calculus of probabilities (statistical inference) that he assumed would lead with ineluctable power from observed phenomena to laws of nature and from laws to causes. The most remarkable by-product of his efforts was his massive fivevolume Traité de Mécanique Céleste , which offered a thorough analysis of the Newtonian astronomical world, using the new language of calculus but entirely stripped bare of the theological and metaphysical concerns Newton prized so dearly.
Like Newton, Laplace shunned unfounded hypotheses. A particularly clear instance of his reluctance to accept speculative theory when no direct evidence was available was his reaction to a clever but bizarre hypothesis proposed by the Genevan Le Sage to explain universal gravitation.[11] This completely mechanistic theory involved numerous tiny ultramundance particles traveling at great speed through space in a random fashion. In this model, attraction was the result of the mutual shielding efect solid bodies would have when in proximity to each other, an effect that would increase greatly as the distance between them decreased. Laplace demanded to know what speeds and masses the hypothesis entialed. When they turned out to be larger than the speed of light, he lost interest. Le Sage's particles were a clever but vacuous jypothesis that could not be tested directly with apparatus at hand and hence were not worthy of the concern of a practicing mechanistic scientist. The Mechanistic Age demanded tanglible evidence rather than un-
supported models, even when these truned out to be based on the principles of matter in motion.
It must not be assumed from this that such a positivist stance, which favored fact over fancy, ruled out hypotheses, or altogether blocked their use. It merely distinguished between pure conjecture and imaginative solutions that were testable. Laplace's postulation of the nebular hypothesis to account for the origins of the solar system is an apt illustration of this. Using probability theory, he argued that there must be a physical cause responsible for the planet's orbiting around the sun in a place close to the ecliptic, all in the same direction and with nearly circular paths. His hypothesis, though thoroughly speculative, was nonetheless testable and fruitful, hence acceptable until it was refuted. Clearly, the philosophy of what we might call phenomenological positivism did not constitute an obstacle to the scientist's imagination. On the contrary, none is more visionary than Laplace's mechanistic assertion of determinism:
The present state of the system of nature is evidently a result of what it was in the preceding instant, and if we conceive of an Intelligence who, for a given moment, embraces all the relations of being in this Universe, It will able be able to determine for any instant of the past and future their respective positions, motions, and generally their affections.
Physical astronomy, that subject of all our understanding most worthy of the human mind, offers us an idea, albeit imperfect, of what such an Intelligence would be. The simplicity of the laws by which celestial bodies move, the relationship of their masses and their distances allow us to follow their motions through analysis, up to a certain point: and in order to determine the state of the system of these large bodies in past or future centuries, it is enough for the mathematician that observation provide him with their position and speeds at any given instant.
Man owes this advantage to the power of the instrument he uses and to the small number of relations he employs in his calculations; but ignorance of the diverse causes that produce the events and their complexity, taken together with the imperfection of analysis, prevent him from making assertions with the same certitude on most phenomena.[12]
It is clear from this remarkable credo that Laplace expected future progress in science to depend on a patient but correct adaptation of the methods of astronomy to the unsolved problems of nature in other realms. On different occasions, he expressed this vision specificially for crystallography, chemistry, and psychology. In an unpublished manuscript, he repeated this conviction for biology. But we would be mistaken if we assumed his philosophy of astronomy depended on the adoption of specific axioms about matter—for example, that it be inert rather than self-activating—any more than if we assumed he refused to consider alternate theories of heat and light to the corpuscular ones favored in his day.
For him, as for many of the biological reductionists of the nineteenth century, it was the proper scientific approach to problem solving that counted more than the specific character of the theory employed. The epistemological virtue of the "mechanistic" approach mattered more than its materialistic ontology.
The physiologist Magendie, who was Laplace's desciple, and his famous student Claude Bernard accepted the notion that living beings could not transgress the laws of the inorganic world but maintained they were additionally held to laws of the organic realm.[13] Du Bois-Reymond, who belonged to the group that signed a reductionist manifesto in 1846, also adhered to this notion and recommended that only physical and chemical tools be employed to analyze physiological phenomena. He did so principally because their operations were intelligible, not because physics and chemistry were ontologically more reliable or prior.
It seems to me a major historical misunderstanding to argue that the philosophy of biological reductionism turned on a temperamental philosophic preference for the physical over the life sciences. The record, as it is now being unfolded by historians of the nineteenth century, shows that it was the example provided by the physical sciences more than their principles that impressed researchers in biology.[14] Most of the great figures received training and developed their ideal picture of what a solid science might be from experience with astronomy, physics, and chemistry. In one way or another, they all took to heart messages like the one offered by Laplace that, with sufficient persistence, the life sciences would soon arrive at a stage of maturity that gave them the kind of power of penetration reached by followers of Newtonian astronomy. We now know that their optimism was perhaps a bit premature. In their enthusiasm, they may have failed to count on the almost limitless variety that exists in the natural world and the complexity of living processes. Yet the faith in progress through the adoption of experimental science is still the driving force behind today's research programs. In that sense, the effects of the Mechanistic Age are still with us.
Ultimately, the critical by-product of the Mechanistic Age was to have provided examples of how nature could be apprehended by men of science. to be acceptable, as we have seen, any new science had to reveal the mechanism of its theories to the eye, to be grounded on verifiable evidence, to adopt an unambiguous vocabulary for the sake of clarity, to aruge cogently (preferably employing a logically reliable language), and to concern itself with immediate rather than distant causes, in any case, causes that could be tested. In short, the Mechanistic Age offered a philosophy for scientific understanding that had proven to be effective and remarkably productive.
There are other features that our rapid historic survey may have
enabled us to have glimpsed, though they are more implied than made manifest. It is perhaps most evident in the Laplacean credo. Whereas in ancient times, man and the universe were considered first as the byproducts of a creator to be contemplated and imitated, since at least the nineteenth century, the scientifically informed mind has been prepared to substitute his mental prowess for that of God. Laplace's Intelligence could just as easily be a perfected human calculator as the Supreme Being. Thus it is that the modern scientist is potentially in as good a position to construct the universe and manipulate it according to his cumulative insights as he is to analyze a universe created by a deity. In the modern era, understanding has become a means of control. Man is now the mechanic, the maker of the machine: What Vico implied by asserting the identity of verum and factum , what man makes, he is able to know.[15]
The Laplacean credo of determinism not only offered the potential of prediction, and hence a measure of control, but it also promoted man to the rank of a lesser god. That message was well understood by many of the biological reductionists who were uneasy with its implications. Du Bois-Reymond was the most explicit spokesman who raised doubts about the ultimate power of the scientific mind. In a series of popular addresses on science over a century ago, he explicitly set limits to the power of science, maintaining that despite the likelihood further research would dissolve some of our ignorance, there were issued that would never be settled by science.[16] He listed seven impenetrable riddles of nature that would forever remain byeond our ken. What is significant about them is that they included issues dear to physical scientists as well as his bilogical colleagues. They are not canonical enigmas, but his list nonetheless illustrates the kinds of metascientific issues he considered beyond the limits of the wisdom of scientists: the essence of force and matter; the origin of movement; the origin of life; the purposeful character of nature; the nature of simple sensations; the origins of intelligence and language; and the riddle of freedom of the will.
Despite the remarkable progress of science, the nineteenth century was also the period in which doubts about the implications of the Laplacean credo emerged. It is a measure of the ambiguity with which his message was greeted that Du Bois-Reymond reminded his listeners that man was not omniscient. Perhaps he should also have reminded us that he is not omnipotent either. I have always been impressed that Goethe's Faust and Shelley's Frankenstein appear on the historic scene just as the Mechanistic Age is in its full glory. Does this not suggest that issues of this magnitude are never settled?
As stated at the outset, the modern age was nonetheless irretrievably transformed by the establishment of a philosophy of mechanism that showed how much understanding could be enlarged by adopting its
tenets. True, modern man could not expect to equal the prowess of the deity simply by the use of his new secular instrument, but he could now hope to deepen his grasp on nature with considerably more confidence.
Nine—
Metaphors for Mind, Theories of Mind:
Should the Humanities Mind?
Allen Newell
The outer frame of this essay is the computer revolution. The computer threatens to infiltrate the humanities and, indeed, all intellectual disciplines. There is much to the world besides disciplines, of course, and the computer has its effects on the larger stage of economics and war. But the disciplines provide a more than adequate frame for us. The computer—by which I mean the hardware, the software, and the algorithms, along with their underlying theory—is leading to a technical understanding of all intellectual operations. By taking intellectual operations to be the technology for processing information as represented in data structures, the computer is proceeding to transform them all. From word processors, to communication networks, to data bases, to data analysis, to theoretical calculations, to simulations, to automatic running of experiments, to preparation of scholarly papers, to scheduling appointments—all across the board, it transforms first this intellectual operation and then that one. The transformations are piecemeal, mostly commercially driven, and often attack the intellectually peripheral and the social mundance. But, as with the locusts, one can hear the munch, munch, munch across the land.
Since we did not previously understand intellectual operations all that well, the sweep of this revolution is unclear and contentious. It is possible to believe that only certain intellectual operations will be transformed and that others will remain within a separate and sheltered realm. This is one reason the computer revolution is only a threat; the reality has only partly arrived, and it is unclear that the rest will ever come. Contentiousness arises because the lack of clarity extends even to whether it is a threat. Perhaps the metaphor of the locusts is false. Perhaps the arrival should be greeted with joy and hosannas. For it will bring with it not only the capability for intellectuals to engage in more intellectual operations
per day but to d so with an immeasurably deepened understanding of what the intellectual operations themselves mean. The situation is yet more complex. One of the insidious aspects of the computer revolution is that it arrives on wings of benefice—cost/benefice, it is true, but since the disciplines value funds as much as the world at large, and also find them in short supply, the inflitration of the computer is usually welcomed in the small, even while in the large, it is discussed in symposia, papers, and books.
So much for the outer frame. The theme of this volume is the location of humanity, and its method is to work along the boundaries between humanity and other things, such as beasts and machines. This connects it with the computer revolution, although in over lapping and partial ways. For the computer as machine touches this theme at more than one place: as an exemplar of the modern technological age, as a machine that deals in the root operations of intellectuals, and as something that mimics the human mind.
Which introduces the inner frame—computers and mind. This is the aspect of the computer revolution that concerns us. Whenever the issue explicitly arises of how the computer locates our humanity, the answer invariably centers on what computers tell us about our mind. It is not clear that we are right in always moving to this focus. Other aspects of the revolution may in the long run be more important. But, as in much that is human, there is recursive self-fulfillment: we inquire about what is important, but our criteria for importance is largely how much we observe ourselves inquiring.
I accept this framing. Let us inquire once more about what the computer tells us about ourselves, as reflected in our minds. However, this inner frame is still too large. We must pick out a single thread within it. Nevertheless, at last we are ready to begin, although the framing is not quite ready to end.
Metaphors for Mind
The computer is a metaphor for the mind . That is how it so often gets said. It does not even seem strange any more. Western society has always used the technologies of the time as metaphors for the human mind. For all I know, all other civilizations do the same. In any event, it is enough that we do.
We often trace the course of technological metaphors for mind from Descartes and the hydraulic fountains of his time, through the telephone switchboard, and on up to the computer. Rising above the sequence, by the sort of modest indictive capability natural to us all, we have often been assured that the leading metaphor for mind will move on with the
next technological advance (holograms were once a favorite). Some truth resides in this, no doubt. Metaphor is a particularly disarming way of arriving at truth. It invites the listener to find within the metaphor those aspects that apply, leaving the ret as the false residual, necessary to the essence of metaphor. And since within the metaphor is always, by the linguistic turn (Toews 1987), within the listener , the invitation is to find within oneself some truth that fits. Having done that, it is difficult to argue against the truth that the metaphor reveals. Indeed, to fail to find any truth that applies seems to say more about the listener than about the metaphor; perhaps he is one of those who does not get jokes either. So there is truth in the computer as a metaphor for mind, though perhaps as much social truth as scientific truth.
This brings us to the final framing, which is personal. This pervasive use of computer as metaphor for mind has always somehow rankled. Always I experience a sense of discomfort in using it, or in hearing it, or in taking it as the right way of putting it. Maybe I do not get the joke, Maybe I do and do not like it. Maybe I like it but do not know how to do it. Maybe I think it is no metaphoring matter.
In any event, let us explore the issue. I shall expand on my William James Lecures at Harvard University (Newell 1987). Although those were a perfect place for a wider view, in fact, I focused strongly on matters internal to the science of cognition and did not address such issues as the computer metaphor.
I now believe, however, that I can answer the question of what disturbs me about the use of computer as metaphor for mind. it contrasts with a theory of mind . It distances the object, so to speak. If the computer is a metaphor for mind, it is by that same token no more about mind than a metaphor. Metaphors being clearly in the eye of the beholder, they leave the objects themselves untouched, or touched in only a literary way that does not derange the topic itself, because it is only commentary. Thus, to view the computer as a metaphor for mind is to keep the mind safe from analysis.
Science is not like that. At least science does not take itself as metaphorical. Acknowledging always the necessity of approximation and the inevitability of error, it still sees itself as attempting to describe its subject matter directly and not metaphorically. It has indeed become fashionable both to deconstruct science (Latour and Woolgar 1979) and to take all science as metaphorical—to take metaphor as a metaphor for science. This can be done, of course. As already noted, metaphor has a certain inevitability about it with respect to squeezing out some truth. A boa constrictor is not a bad metaphor for metaphor. But sometimes the bones crack. In particular, it is clearly wrong to treat science as metaphor, for the more metaphorical, the less scientific. In contrast to metaphors
for mind, to understand the mind—what it is, how it can be, why it works, how far it reaches, whence it arises, and where it is located—requires a scientific theory of mind.
The metaphor for mind that the computer makes available for our use is available to us all already. It is unclear that it can or needs to be sharpened. It is already richer than might be imagined. It indicates mind as something mechanical, atomistic, context independent, slavishly rule following, repetitive, stereotypical, jerky, single minded [sic], quantitative, unchanging, rigid, unadaptive, powerful, compulsive, exhaustive, obsessed with detail, noncaring, a spindler/mutilator, passionless, formal, logical, errorless . . . Pull the plug! I am being too compulsive.
In fact, the computer as metaphor mixes many sources and changes before our eyes. The computer as computer continues to become different things at a rapid rate. Our exposure to it, in all its forms, increases correspondingly, both directly by personal experience and indirectly through both the advertising and public media and the technical and intellectual literature. One source goes back all the way to the computer as machine, in which the computer is all gears. But we have all seen enough to realize that the computer is clearly not a rolling mill or ticktock clock. So the machine view gets overlaid with others that arise from the nature of programming and from the specialized nature of applications, such as the lean, clean, saturated color panels of computer animation or the whiz and abstract thrill and terror of interactive computer games. These different sources bear family resemblances, but they blur the metaphor and allow it to convey different things on different occasions. Good thing, in fact, for a metaphor, where the richer, the better. The computer metaphor can take care of itself very well, without any help from me. My purpose is to probe whether the way to treat what the computer can tell us about mind is as metaphor or as science.
Theories of Mind
More effort needs to be spent explicating the nature of a scientific theory of mind. I prefer to do that at a specific level, not at the level of science in general. Still, something general needs to be said, if only because so much has been written and mused about the nature of science as it might pertain to humans and their societies and cultures, or whether in fact it could pertain at all. Again, the focus here is not to straighten out the notion of science per se. Rather, I want to be sure the notion of science that I use is clear.
By a theory of mind , I mean just what I would in talking about any scientific theory. I mean it in the same sense as in the theory of plate tectonics in geology, the theory of orgain chemistry, the astronomical
theory of the planetary orbits, the theory of the atom, and on and on. These examples are themselves not quite the same, but they all contain a solid common kernel. Society, in the body of the attending scientists, attempts to organize its knowledge of some body of phenomena. Its goal is to use the knowledge for prediction, explanation, design, control, or whatever. Theory is the symbolic organization of this knowledge.
Sciences all grow to look alike in many ways—the natural and biological sciences very much so, as well as bits and parts of the human sciences. They all develop bodies of solid fact and regularities and surround them with an explicit, if somewhat conventional, apparatus of evidential support. They all develop theories, which tend to mathematical and formal symbolic form. These theories tend to be mechanistic. That is, they posit a system or collection of mechanisms, whose operation and interaction produce the regularities. The theories of all the sciences all fit, more or less well, into a single theoretical fabric that is a stitched-together coherent picture of a single universe. An article of faith for a long time, this has become increasingly evident with the amazing emergence of biology to match in power and elegance the older physical sciences—and to be one with them in a seamless scientific web.
Some aspects of the sciences reflect the nature of the world, others the nature of the enterprise itself. That scientific theories are cast in terms of underlying mechanisms seems to reflect the nature of the world. Theories could be different and sometimes are. That theories have a formal and calculational character reflects the scientific enterprise. This quality makes the knowledge that is the science derivable from the theory and not from the theorist. To use a scientific theory requires both knowledge and skill, especially the latter. Hence, not everybody can take a theory and produce its results (or even reproduce them). But the results, when produced, are the results of the theory, not of the theorist—a matter of no small import, since humans themselves have (or contain, depending on your metaphor) bodies of knowledge. Humans can predict, explain, design, and control, all without benefit of science, much less theory. Perhaps what best characterizes science methodologically is its ability to get these activities into external symbolic artifacts, available to all who are "skilled in the art."
If science stayed outside the house of man, there would be nothing to consider in contrasting metaphors and theories of mind. But, of course, a scientific psychology does exist, and it is recognizably a family member of the science kin group. The contrast requires more than just a scientific psychology, however. The computer must underlie both the metaphor of mind (which it avowedly does) and the theory of mind. If this were not the case, the contrast would still amount only to the proverbial one hand clapping.
But the cognitive revolution has occurred (Gardner 1985). It is thirty years old. It has come to dominate individual psychology. New scientific upstarts now rail against it instead of against behaviorism. And this revolution has been dominated by the computer—or more correctly, by the abstract notions of computation and information processing that have emerged as the theoretical counterpart to the technological advance. Even the phiosophers say so (Dennett 1988, Fodor 1983). The acceptance has moved to the creation of an umbrella interdiscipline called cognitive science. In some quarters, we can actually hear the clapping. There is indeed a contrast to consider.
Unified Theories of Cognition
My William James Lectures give some indication of what it might mean for there to be a theory of mind, in the sense we have been discussing.
Psychology has arrived at the possibility of unified theories of cognition—theories that gain their power by having a single system of mechanisms that operate together to produce the full range of human cognition.
I did not say they are here yet, but I argued they are within reach and that we should strive to attain them. Nor did I claim there was a single such unified theory. Indeed, in my lectures I argued that in our current state of knowledge, there would be several theories. I did claim that enough was known to attempt unified theories and that they had immense benefits for cognitive science—bringing into one theoretical structure the constraints from the great store of empirical regularities that cognitive psychology has amassed, along with what we now understand about the mechanisms of cognition.
The lectures were built around the presentation of an exemplar unified theory, embodied in a system called Soar, developed by John Laird of the University of Michigan, Paul Rosenbloom of the Information Sciences Institute at the University of Southern California, and me (Laird, Newell, and Rosenbloom 1987). Soar provides an appreciation of what is required of a unified theory, what its yield might be, and how ready the field is to develop them. Soar is only an exemplar; there are others as well (Anderson 1983).
Figure 9.1 presents the elements of the theory of human cognition embodied in Soar. So far, I have taken care to say theory embodied in Soar . As we shall see, Soar is a specific kind of system—an architecture or machine organization.[1] We usually take a theory of some domain, here a theory of the mind, as being the assertions about the nature of that domain—here assertions about how the mind is structured, how it operates, how it is situated, and so on. So Soar, as a system, cannot literally be a theory. But the theory asserts that the central structure in mind is the
1. Controller-Perception-Cognition-Motor 2. Knowledge and Goals 3. Representation, Computation, Symbols 4. An Architecture plus Content 5. Recognition Memory (about 10 ms) 6. Decision Cycles—Automatic (about 100 ms) 7. Problem Spaces and Operators (about 1 sec.) 8. Impasses and Subgoals 9. Chunking (about 10 sec.) 10. Intended Rationality (100 sec. and up) | ![]() |
Fig. 9.1
Soar a unified treory of cognition.
cognitive architecture, that humans have one nad that its nature determines the nature of mind. The theory then specifies a lot about that architecture. Soar is a system that embodies these particular specifics. Because the architecture is so central and determines so much about the mind, it is convenient to slip language a bit and identify Soar with the theory of cognition it embodies.
Figure 9.1 enumerates the main mechanisms in Soar. The top four items are shared by all comprehensive cognitive-science theories of human cognition. Soar operates as a controller of the human organism, hence it is a complete system with perception, cognition, and motor components. This already takes mind in essentially functional terms—as the system that arose to control the gross movements of a manmmal in a mammalian world. Soar is goal oriented with knowledge of the world, which it uses to attain its goal. That knowledge is represented by a symbol system, which means that computation is used to encode repre-
sentations, extract their implications for action, and decode specific desired actions. Thus, Soar is an architecture—a structure that makes possible a hardware-software distinction. Most of the knowledge in such a system is embodied in the content that the architecture makes meaningful and accessible.
The rest of the items describe Soar from the bottom up, temporally speaking. Soar comprises a large recognition memory . This is realized by an Ops5-like production system (Brownston et al. 1985). A production system consists of a set of productions, each consisting of a set of conditions and a set of actions. At each moment, the conditions of all productions are matched against the elements of a temporary working memory , and those productions that are satisfied then execute, putting new elements into working memory. Human long-term memory comprises many productions, in the millions perhaps. A cycle of production execution also occurs very rapidly, around 10 milliseconds (ms).[2] Although in artificial intelligence (AI) and cognitive science, productions are usually taken to correspond to operators (deliberately deployed actions), in Soar they correspond to an associational memory. Thus, production actions behave like a memory retrieval: they only enter new elements into working memory and cannot modify or delete what is there. Also, there is no conflict resolution (of the kind familiar from Ops5); instead, each production executes independently, just like an isolated memory access and retrieval.
The nexe level of organization, which occurs within about 100 ms, consists of the decision cycle . This comprises a sequence of retrievals from long-term memory (i.e., a sequence of production firings) which assemble from memory what is immediately accessible and relevant to the current decision context. This sequence ultimately terminates when no more knowledge is forthcoming (in practice, it quiesces quickly). Then a decision procedure makes a choice of the next step to be taken. This changes the decision context, so that the cycle can repeat to make the next decision. At the 100 ms level, cognitive life is an endless sequence of assembling the available knowledge and using it to make the next deliberate choice.
The decisions taken at the 100 ms level implement search in problem spaces , which comprise the next level of organization, at the 1 second (sec.) level. Soar organizes all its goal-oriented activity in problem spaces, from the most problematical to the most routine. It performs a task by creating a space within which the attainment of the task can be defined as reaching some state and where the moves in the space are the operations that are appropriate to performing the task. The problem then becomes which operators to apply and in what order to reach a desired state. The search in the problem space is governed by the knowledge in the recogni-
tion memory. If Soar has the appropriate knowledge and if it can be brought to bear when needed, then Soar can put one operator in front of another, so step its way directly to task attainment. If the memory contains little relevant knowledge or it cannot be accessed, then Soar must search the problem space, leading to the combinatorial explosion familiar to AI research.
Given that the problem-space organization is built into the architecture, the decisions to be made at any point are always the same—what problem space to work in; what state to use (if more than one is available); and what operator to apply to this state to get a new state, on the way to a desired state. Making these choices is the continual business of the decision cycle. Operators must actually be applied, of course; life is not all decision making. But applying operators is merely another task, which occurs by going into another problem space to accomplish the implementation. The recursion bottoms out when an operator becomes simple enough to be accomplished within a single decision cycle, by a few memory retrievals.
The decision procedure that actually makes the choice at each point is a simple, uniform process that can only use whatever knowledge has accumulated via the repeated memory searches. Some of this knowledge is in the form of preferences about what to choose—that one operator is preferred to another, that a state is acceptable, that another state is to be rejected. The decision procedure takes whatever preferences are available and extracts from them the decision. It adds no knowledge of its own.
There is no magic in the decision cycle. It can extract from the memory only what knowledge is there, and it may not even get it all. And the decision procedure cna select only from the options thereby produced and by using the preferences thereby obtained. Sometimes this is sufficient, and Soar proceeds to move through its given space. Sometimes—often, as it turns out—the knowledge is insufficient or conflicting. Then the architecture is unable to continue: it arrives at an impasse . This is like a standard computer trying to divide by zero. Except that, instead of aborting, the architecture sets up a subgoal to resolve the impasse. For example, if several operators have been proposed but there is insufficient information to select one, then a tie impasse occurs, and Soar sets up a subgoal to obtain the knowledge to resolve the tie, so it can then continue.
Impasses are the dynamo of Soar; they drive all its problem solving. Soar simply attempts to execute its top-level operators. If this can be done, Soar has attained what it wanted. Failures imply impasses. Resolving these impasses, which occurs in other problem spaces, can lead to other impasses, hence to subproblem spaces, and so on. The entire
subgoal hierarchy is generated by Soar itself, in response to its inability to attain its objectives. The different types of impasses generate the full variety of goal-driven behavior familiar in AI systems—operator implementation, operator instantiation, operator selection, precondition satisfaction, state rejection, and so on.
In addition to problem solving, Soar learns continuously from its experiences. The mechanism is called chunking . Every time Soar encounters and resolves an impasse, it creates a new production (a chuck) to capture and retain that experience. If the situation ever recurs, the chunk will fire, making available the information that was missing on the first occasion. Thus, Soar will not encounter an impasse on a second pass.
The little diagram at the right of chunking in figure 9.1 sketches how this happens. The view is looking down on working memory, with time running from left to right. Each little circle is a data element that encodes some information about the task. Starting at the left, Soar is chugging along, with productions putting in new elements and the decision procedure determining which next steps to take. At the left vertical line, an impasse occurs. The architecture adds some elements to record the impasse, hence setting a new context, and then behavior continues. Finally, Soar produces an element that resolves the impasse (the element c at the right vertical line). Behavior then continues in the original context, because operationally resolving an impasse just is behavior continuing. The chunk is built at this point, with an action corresponding to the element that resolved the impasse and with conditions corresponding to the elements prior to the impasse that led to the resolution (the elements a and b). This captures the result of the problem solving to resolve the impasse and does so in a way that permits it to be evoked again to avoid that particular impasse.
Chunking operates as an automatic mechanism that continually caches all of Soar's goal-oriented experience, without detailed interpretation or analysis. As described, it appears to be simply a practice mechanism, a way to avoid redoing the problem solving to resolve prior impasses, thus speeding up Soar's performance. However, the conditions of the productions reflect only a few of the elements in working memory at the time of the impasse. Thus, chunks abstract from the situation of occurrence and can apply in different situations, as long as the specific conditions apply. This provides a form of transfer of learning. Although far from obvious, this mechanism in fact generates a wide variety of learning (Steier et al. 1987), enough to conjecture that chunking might be the only learning mechanism Soar needs.
Chunks get built in response to solving problems (i.e., resolving impasses). Hence, they correspond to activities at about the the 1 sec. level and
above. The chunk itself, of course, is a production, which is an entity down at the memory-access level at about 10 ms.
The higher organization of cognitive activity arises from top-level operators not being implementable immediately with the information at hand. They must be implemented in subspaces with their own operators, which themselves may require further subspaces. Each descent into another layer of subspaces means that the top-level operators take longer to complete, that is, are higher level. Thus, the time scale of organized cognitive activity climbs above what can be called the region of cognitive mechanism and toward the region of intendedly rational behavior. Here, enough time is available for the system to do substantial problem solving and use more and more of its knowledge. The organization of cognition becomes increasingly dictated by the nature of the task and the knowledge available, rather than by the structure of the architecture.
This rapid-fire tour through the mechanisms of Soar serves primarily to box its compass, to see the mechanisms that are involved. It is an architecture that spans an extremely wide range of psychological functions. Some limits of the range should be noted. Perception and motor behavior currently exist in the theory only in nascent form. Perhaps as important, the impasse-driven means-ends structure that builds up in a given situation is ephemeral. Long-term stable organization of behavior could hardly be held in place by the momentary piled-up impasse subgoal hierarchy. Soar does not yet incorporate a theory of what happens as the hours grow, disparate activities punctuate one another, and sleep intervenes to let the world of cognition start afresh each morning. All these aspects must eventually be within the scope of a unified theory of cognition. Soar's failure to include them shows it to be like any scientific theory, always in a state of becoming.
Our description of Soar contains a strong emphasis on temporal level . Soar models behavior from about 10 ms on up to about 1,000 sec. (30 min.). Soar, as a theory of human cognition, is tied strongly to the world of real time. Figure 9.2 provides a useful view of the time scale of human action. The characteristic time taken by processes fractionates our world into realms of distinct character. Neural systems take times of the order of 100 microseconds (µsec) to 10 ms to produce significant effects. Cognitive systems take times of the order of 100 ms to 10 sec. to produce significant effects. Beyond that, in the minutes to hours range, is something labeled the rational band. And up above that stretch time scales that are primarily social and historical, left blank because theories of unified cognition are initially situated in the lower bands, focused on the architecture.
These banks correspond to realms of scientific law. The neural band is within the realm of physical law, as we have come to understand it in
|
Fig. 9.2.
Time scale of human action
natural science. And it is physical law on down, although with a twist as it enters the realm of the very small and quantum indeterminacy. But the cognitive band, which is the structuring into a cognitive architecture, is the realm of what can be called representational law. By appropriate computational structuring, internal happenings represent external happenings. The computations obey physical laws; they are physical systems after all. But they also obey the laws of what they represent. From the internal tokens that represent two numbers, an addition algorithm fashions another internal token for a sum of the two numbers. To discover an addition algorithm is precisely to discover a tiny physical system that, while doing its physical thing, also produces situations that obey the laws of addition (given further encoding and decoding processes).
As computations operate in the service of the system's goals, the sys-
tem itself begins to behave as a function of the environment to attain its goals. This is the realm of reason. No rigid laws hold here, because goaloriented computation is precisely a device to circumvent whatever is in the way of goal attainment. In Aristotelian terms, this is the realm of final causes, whereas the neural band is the realm of efficient causes, and there was nothing in the Aristotelian scheme that corresponded to computation, which is the apparatus for moving between the two. A key point in this is that it takes time to move away from the mechanics (the architecture0 and up into rational behavior. And, indeed, it never fully happens, so that a longer but better term would be intendedly rational band .
With the picture of figure 9.2, one can see that a unified theory of cognition is primarily a theory of the cognitive band. it provides a frame within which to consider the other great determiners of human behavior—the structures of the task environments people work in and the knowledge people have accumulated through their social worlds—but it does not determine this. Rather, it describes how these determiners can be possible and what limits their expression.
Fragments of the Theory
Let me provide a few quick illustrations of the theory. These will be like strobe-light exposures—a fragment here, a flash there. Still, I hope they can bring home two critical points. First, Soar is a theory, in the same mold as theories in the other sciences, a collection of mechanisms that combine togethet to predict and explain empirical phenomena. The predictions come from the theory, not the theorist. Second, as a unified theory of cognition, Soar has a wide scope, both in types of behavior covered and in terms of time scale. Though never as great as wishes would have it, Soar can still stand for the possibility that unified theories of cognition might be in the offing. Let us begin with immediate reactive behavior, which occurs at a time scale of about 1 sec., and work up the time scale of human action.
Stimulus-Response Compatibility
Stimulus-response compatibility is a phenomenon known to everyone, though perhaps not by that name. Anyone who has arrived at an elevator to find the Up button located physically below the Down button would recognize the phenomena. The Up button should map into the direction of travel—up on top. This human sense of should , in fact translates into longer times to hit the button and greater chances to hit the wrong button. Stimulus-response compatibility effects are everywhere. Figure 9.3 shows another example, perhaps less obvious. A person at a computer editor wants to delete some word. The editor uses abbrevations, in this case dropping the vowels to get dlt . Thus, the
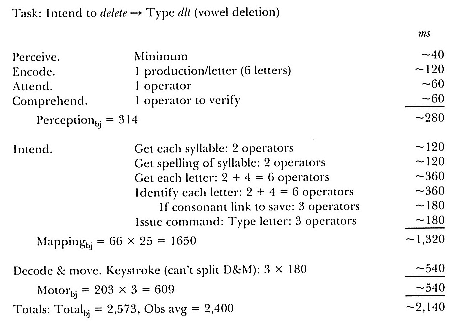
Fig. 9.3.
SRC example: Recall command abbreviation
person needs to get from delete to dlt to command the editor appropriately. Stimulus-response compatibility occurs here. On the more compatible side, the designer of the editor might have chosen delete itself, although it would have required more typing. On the less compatible side, the designer might have chosen gro , thinking of get rid of .
Figure 9.3. shows an accountinf of how Soar would predict the time it takes a person to type dlt . First is the processing that acquires the word and obtains its internal symbol: perceive the sensory stimulus (in the experimental situation, the word was presented on a computer display); encode it (automatically) to obtain its internal symbol; attend to the new input; and comprehend it to be the task word. Second is the cognitive processing that develops the intended answer: getting each syllable; extracting each letter; determining if it is consonant; and, if so, creating the command to the motor system that constitutes the internal intention. Third is the motor processing: decode the command, and move the finger to hit the key (successively d, l , and t ). The entire response is predicted to take about 2.1 sec. (2,140 ms), whereas it actually took 2.4 sec.
Soar is operating here as a detailed chronometric model of what the human does in responding immediately in a speeded situation. This does not fit the usufl view of an AI-like system, which is usually focused on higher-level activities. But a theory of cognition must cover the full tem-
poral range of human activity. In particular, if the theory of the architecture is right, then it must apply at this level of immediate behavior.
These operators and productions are occurring within the architectural frame indicated in figure 9.1. But Soar is not an origional theory here. Lots of psychological research has been done on such immediate-response tasks, both theoretical and experimental. It has been a hallmark of modern cognitive psychology. In this case, the experimental work goes back many years (Fitts and Seeger 1953), and there is an extant theory, developed primarily by Bonnie John (1987), which makes predictions of stimulus-response compatibility. What is being demonstrated is that Soar incorporates the essential characteristics of this theory to produce roughly the same results (the numbers subscripted with bj are the predictions from John's theory).
Acquiring a task
Figure 9.4 shows a sequence of situations. At the top is a variant of a well-known experiment in psycholinguistics from the early 1970s (Clark and Chase 1972). In the top panel, a person faces a display, a warning light turns on, then a sentence appears in the lefthand panel and a picture of a vertical pair of symbols in the right-hand panel. The person is to read the sentence, then examine the picture and say whether the sentence is true or not. This is another immediate-response chronometric experiment, not too different in some ways from the stimulus-response compatibility experiment above. In this case, one can reliably predict how long it takes to do this task, depending on whether the sentence is in affirmative or negative mode, uses above or below , and is actually true or false. This experiment, along with many others, has shed light on how humans comprehend language (Clark and Clark 1977).
Our interest in this example does not rest with the experiment itself but with the next panel down in the figure. This is a set of trial-specific instructions for doing the task. A cognitive theory should not only predict the performance in the experiment but also how the person reads the instructions and becomes organized to do the task. The second panel gives the procedure for doing the task. Actually, there were two variants of the experiment, the one show, and one where 4 reads "Examine the picture" and 5 reads "Then read the sentence." These are not the only instructions needed for doing the task. The bottom who panels indicate increasingly wider contexts within which a person does this task. These panels, written in simple language, are an overly homogeneous and systematic way of indicating these layers of context. In an actual experiment, the person would gather part of this information by observation, part by the gestures and behavior of the experimenter, and part by interaction directly with the experimental apparatus.
The experiment occurs 1. Light turns on. 2. Display shows. | ![]() |
3. Subject roads, exzmines, and press a button. Prior trial-specific instructions 4. "Read the sentnece." 5. "Then examine the picture." 6. {Press the T-button if the sentence is true of the picture." 7. "Push the F-button if the sentnece is false of the picture." 8. "Then the task is done." Prior general instructions 9. "at some moment the light will come on." 10. "After the light comes on, a display will occur." 11. 'The left side of the display shows a sentence." 12. "The right side of the display shows a picture." Introduction 13. "Hello." 14. "This morning we will run an experiment." 15. "Here is the experimental apparatus." 16. . . . |
Fig. 9.4.
Acquiring a task
Soar does both the top two panels (but not the buttom two). Focusing on the second panel, as the interesting one for our purposes, Soar takes in each simple sentence and comprehends it. This comprehension results in a data structure in the working memory. Soar then remembers these specifications for how to behave by chunking them away, that is, by performing a task whose objective is to be able to recall this information, in the context of being asked to perform the actual task. On recalling the instructions at performance time, Soar performs the task initially by following the recalled instructions interpretively, essentially by following them as rules. Doing this leads to building additional chunks (since Soar builds chunks to capture all its experiences). On subsequent occasions, these chunks fire and perform the taks without reference to the explicitly expressed rule. Soar has now internalized this task and performs it directly thereafter.
The point is that Soar combines performance and task acquisition in a single theory, as required of a unified theory of cognition. It shows one advantage of having unified theories. The theory of the performance
task is not simply stipulated by the theorist (as Clark and Chase had to do) but flows, in part, from the theory of how the task instructions organize the person to do that performance.
Problem Solving
Let us move up the time scale. Figure 9.5 shows a little arithmetical puzzle called cryptarithmetic. The words DONALD, GERALD, and ROBERT represent three 6-digit numbers. Each letter is to be replaced by a distinct digit (e.g., D and T must each be a digit, say D = 5 and T = 0, but they cannot be the same digit). This replacement must lead to a correct sum, that is, DONALD + GERALD = ROBERT. The figure shows the behavior of a subject solving the puzzle (Newell and Simon 1972). Humans canb e given cryptarithmetic tasks and protocols obtained from transcripts of their verbalizations while they work. The subject proceeds by searching in a problem space; the figure shows the search explicitly, starting in the initial state (the upper left dot). Each short horizontal segment is an operator application, yielding a new state. When the search line ends at the right of a horizontal line, the subject has stopped searching deeper and returns to some prior state already generated (as inicated by the vertical line, so that all vertically connected dots represent the same state on successive returns). The subject often reapplies an earlier operator, as indicated by the double lines, so the same path is retrod repeatedly.
It takes the subject about 2,000 sec. (30 min.) to traverse the 238 states of this search, averaging some 7 sec. per state. Although a puzzle, it is still genuinely free cognitive behavior, constrained only by the demands of the task. This particular data is from 1960, being part of the analysis of problem solving by Herb Simon and me (Newell and Simon 1972). A unified theory of cognition should explain such cognitive behavior, and Soar has been organized to do so, providing detailed simulations of two stretches, lines 1–4 and 8–12. Figure 9.6 shows the more complex behavior fragment (lines 8–12), where the subject has trouble with column 5 of the sum (E + O = O) and thus goes over the material several times, a behavior pattern called progressive deepening . These two stretches are far from the whole protocol, but they still amount to some 200 sec. worth.
The reason for reaching back to old data is the same as with the stimulus-response compatibility and the sentence-comprehension cases. Initially, the most important element in a proposed unified theory of cognition is coverage—taht it can explain what existing theories can do. One attempts to go further, of course. In the sentence case, it is getting the theory to cover the acquisition of the task by instruction. In the cryptarithmetic case, it is attaining completness and detail.
Development
Finally, consider an attempt to understand how the development of cognitive functions might occur. This territory has been
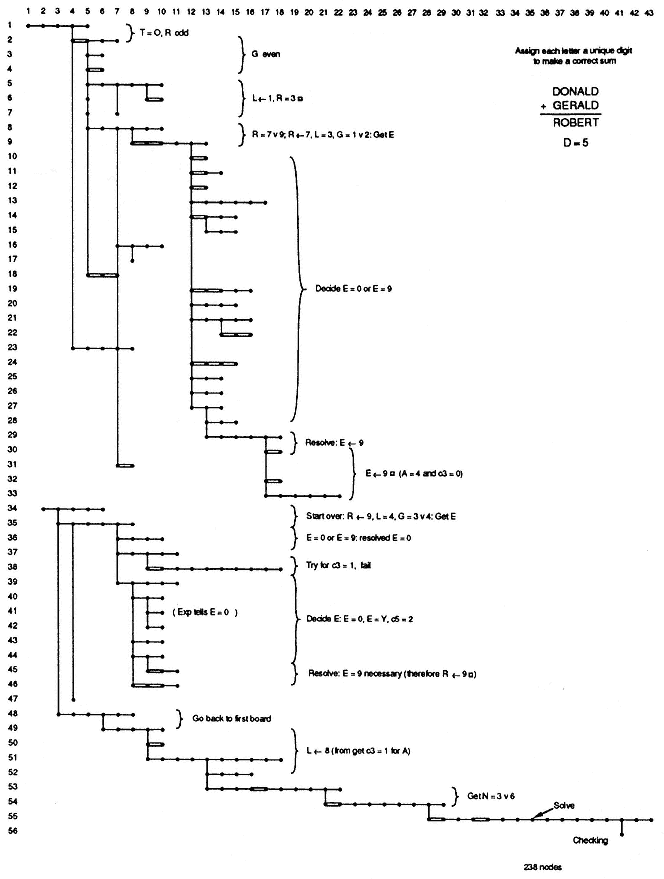
Fig. 9.5.
Behaviro of a person on the cryptarithmetic task
mapped out by Piaget, who gave us an elaborate, but imperfect and incomplete, theoretical story of stages of development, with general processes of assimilation and accommodation, oscillating through repeated equilibrations. Piaget also mapped the territory by means of a large and varied collection of tasks that seem to capture the varying capabilities of
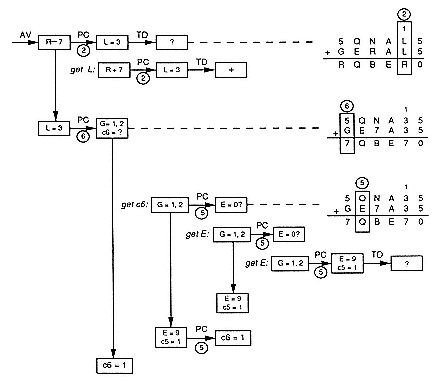
Fig. 9.6.
Soar simulation of the cryptrithmetic task
children as they grow up. Some are widely known, such as the conservation tasks, but there are many others as well.
This exploration with Soar uses the Piagetian task of predicting whether a simple balance beam (like a seesaw with weights at various distances on each side) will balance, titl right, or tilt left with various placements of weights. As they grow up, children show striking differences in their ability to predict, only taking total weight into account (around 5 years), to considering both weights and distance, providing they are separable, to (sometimes) effectively computing the torque (by late adolescence). Developmental psychologists have good information-processing models of each of these stages (Siegler 1976), models that are consonant with cognitive architectures such as Soar. What is still missing—here and throughout developmental psychology—is what the transition mechanisms could be (Sternberg 1984). That, of course, is the crux of the developmental process. It will finally settle, for instance, whether there really are stages or whether cognitive growth is effectively continuous.
Soar provides a possible transition mechanism. It learns to move
through the first two transitions: from level 1 (just weights) to level 2 (weights and distance if the weights are the same) to level 3 (weights, and distance if they do not conflict). It does not learn the final transition to level 4 (computing torques).[3] Soar predicts how the vbeam will tilt by encoding the balance beam into a description, then using that description to compare the two sides, and finally linking these comparisons to the three possible movements (balance, tilt-left, tilt-right). Soar has to learn both new encodings and new comparings to accomplish the transitions, and it does both through chunking. Figure 9.7 provides a high-level view of the transition from level 1 to level 2. It shows the diffrent problem spaces involved and only indicates schematically the behavior within problem spaces. My purpose, however, is not to show these learnings in detail. In fact, both types of learning are substantially less rich than needed to account for the sorts of explorations and tribulations that children go through.
The above provides the context for noting a critical aspect of this effort to explore development with Soar. Soar must learn new knowledge and skill in the face of existing learned knowledge and skill, which is now wrong. In this developmental sequence, the child has stable ways of predicting the balance beam; they are just wrong. Development implies replacing these wrong ways with correct ways (and doing so repeatedly). That seems obvious enough, except that Soar does not forget its old ways. Chunking is a process that adds recognitional capability, not one that deletes or modifies existing capability. Furthermore, the essence of the decision cycle is to remain open to whatever memory cn provide. Soar, as a theory of human cognition, predicts that humans face this problem, too, and there is good reason and some evidence on this score. Humans do not simply forget and destroy their past, even when proved wrong.
The solution within Soar is to create cascades of problem spaces. If an existing problem space becomes contaminated with bad learning, a new clean space is created to be used in its stead. That is, whenever the old space is to be used, the new one is chosen instead. Of course, when first created, this new space is empty. Any attempt to use it leads to impasses. These impasses are resolved by going back into the old space, which is still around, since nothing ever gets destroyed. This old space contains the knowledge necessary to resolve the impasse. Of course, it also has in it the bad learning. But this aspect can be rejected, even though it cannot be made to go away. The knowledge for this must come from a higher context, which ultimately derives from experimental feedback. Once an impasse has been resolved by appropriate problem solving in the old space, chunks are automatically formed (as always). These chunks transfer this knowledge into the new space. Thus, on subsequent occurrences
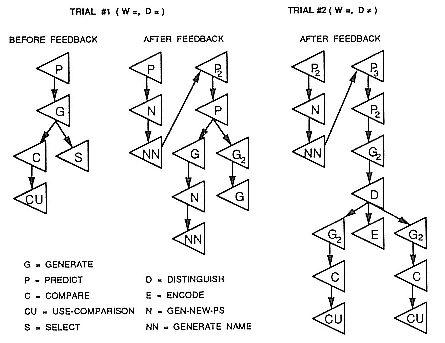
Fig. 9.7.
Problem spaces used in learning about the balance beam
of using the new space, it will not have to return to the old space. It may do so for some other aspect, but then that too is transferred into the new space. Gradually, with continued experience, the new space is built up and the old space entered less and less often. But it always remains, because Soar never knows all the information that was encoded in the old space, nor could it evaluate its quality in the abstract. Only in the context of an appropriate task does such knowledge emerge.
The Scope of Soar
Soar addresses a significant range of other phenomena that surround central cognition. Figure 9.8 provides a summary list. Heading the list is the demonstration that Soar accounts for the ability to be intelligent. One of the reasons AI is closely related to cognitive psychology is that functionality is so important. A theory of cognition must explain how humans can be intelligent. But there seems no way to demonstrate this without constructing something that exhibits intelligent behavior according to the theory. Soar demonstrates this by being a state-of-the-art AI system (Laird, Newell, and Rosenbloom 1987).
Soar exhibits the qualitative shape of human cognition in many global
1. The ability to exhibit intelligence 2. Global properties of cognitive behavior 3. Immediate-response behavior 4. Simple discrete motor-perceptual skills 5. Acquisition of cognitive skills 6. Recognition and recall of verbal material 7. Short-term memory 8. Logical reasoning 9. Problem solving 10. Instructions and self-organization for tasks 11. Natural language comprehension 12. Developmental transitions |
Fig. 9.8.
Cognitive aspects addressed by Soar
ways. For instance, it is serial in the midst of parallel activity and it is interrupt driven. Next, Soar provides a theory of immediate responses, those that take only about 1 sec. Stimuls-response compatibility was an example. Soar also provides a theory fo simple discrete motor-perceptual skills, namely, transcription typing. In general, however, Soar is still defincient in its coverage of perceptual and motor behavior, with typing as close as we have gotten to these critical aspects.
Soar provides a plausible theory of acquisition of cognitive skills through practice. It also offers the main elements of a theory of recognition and recall of verbal material—the classical learning domain of stimuls/response psychology. Soar also provides some aspects of a theory of short-term memory, including a notion on how the plethora of different short-term memories (whose existence has been revealed experimentally) might arise. It provides a theory of logical reasoning, of problem solving, and of how instructions are converted into the self-organization for doing new immediate-response tasks. In addition, it implies a specific but still undeveloped theory of natural language comprehension. Finally, it has demonstrated an idea for a transiton mechanism in developmental psychology.
Figure 9.8 presents the range of things that one candidate for a unified theory of cognition has addressed. it has done this with varying degrees of success, depth, and coverage. No claim is made for its superiority over existing cognitive theories. Indeed, Soar contains mechanisms that make it a variant of existing successful theories. The issue is not to demonstrate nvoelty but to show that a single unified theory can cover all these phenomena—that it is one architecture that does all of these tasks and does them in fair accord with human behavior on the same tasks. Finally, to come back to our main point, Soar is a genuine theory. It is not just a broad framework or a simulation language that provides a medium
to express specific microtheories. One does calculations and simulations with Soar and reasons from the structure of the architecture to behavior. Above all, Soar is not a metaphor for mind.
Should the Humanities Mind?
We have taken a brief tour of Soar. Should the humanities care? They could be fascinated, of course, or repelled, or bored, or mildly pleased at being informed about events across the intellectual river. But should they care ?
Recall the contrast: metaphors for mind or theories of mind. The computer provides a metaphor for mind, to be used at will, for all that metaphor is good for. Soar represents the other side of the contrast. It is also the computer—a living representative of artificial intelligence and computer science, a state-of-the-art AI system realized as a computer program, employing many mechanisms historically central to AI. But Soar is also a theory of human cognition, a theory of mind. It is put forth not as metaphor but as a standard sort of scientific theory, analogous to, say, the valence theory of chemical compounds or the kinetic theory of gases in thermodynamics. Soar embodies much that has gone into the computer-derived theories of mind, which have played a central role in the cognitive sciences. Soar is not pure AI, whatever that might be, but the melding of cognitive psychology and AI.
What follows for the humanities if it turns out that the metaphorical stance cannot prevail and that, with greater or lesser speed, a theory of mind emerges? Is the mind likely to clank, if the computational view is sustained? Or will it make no difference, because it will simply become a new psychophysics that occupies some obscure scientific corner, while the great questions march on always as before? Or will it turn the humanities into a part of science? If such a transformation were to occur, would it be a good thing for the humanities? Would it finally give them power to deal with questions they have found it difficult to grapple with? Would they pose new questions? Would they start to accumulate knowledge as opposed to history? Or would it destroy them, by grinding them down into laboratory drudges focused only on small questions? Even if it could be maintained that the true, good, and beautiful still lay over the far horizon, would it raise so much havoc in the transition to not be worth the candle? And if it is not worth the candle, can it then be avoided? Or must it be suffered like the plague?
Such questions cannot be answered any more than history can be lived in advance. One way to approach them is by speculating from Soar as a unified theory of cognition. Suppose this is how a cognitive theory will look. Suppose, further, this theory is as right about humans as, say, the
classical valence theory is about chemical reactions (which is not all that right, by the way, and much better approximations exist in orbital theory). What kidns of things might follow for the humanities? What would such a science tell them about the nature of mind, hence the nature of man, that they might find useful, interesting, or provocative?
I offer several such speculations. They must be taken as such. They can be appreciated, criticized, and used for further speculation. But they are not solid scientific claims. They attempt too much. Treat them as precursors, perhaps. But none of this makes them metaphorical—approximate, irrelevant, or downright wrong, perhaps, but not metaphorical.
Speculation 1—
On being Logical and the Nature of Insight
The issue is familiar and easily stated. Are people logical? Why not? Do people follow rules? If so, do they do so on everything and always? Why in insight opposed to reason? What is insight anyway? These are not all the same question, but they all cluster in the same corner of our concern with our nature. Indeed, the computer metaphor for mind is itself part of this cluster, though here we use the computational theory of mind to explore the nature of the corner.
What does the theory say? Let us start with representation—the form in which knowledge about the world or task is encoded into internal data structures. Consider a situation with an apple, ball, and cap on a table, viewed by someone in passing (fig. 9.9). A moment later, when asked where the ball is, the person promptly answers that it is between the apple and the cap. The situation is simple, but prototypical, permitting the inference that humans have memory, containing knowledge of the external world, organized to permit the performance of tasks (in this case, answering a simple question about an observed situation).
Two broad classes of representations can be distinguished (among others). On the one hand are propositions . The statement, the apple is to the left of the ball, can serve as prototype, although it is necessary to abstract away from its specifically linguistic form to amdit various graphlike and Lisp-like symbolic structures (and P1 of the figure is abstracted a little). On the other hand are models . The list (A B) in the figure at the right side (M1) can serve as prototype. An item is to the left of another if it occurs before it in a list.
Both representations are symbolic: they refer to something external, to (in this case) apples, balls, and their spatial relation, and they are realized in symbolic structures of a familiar kind. But they represent in different ways. Each requires different processes to encode knowledge into the representationa nd make use of it. Propositions involve the familiar apparatus of connectives, variables, predicates, functions, quantifiers, modalities, and rules of inference. Their processing is easily de-
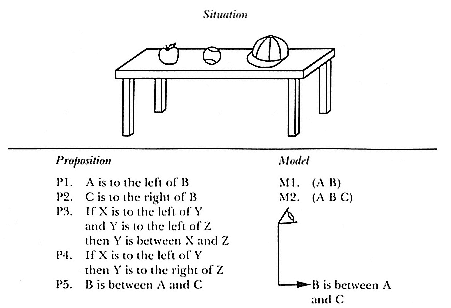
Fig. 9.9.
Propositions and models
limited and realized in many kinds of computer programs, for example, theorem provers. Models are also familiar. Their structures can be put in part-to-part correspondence with what they represent. Their processing is not quite so easily delineated, but it can be described as matchlike .
The difference is easily illustrated in figure 9.9 by what is required to answer the question of the whereabouts of the ball. On the propositional side, P1 and P2 encode the information about the table as acquired in passing. P3 and P4 are two general rules of inference, and by their appropriate application, the answer P5 is produced. on the model side, M2 encodes the information acquired in passing (being an augmentation of M1, the initial encoding), and then inspection of this representation (by the eye at the ottom) simply reads off the fact that the ball is between the apple and the cap. The reading-off process must, of course, embody the semantics of the encoding, which means it must have been formed especially for this encoding scheme (though not especially for this particular question).
Soar posits that humans always represent by models.[4] The eye of figure 9.9 is the recognition memory of figure 9.1. The construction of special recognizers for various classes of tasks is accomplished by chunking in response to initially working it through deliberately. There is a fair amount of evidence generally in the cognitive literature that humans do
use models (Johnson-Laird 1983), although the case is not conclusive. It is difficult to be conclusive about representations since alternative representations can mimic one another in many ways.
But we are interested in what our theory says. Why might humans employ models? A prime reason is functional. A model is a representation that can be processed rapidly and with assurance by recognition (matchlike) processes. Two models of something can be seen to be the same (or different) just by putting them into part-to-part correspondence and checking each part locally. Not only are models fast but they can be relied on to be fast. Propositions, however, require general inference techniques—essentially the proving of theorems and the derivation or results. These are processes that can take an indefinite amount of tiem and that lead to combinatorial explosions. For a system built to operate in real time, under the necessity of rapid response, models are substantially more satisfactory than propositions. It might also be observed that models are a natural representation for recognition-memories such as Soar's; after all, the production system is the eye that surveys the model and sees its properties. But that is not a coincidence. Model and recognition are as yin and yang.
Models satisfy another function, namely, the relation of being the internal representation of the external situation with which the organism is in close-coupled sensory contact. To track and fixate a moving visual target requires an internal representational structure. Such a structure functions as a pointer-structure to permit the coordination of eye movements, occlusion, object permanence when the object disappears behind a tree, and so on. It has intense real time constraints, for continuous updating and for coordinated action. Phenomenally, it would be transparent—pipes through which attention reaches out to contact the moving scene. Models with their part-to-part correspondences are clearly fitted for this task—built like an optic fiber, with its multiplicity of channels, to stay with the pipe metaphor. It is a small (but real) leap to take the representations for all thought as being grounded structurally in the representations that are available for perceptual-motor purposes. But it is another reason to believe that human representations are models, to wit, they grew out of the operational models used in perceiving and acting.
As might be expected, a trade-off is involved. Models are not nearly as powerful a representation as propositions. In their pure form, they cannot deal with notions of disjunction, negations, or quantification. Even if augmented by various notations, such as a tag that a given model part is not in the real situation (which thus adds a local propositional element), they remain extremely limited. However, propositional representations, especially forms that are equivalent to quantified logics, have essentially, unlimited representational power.
In terms of the theory, humans are not logical because models do not lend themselves to working with general situations. Models work well for situations such as that of figure 9.9. But human failings in reasoning are typically revealed by tasks such as syllogisms:
All bakers are golfers.
Some bakers are not cardplayers .
What follows necessarily about folfers and cardplayers?
It is difficult to represent such quantified situations with models. The expressions can represent a diversity of possible situations, which cannot be captured in any single model. To reason correctly requires generating all possible models and accepting as valid only what holds in all of them. But this requires elaborate and controlled processing, which humans find difficulties do in their heads.
That humans represent by models does not rule out their employing propositions mentally. They certainly hold internal dialogues with themselves and, no doubt, more complex reasonings as well. Within the theory, this occurs by working with models of propositions . The internal representation is a model of the propositional form, and the operators are rules of finerence. They could be rules such as P3 and P4 in the figure, or even more basic ones such as modus ponens and substitution, which apply encoded forms of rules such as P3 and P4 to other propositions such as P1 and P2. This might seem to abolish the distinction between models and propositions. On the contrary, it reveals how different they are. For the cognitive system, working with a model is working directly in terms of what the model refers to. Thus, when it working with propositions, it is working directly with propositions, all right, but therefore only directly—that is, at one remove—from what the prositions refer to. This is the basis, within the theory, for the fact that following rules is always cognitively remote, compared to directly grasping or seeing the facts themselves.
Insight is bound up with the modellike structure we have described Seeing that the ball is between the apple and the cap in M2 of figure 9.9 is as close to direct appreciation as the organism comes. No application of rules mediates the process.[5] The recognition of the memory system is inner sight, so to speak. It is not a homunculus: it does not reason, and it does not reflect (that happens in the higher levels of decision cycles and problem spaces). It responds to patterns. The patterns it sees are not fixed in advance, say, by nature. Chunking adds continually new patterns. These can be highly specific, for example, involving the relationship of betweenness, as expressed in a given modellike representation.
Suppose all the above were true? Should the humanities care? It would seem so. The central point would be to make evident exactly why
it is that humans fail to be logical or arrive at conclusions by applying rules versus seeing the matter directly. It would also make clear that this depends on specific features of the human architecture and why these features are part of the architecture. This would lead on to understanding that there could be intelligent systems with different architectural properties, which would be quite different—never showing insight, or always being logical on small reasoning tasks, on never being able to apply rules and working by insight. Such a zoo of intelligences would let us understand our own humanity, with the liberation that comparative study always brings.
Speculation 2—
On Being Embedded in the Natural Order
The issue is the place of man in the universe. The background can start with the special version of this in our Western heritage—Greek and Hebrew roots, through Rome and up through the emergence of European civilization. A strand of this heritage is the uniqueness of man and his separateness from the other creatues of the world, even his hegemony over them. Not all cultural heritages have this particular homocentric focus, but ours certainly does. The scientific contribution to this heritage, at least in the received version of cultural history, is a succession of defeats for uniquesness and special position and a gradual realization that man is part of nature, not to be distinguished in kind and maybe only modestly in degree. Some of the major scientific revolutions are associated with such defeats (or victories, if you are rooting for the other side). Think of the Copernican revolution, which decentered man and his earth, the Darwinian revolution, which joined man firmly with the animals, and the Freduian revolution, which removed man's action from the control of his own conscious will.
One current battleground over human uniqueness seems to be the human mind—its intelligence, ability to use language, and so on.[6] The computer, itself, is one of the skirmish lines. For it humans were like a computer—whatever that might mean, given the diverse and multiple notions comprising the computer metaphor for mind—then humankind would no longer be unique. Or at least its mind would not, so that some other source of uniqueness would have to be found. And the supply is becoming a little lean.
What does the theory say? There is the obvious statement that a scientific psychology makes the human mind a part of the natural order. Indeed, a scientific biology does so almost as well. However, both could still leave the central claim of uniqueness essentially untouched. Instead, let us focus on the attempt to admit computers as capable of solving difficult tasks but to take them as doing so in an entirely different way than human intelligence. Thus, it is said, the computer solves problems
by brute force. In the context of the grand battle above, such a move yields on functionality—solving difficult problems is not unique to humans—but preserves style and type. In the words of Monty Python's Flying Circus, any discussion of computer intelligence should be prefaced by, "And now for something completely different."
Figure 9.10 provides an analysis of this issues. It shows a space within which intelligent systems are located by the means they use to perform their task.[7] The vertical axis is preparation , the extent to which a system draws on what it has prepared in advance of the task. The horizontal axis is deliberation , the extent to which a system engages in processing once the task has begun. The curves represent equal-performance isobars. Different choices of how much to draw on prepared material and how much to compute once the task is set can yield the same performance—more preparation and less deliberation versus less preparation and more deliberation. Traveling out along the arrow moves from lower performance isobars to higher performance ones. Both axes represent knowledge—knowledge that comes from stored memory structures (what has been prepared) and knowledged that comes from computation during the decision process. The axes, however, are not measured in knowledge abstractly. Stored knowledge is measured in structures (number of rules or number of memory bits) and acquired knowledge is measured in situations searched or processed.
This trade-off is fundamental to information-processing systems. The structure of a system places it in a local region of this space, for it tends to treat all tasks similarly. The rough locations of various types of AI systems are shown in figure 9.10. The early AI systems were search oriented, with little knowledge (equivalent to a dozen rules) and modest search (hundreds of situations). Expert system use more knowledge (up to about 104 rules currently) but do much less search. Indeed, they can be seen as exploration into what can be gained by immediately accessible knowledge without appreciable search. Hitech, Hans Berliner's highmaster chess machine (Berliner and Ebeling 1988) is way out at the extreme of deliberation, with about 107 situations examined per external move and with only about 102 rules. Hitech would certainly qualify as one of those systems that attains its results by brute force, that is, by massive search.
It is also possible to locate humans on this curve at least approximately. Taking chess as an example, humans can search only about 102 situations in deciding on a move. This rough estimate can be derived from Soar and is attested empirically. But humans have about 105 rules, as inferred from a Soar-like theory (Simon and Gilmartin 1973) to explain the empirical results of the well-known chess perceptions experiments (Chase and Simon 1973). Chess is only one area of expertise, of
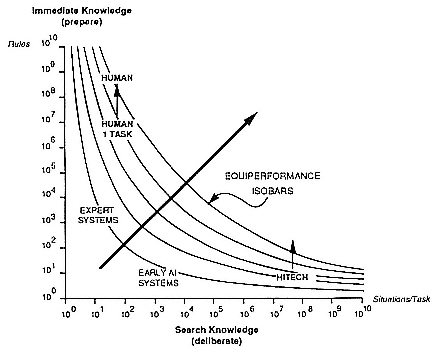
Fig. 9.10.
The preparation vs. deliration trade-off
course. The total knowledge that a human has available over all tasks would amount to many times this, although perhaps not by more than two orders of magnitude.
This space provides a fundamental view of how information-processing systems can differ and yet be related to one another. It tells us that systems, such as Hitech, are not completely different—not an unrelated way of attaining task performance (labeled say as brute force ) but rather a different part of the total space of information-processing systems. The same considerations enter into systems such as Hitech as into other systems in the space. For instance, once the architecture of a system is fixed, whether for a human or Hitech, the amount of deliberation in a given time becomes essentially fixed. Then the system can improve only by vertical movement in the space (see the small vertical arrows in fig. 9.10). Indeed, Hitech has moved from a low expert to a high master entirely by adding recognition knowledge. For another instance, it is possible to have systems that move back and up along an isobar, that is, decreasing deliberation and increasing preparation. Soar, with its chunking, is such a system. It appears much more difficult to move in the other direction. In fact, I do not know any artifical systems
that do so in any substantial way. To understand intelligence requires understanding the nature of this entire space.
Having located humans and brute force intelligent systems in the same space, can we push farther? Why, for instance, are humans located where they are?[8] The theory we are assuming (Soar) offers a clue and echoes the prior example. Human minds are controllers of real time systems. Their form as recognition-intensive rather than search-intensive systems is functional in that respect. Furthermore, their architecture is structured to learn continuously from experience, which continuously moves the system away from deliberation toward recognition.[9]
Suppose all the above were true? Should the humanities care? This analysis denies the separateness of machinelike, brute force intelligence from human recognition-intensive intelligence, which relates only to one aspect of whether the human mind is unique and what assures its unique role in the universe. Nevertheless, this is reason enough to care, given the long multistage battle on this topic, which has occupied such a secure place in Western thought.
There is, perhaps, another small yield. Such a view emphasizes that our minds are a technology—like other technologies in being a set of mechanisms for the routine solution of a class of problems. Technologies differ with respect to the features of the neatural they exploit and to the class of tasks they solve. But all technologies have much in common. This might join with our growing appreciation of other natural biological technologies—the biochemical technologies of metabolism, the macromolecular (DNA/RNA) technologies of replication and protein manufacture, and the cellular technologies of the immune system. We might come to realize the essential role of technologies in the scheme of all organized complex systems, whether natural or artifical. This might even lead us to accept technology as an essential part of what is human and therefore an essential part of a liberal and humanistic education. That also would be reason enough for the humanities to care.
Speculation 3—
On Linking the Mundane and the Sublime
The issue is the nature of creativity, which occupies a special place in the humanities. There is confounding between the role of social worth, which introduces an unsettling relativity, an the role of individual talent, which is where we Westerners seem to want to ground creative action. However, too much of what the humanities celebrate—from great composers to great artists to great writers—is polarized by the matter of creativity. The topic remains special.
What does the theory say? There is a long history of attempts within cognitive psychology to describe creativity as just problem solving (Newell, Shaw, and Simon 1962). More specifically, the activity of the creative agent
within the total social and historical creative process is asserted to be intellectual activity as predicted by Soar-like theories of cognition. It consists of searches in problem spaces, under various degrees and amounts of knowledge, as produced by the experiences of the agent. This does not provide a full accounting of what happens in creative activity, of why certain creative events occur on certain occasions with certain individuals, and so on. Much is buried in the social and historical contexts. The theory does say that no special processes are involved, beyond those that we understand from more mundane cognitive activities.[10]
Such a claim does not follow from the theory. Creativity is a phenomenon in the world, not a defined notion within the theory. Rather, such a claim obtains support (or not), as the cognitive theory of the mind is successful (or not) in explaining empirically labeled creative activities. We need to examine attempts to describe and explain creative activities, and clearly the more creative, the better.
A useful case is the research over the years by my colleague Herb Simon to understand scientific discovery in information-processing terms. This work has recently been summarized at book length (Langley et al. 1987), emphasizing that scientific discovery is problem solving as formulated in current cognitive theories. The theory that the work draws on is not a detailed cognitive architecture, such as Soar, but a more generalized formulation that is consonant with the Soar model, to wit, symbol manipulation, goal hierarchies, problem spaces, and recognition memory. The temporal grain of scientific discovery is too long to require sharp assumptions about the details of the architecture. By the same coin, this word extends the reach of a theory of the architecture, such as Soar.
The methodology employed in this research is both interesting and important. It explains historically significant events of scientific discovery—Boyle's law or Kepler's third law. These satisfy the criteria of being highly creative events. It is not possible to experiment with these events directly; they are history. Therefore, the goal is to reproduce these discoveries by a program. The actual data that the discoverer used is presented to a discovery program, and the program should make the actual discovery. It should produce Boyle's law, for instance. The context of what the discoverer knew is set as closely as possible according to the historical evidence. The record is only partial, especially the record of immediate knowledge and skill. But, in a positive confluence, creative scientific discoveries are exactly those on which historians of science lavish special attention, so that often much can be said. This approach is quite analogous to that of the paleoarchaeologists, who endeavor to chip flints by the methods available to early man, or to Thor Hyderdahl, with his sailing of the Ra from Ecuador to the Polynesian islands. Care must be taken, both in setting the stage and in
interpreting the results. But the yield is new data of a particularly intriguing and relevant sort.
Figure 9.11 summarizes the yield of this research effort. The cases are taken from Langley et al. (1987), plus one more recent effort, Kekada (Kulkarni and Simon 1988), which reproduces the synthesis of urea. There have been several different programs, each of which addresses a different type of scientific discovery problem. The varieties of the Bacon program perform data-driven induction from quantitative data, Glauber and Stahl discover qualitative laws, and so on. Some of the programs provide alternatives or more complete versions of the same discovery. The exact number of cases depends on how to count, but essentially a score of examples has now been worked through. It is important to realize that these programs (Bacon, et.) actually discover the laws. The stipulation is the context of discovery, and the program then engages in a search in the relevant problem spaces.
This research raises many questions in addition to providing support for the general proposition that creativity is continuous with problem solving on mundane tasks. Such programs require very modest search: they discover the laws quickly. Scientific discovery, as we well know, takes long efforts. Thus, there is dissonance between theory and common understanding. There are several possibilities to be explored in explaining this. Perhaps the greats, like the rest of us, never find the time actually to do science, so when they finally get a few hours, interesting things happen. Perhaps there is lots of scientific detailed work to be done, such as setting up experiments and tabulating data, activities not accounted for by these programs. Perhaps the problem spaces they work in are really much bigger and the problem much more difficult, but that explanation contains a disturbing reversal. Perhaps, finally, the creative activities are really located elsewhere, and the actual discovery from the data of, say, Boyle's law, is just a minor bit of routine activity. Then the programs are working on the wrong aspect. However, one should not embrace this last explanation too quickly. The events dealt with by the programs have certainly been considered central by scientific philosophers and historians and by the scientists themselves—as attested by Archimedes's cry of Eureka! as he leapt from a bath that could not have lasted more than a few hours.
Suppose all the above were true? Should the humanities care? The research certainly has an impact on history of science and philosophy of science. But that is to be expected, because the research speaks to them directly with the details of its findings. If, however, the research were taken to be a conclusive demonstration about the nature of creativity, it would affect all future discourse on the topic. But the research has
|
Fig. 9.11
Scientific discoveries reproduced by programs of Langley et
al. (1987) and Kulkarni and Simon (1988)
focused only on scientific creativity. Many other activities are included under the rubric of creativity—novel and impressive performances in the fine arts, the performing arts, literature, and poetry—to list only those in the heartland of the humanities. There is much evidence about the separability of these various areas, each involving unique technologies to be manipulated and skills to do the manipulations. Thus, it is possible to treat this evidence from creative scientific discovery as fragmentary. However, it would seem to raise strong enough signals to encourage seeking analogous investigations in related areas where creativity plays a similarly important role.
Speculation 4—
On Knowing and Not Articulating
The issue is that humans know things they cannot articulate. This is often said with an overtone of amazement or disbelief. One scientist cum philosopher, Michael Polyani, even turned this observation into the central theme of his philosophic oeuvre: he called it tacit knowledge (Polyani 1958). A favorite example was riding a bicycle, where it seemed clear for purposes of philosophy (i.e., without gathering empirical data) that bicycle riders could not articulate how they rode a bicycle, nor would telling nonbicylists how to ride, that is, what physical principles to use, enable them to ride bicycles. The central significance of tacit knowledge has been widely acknowledged throughout the humanities (Grene 1966), though not always tied to Polyani.
What does the theory say? It yields a straightforward answer to why human cognition shows the phenomena of tacit knowledge. Actually, it provides two separate answers: one for extended behavior and one for immediate behavior. The extended case comes from the nature of longterm memory, which is a recognition memory, in the sense we described above. In such a memory, knowledge is accessed by means of the retrieval cues that occur in short-term working memory. Thus, knowledge is accessible only if the right cues exist. But the cues are right only if they pull out the right knowledge, and the system cannot know what the right knowledge is. That is what it is trying to find out. Thus, the system in effect must guess at the retrieval cues (although the guessing can be intelligent). This situation holds for any associative or content-addressed memory, not just the specific recognition memory system posited for Soar (fig. 9.1).
In such a memory, it is no wonder that what the system knows for some purposes is not generally available to it for another. Couple this with the fact, in Soar, that procedures are also stored this way, that is, availably by recognition when the behavior is to be produced. It follows that people in general cannot articulate the knowledge of how they behave. When they do, they only construct an articulated view on the basis of the internal and external observation of their own performance.
The immediate-behavior case arises from the structure of the decision cycle (fig. 9.1). The decision cycle is composite, consisting of a sequence or production firings that accumulate the available knowledge prior to a decision actually being made. Simple reasoning occurs within a cycle as productions trigger succeeding productions. However, the decision cycle is the shortest time scale at which deliberate action can be taken. It is not possible for a person to articulate what goes on within the decision cycle. They can report various products that end up in working memory, but there is no way they can monitor the detailed processing within the cycle whereby these products originated. Soar provides an explicit picture of the lower limits of articulation. This is not, in general, what Polyani was referring to by tacit knowledge, but both types of limitations easily merge together, if there is no theoretical reason for separating them.
Suppose all the above were true? Should the humanities care? The main effect might be just debunking—to make obvious the limits on articulation, so that it would cease to play a role in larger, more profound discussions. The consequences of knowing without articulation remain, however. It remains an important ingredient in discussing artistic and literary skills and how they relate to the thoughts that artists and writers have of their own art and craft. From this standpoint, having a theory of the mechanisms that lead to the separation of knowing from articulating would seem to be extremely worthwhile.
Speculation 5—
On Repression
Ths issue is emotion and cognition. One striking feature of modern cognitive theories is their failure to incorporate emotional behavior with the cognitive mechanisms. Soar is no exception. There have been a few efforts (Colby 1975; Mueler 1987; Oatley and Johnson-Laird 1987; Simon 1967), which mostly converge on the role of emotions in the interruption of behavior. However, they do not change the overall picture.
This situation reflects the problematic relation of emotion to reason in our society as a whole. The emotion side of the scientific literature shows the same tension. For instance, althoug some theories actually compute a trajectory of a person's affective state during an interaction, they have no cognitive or task dimension (Heise 1977). Yet, it seems almost undeniable that emotion and affect exist within, raound, among, and throughout the mechanisms of the cognitive architecture. One potential approach is to identify cognitive mechanisms that might enter into emotional behaviors in an interesting way, as preparations for understanding how cognition and emotion might fit together into a single system.
What might the theory suggest? The snapshot on developmental mechanisms in Soar provides an interesting example. Recall (fig. 9.7) that the major system problem for Soar in the balance becam task was how to relearn in the face of prior learning that is wrong. This problem has a specific character in Soar, because chunking is the permanent acquisition of productions. Soar's solution was to create new problem spaces, without the bad learning. They did not necessarily contain all the good learning, but these into the originals. By operating mementarily in the old space, with additional considerations from the now one to filter out the wrong behavior, it could operate successfully. Chunking in the old space gradually transfers filtered behaviors into the new space. In this respect at least, Soar would not have to return to the old space on subsequent occasions. This entire mechanism is built within Soar, using chunking, and does not involve any architectural modifications.
The purpose in reviewing this methanism is to note what has been produced. Problem spaces that contain underirable effects are being gradually insulated from access by the new problem spaces built in front of them. Gradually, it will become more and more difficult to access these insulated spaces. HOwever, if one some occasion, perhaps much later, a way of working with the new space occurs which has not previously been attempted, the old space will be reentered. It could be like opening the door to a long-locked room. The mind is populated by such old spaces, since new ones get overlaid with additional new ones ad infinitum, depending on the larning history of the system.
This looks like a mechanism for repression, as Freud has taught us to
understand it, namely, as central in the emotional life of the individual. The Soar methanism was not deliberately designed for repression; this is a side effect. It was designed to let new learning become effective and not get mixed up with old learning that had been rejected. It is a cognitive mechanism for cognitive purposes. When we consider what a fully comptional mind might be like, we can imagaine that the phenomenton we (as good neo-Freudians) think of as repression would involve this mechanism. This does not say that Freudian repression (more generally, affectively grounded repression) is nothing but this mechanism. We know too little about emotion within the congitive realm to guess at that. Rather, it opens the possibility that emotional repression will inhabit and use such a mechanism, perhaps rather like the hermit crab, a mechanism defined for entirely cognitive reasons, and become an integral part of the emotional apparatus.
Suppose all the above were true? Should the humanities care? I would think so. The attempt to understand the realms of behavior of major significance to the humanities—represented here by emotion and affect—will be enriched by the use of theories of cognition. These will be a source of ideas about the character of emotion and how it is manifest. In short, such a theory of mind might end up becoming a central theoretical resource for the humanities.
Conclusions
We have had five speculations to provide some indication of what might follow if there were to emerge from the coming of the computer a theory of mind. They represent a postential yield from Soar, but Soar, in turn, represents the possibility of a unified theory of cognition, and such a theory, in its turn, represents the general and continued development of cognitive science.
These speculations are fragile, but I hope they are useful. A greater concern, perahsp, is their being something of a congreries, rather than a coherence. This arises from the method we chose, namely, to move from Soar toward the humanities. It was not within my ken to pick a central issue in the humanities and build the case for what a theory of mind might say. Scattershot seemed the only way to have a chance at contact.
The point of all the speculations is to illustrate that a theory of mind has a wealth of detail that allows sustained argument and investigation about why humans are the way they are. There are mechanisms of particular shapes and kinds behind the capacities and limits of our abilities to articulate, be logical, have insight, or repress. In this web of mechanisms lies many of the clues to our humnaity—what it is like and what it can be like. The details of these speculations are not critical for
this point, though to some extent their plausibility is. But most important is that they arise from the operations of a theory, not from reflections on a metaphor.
Finally, does it make any difference to the humanities which one of these two ways of viewing the matter of mind, metaphor or theory, turns out to be sustained? The two views are not symmetric. Sustain the metaphorical view and a nonevet has happened. The computer as metaphor enriches a little our total view of ourselves, allowing us to see facets that we might not otherwise have glimpsed. But we have been enriched by metaphors before, and on the whole, they provide just a few more threads in the fabric of life, nothing more. The computer as generator of a theory of mind is another thing entirely. It is an event. Not because of the computer but because finally we would have obtained a theory of mind. For a theory of mind, in the same sense as a theory of genetics or of plate tectonics, will entrain an indefinite sequence of shocks through all our dealings with mind—which is to say, through all our dealings with ourselves. And the humanities might just be caught in the maelstrom. Or so it would seem.
References
Anderson, J. R. The Architecture of Cognition. Cambridge: Harvard University Press, 1983.
Berliner, H., and C. Ebeling. "Pattern Knowledge and Search: The SUPREM Architecture," Artificial Intelligence 38 (1988): 161-198.
Brownston, L., R. Farrell, E. Kant, and N. Martin. Programming Expert Systems in OPS5. Reading, Mass: Addison-Wesley, 1985.
Chase, W. G., and H. A. Simon. "Perception in Chess," Cognitive Psychology 4 (1973): 55-81.
Clark, H. H., and W. G. Chase. "On the Process of Comparing Sentences against Pictures," Cognitive Psychology 3 (1972): 472-517.
Clark, H., and E. Clark. The Psychology of Lauguage: An Introduction to Psycholingustics. New York: Harcourt Brace Jovanovich, 1977.
Colby, K. M. Artificial Paranoia. Elmsford, N.Y.: Pergamon, 1975.
Dennett, D. C. The Intentional Stance. Cambridge: Bradford Books/MIT Press, 1988.
Fitts, P. M., and C. M. Seeger. "S-R Compatibility: Spatical Characteristics of Stimulus and Response Codes," Journal of Emperimental Psychology 45 (1953): 199-210.
Fodor, J. A. The Modularity of Mind. Cambridge: Bradford Books/MIT Press, 1983.
Gardner, H. The Mind's New Science: A History of the Cognitive Revolution. New York: Basic Books, 1985.
Grene, M. The Knower and the Known. London: Faber and Faber, 1966.
Heise, D. R. "Social Action as the Control of Affect," Behavioral Science 22 (1977): 163-177.
John, B. E., and A. Newell. "Predicting the Time to Recall Computer Command Abbreviations," in Proceedings of CHI'87 Human Factors in Computing Systems , April 1987. New York: Association for Computing Machinery.
Johnson-Laird, P. Mental Models. Cambridge: Harvard University Press, 1983.
Kulkarni, D., and H. A. Simon. "The Processes of Scientific Discovery: The Strategy of Experimentation," Cognitive Science 12 (1988): 139-175.
Larid, J. E., A. Newell, and P. S. Rosenbloom. "Soar: An Architecture for General Intelligence," Artifical Intelligence 33 (1987): 1-64.
Langley, P., H. A. Simon, G. L. Bradshaw, and J. M. Zytknow. Scientific Discovery: Computational Explorations of the Creative Processes. Cambridge: MIT Press, 1987.
Latour, B., and S. Woolar. Laboratory Live: The Social Construction of Scientific Facts. Beverly Hills: Sage, 1979.
Mueller, E. T. "Daydreaming and Computation: A Computer Model of Everyday Creativity, Learning, and Emotions in the Human Stream of Thought." Ph.D.
dissertation, Computer Science Department, University of California, Los Angeles, 1987.
Newell, A. Unified Theories of Cognition. Cambridge: Harvard University Press (in press). (The William James Lectures, Harvard University, Spring 1987).
Newell, A., and H. A. Simon. Human Problem Solving. Englwood Cliffs, N.J.: Prentice-Hall, 1972.
Newell, A., J. C. Shaw, and H. A. Simon. "The Processes of Creative Thinging," in H. E. Gruber, G. Terrell, and J. Wertheimer, eds., Comtemporary Approaches to Creative Thinging. New York: Atherton, 1962.
Oatley, K., and P. N. Johnson-Laird. "Towards a Cognitive Theory of Emotions," Cognition and Emotion 1 (1987): 29-50.
Polayani, M. Persoanl Knowledge: Toward a Post-Critical Philosophy. Chicago: University of Chicago Press, 1958.
Siegler, R. S. "Three Aspects of Cognitive Development," Cognitive Psychology 8 (1976): 481-520.
Simon, H. A. "Motivational and Emotional Controls of Cognition," Psychological Review 74 (1967): 29-39.
Simon, H. A., and K. Gilmartin. "A Simulation of Memory for Chess Positions," Cognitive Psychology 5 (1973): 29-46.
Steier, D. E., J. E. Larid, A. Newell, P. S. Rosenbloom, R. A. Flynn, A. Golding, T. A. Polk, O. G. Shivers, A. Unruh, and G. R. Yost. "Varieties of Learning in Soar: 1987," in Proceedings of the Fourth International Workshop on Machine learing , June 1987. Los Altos, Calif: Morgan Kaufman.
Sternberg, R. J., ed. Mechanisms of Cognitive Development. New York: Freeman, 1984.
Toews J. E. "Intellectual History after the Lingusitic Turn: The Autonomy of Meanning and the Irreducibility of Experience," The American Historical Review 92 (1987): 879-907.
Ten—
Thinging Machines:
Can There Be? Are We?
Terry Winograd
Introduction
Futurologists have proclaimed the birth of a new species, Machina sapiens , that will share (perhaps usurp) our place as the intelligent sovereigns of our earthly domain. These "thinking machines" will take over our burdensome mental chores, just as their mechanical predecessors were intended to eliminate physical drugery. Eventually, they will apply their "ultraintelligence" to solving all of our problems. Any thoughts of resisting this inevitable evolution is just a form of "speciesism," born from a romanic and irrational attachment to the peculiarities of the human organism.
Critics have argued with equal fervor that "thinking machine" is an oxymoron, a contradiction in terms. Computers, with their foundations of cold logic, can never be creative or insightful or possess real judgments. No matter how competent they appear, they do not have the genuine intentionality that is at the heart of human understanding. The vain pretensions of those who seek to understand mind as computation can be dismissed as yet another demonstration of the arrogance of modern science.
Although my own understanding developed through active participation in artificial intelligence research, I have now come to recognize a larger grain of tru th in the citicisms than in the enthusiastic predictions. But the story is more complex. The issues need not (perhaps cannot) be debated as fundamental questions concerning the place of hymanity in the universe. Indeed, artificial intelligence has not achieved creativity, insight, and judgment. But its shortcomings are far more mundane: we have not yet been able to construct a machine with even a modicum of common sense or one that can converse on everyday topics in ordinary lauguage.
The source of the difficulties will not be found in the details of silicon microcircuits or of Boolean logic. The basic philosophy that has guided the research is shallow and inadequate and has not received sufficient scrutiny. It is drawn from the traditions of rationalism and logical empiricism but has taken a novel turn away from its predecessors. This new "patchwork rationalism" will be our subject of examination.
First, we will review the guiding principles of artificial intelligence and see how they are embodied in current research. Then we will look at the fruits of that research. I will argue that "artficial intelligence" as now conceived is limited to a very particular kind of intelligence: one that can usefully be likened to bureaucracy in its rigidity, obtuseness, and inability to adapt to changing circumstances. The weakness comes not from insufficient development of the technology but from the inadequacy of the basic tenets.
But, as with bureaucracy, weaknesses go hand in hand with unique strengths. Through a reinterpretation and reformulation of the techniques that have been developed, we can anticipate and design appropriate and valuable uses. In conclusion, I will briefly introduce an orientation I call "hermeneutic constructivism" and illustrate how it can lead down this alternative path of design.
The Mechanization of Rationality
in the quest for mechanical explanations of (or substitutes for) human reason, researchers in artificial intelligence are heirs to a long tradition. In his "Discourse on the Method of Properly Guiding the Reason in the search of Truth in the Sciences" (1637), Descartes initiated the quest for a systematic method of rationality. Although Descartes himself did not believe that reason could be achieved through mechanical devices, his understanding laid the groundwork for the symbol-processing machines of the modern age.
In 1651, Hobbes described reason as symbolic calculation:
When a man reasoneth, he does nothing else but conceive a sum total, from addition of parcels; or conceive a remainder. . . . The operations are not incident to numbers only, but to all manner of things that can be added together, and taken one out of another . . . the logicians teach the same in consequences of words; adding together two names to make an affirmation, and two affirmations to make a syllogism; and many syllogisms to make a demonstration.[1]
Leibniz (as described by Russell)
cherished through his life the hope of discovering a kind of generalized mathematics, which he called Characteristica Universalis , by means of which
thinking could be replaced by calculation. "If we had it," he says, "we should be able to reason in metaphysics and morals in much the same way as in geometry and analysis. If controversies were to arise, there would be no more need of disputation between two philosophers than between two accountants. For it would suffice to take their pencils in their hands, to sit down to their slates, and to say to each other . . . 'Let us calculate.'"[2]
Behind this program of mechanical reason was a faith in a rational and ultimately understandable universe. The model of "Let us calculate" is that of Euclidean geometry, in which a small set of clear and self-evident postulates provides a basis for generating the right answers (given sufficient diligence) to the most complex and vexing problems. Reasonable men could be relied on to agree on the postulates and the methods, and therefore dispute could only arise from mistaken calculation.
The empiricists turned to physical experience and experiment as the true basis of knowledge. But in rejecting the a priori status of the propositions on which reasoning was based, they did not abandon the vision of rigorous (potentially mechanizable) logical procedures. For our purposes, it will suffice to adopt a broader characterization, in which much of both rationalism and empiricism fall within a common "rationalistic tradition."[3] This label subsumes the varied (and at times hotly opposed) inheritors of Descartes's legacy—those who seek to achieve rational reason through a precise method of symbolic calculation.
The electronic computer gave new embodiment to mechanical rationality, making it possible to derive the consequences of precisely specified rules, even when huge amounts of calculation are required. The first decades of computing emphasized the applicationof numerical techniques. Researchers in operations research and decision theory addressed policy questions by developing complex mathematical models of social and political systems and calculating the results of proposed alternatives.[4] Although these techniques work well in specialized caes (such as scheduling delivery vehicles or controlling the operation in a refinery), they proved inadequate for the broader problems to which they were applied. The "mathematization" of experience required simplifications that made the computer results—accurate as they might be with respect to the models—meaningless in the world.
Although there are still attempts to quantify matters of social import (for example, in applying mathematical risk analysis to decisions about nuclear power), there is an overall disillusionment with the potential for adequately reducing human concerns to a precise set of numbers and equations.[5] The developers of artificial intelligence have rejected traditional mathematical modeling in favor of an emphasis on symbolic, rather than numerical, formalisms. Leibniz's "Let us calculate" is taken in
Hobbes's broader sense to include not just numbers but also "affirmations" and "syllogisms."
The Promise of Artificial Intelligence
Attempts to duplicate formal nonnumerical reasoning on a machien date back to the earliest computers, but the endeavor began in earnest with the artificial intelligence (AI) projects of the mid-1950s.[6] The goals were ambitious: to fully duplicate the human capacities of thought and language on a digital computer. Early claims that a complete theory of intelligence would be achieved within a few decades have long since been abandoned, but the reach has not diminished. For example, a recent book by Minsky (one of the founders of AI) offers computational models for phenomena as diverse as conflict, pain and pleasure, the self, the soul, consciousness, confusion, genius, infant emotion, foreign accents, and freedom of will.[7]
In building models of mind, there are two distinct but complementary goals. On the one hand is the quest to explain human mental processes as thoroughly and unambiguously as physics explains the functioning of ordinary mechanical devices. On the other hand is the drive to create intelligent tools—machines that apply intelligence to serve some purpose, regardless of how closely they mimic the details of human intelligence. At times, these two enterprises have gone hadn in hand; at other times, they have led down separate paths.
Researchers such as newell and Simon (two other founding fathers of artificial intelligence) have sought precise and scientifically testable theories of more modest scope than Minsky suggests. In reducing the study of mind to the formulation of rule-governed operations on symbol systems, they focus on detailed aspects of cognitive functioning, using empirical measures such as memory capacity and reaction time. They hypothesize specific "mental architectures" and compare their detailed performances with human experimental results.[8] It is difficult to measure the success of this enterprise. The tasks that have been examined (such as puzzle solving and the ability to remember abbrevations for computer commands) do not even begin to approach a representative sample of human cognitive abilities, for reasons we will examine below.
On the other side lies the goal of practical system building. In the late 1970s, the field of artificial intelligence was drastically affected by the continuing precipitous drop in computing costs. Techniques that previously demanded highly specialized and costly equipment came within the reach of commercial users. A new term, "knowledge engineering," was coined to indicate a shift to the pragmatic interests of the engineer, rather than the scientist's search for theoretical knowledge.
FACTS:
Tank #23 contains sulfuric acid.
The plaintiff was injured by a portable power saw.
RULES:
If the sulfate ion test is positive, the spill material is slufuric acid.
If the plaintiff was negligent in the use of the product, the theory of contributory negligence applies.
Fig. 10.1. Rules for an expert system (from D. Waterman, A Guide to Expert Systems , 16)
"Expert systems," as the new programs were called, incorporate "knowledge bases" made up of simple facts and "if . . . then" rules, as illustrated in figure 10.1.
These systems do not attempt to explain human intelligence in detail but are justified in terms of their practical applications, for which extravagant claims have been made.
Humans need expert systems, but the problem is they don't often believe it. . . . At least one high-performance medical diagnosis program sits unused because the physicians it was designed to assist didn't perceive that they needed such assistance; they were wrong, but that doesn't matter. . . . There's a manifest destiny in information processing, in knowledge systems, a continent we shall be spread out upon sooner or later.[9]
The high hopes and ambitious aspirations of knowledge engineering are well documented, and the claims are often taken at face value, even in serious intellectual discussions. In fact, although a few widely known systems illustrate specific potentials, the successes are still isolated pinnacles in a landscape of research prototypes, feasibility studies, and preliminary versions. It is difficult to get a clear picture of what has been accomplished and to make a realistic assessment of what is yet to come. We need to begin by examining the difficulties with the fundamental methods these programs employ.
The Foundations of Artificial Intelligence
Artificial intelligence draws its appeal from the same ideas of mechanized reasoning that attracted Descartes, leibniz, and Hobbes, but it differs from the more classical forms of rationalism in a critical way. Descartes wanted his method to stand on a bedrock of clear and self-evident truths. Logical empiricism sought truth through observation and
the refinement of formal theories that predicted experimental results. Artificial intelligence has abandoned the quest for certainty and truth. The new patchwork rationalism is built on mounds of "micro-truths" gleaned through commonsense introspection, and hoc programming, and so-called knowledge acquisition techniques for interviewing experts. The grounding on this shifting sand is pragmatic in the crude sense: "If it seems to be working, it's right."
The resulting patchwork defines logic. Minsky observes,
For generations, scientists and philosophers have tried to explain ordinary reasoning in terms of logical principles—with virtually no success. I suspect this enterprise failed because it was looking in the wrong direction: common sense works so well not because it is an approximation of logic; logic is only a small part of our great accumulation of different, useful ways to chain things together.[10]
In the days before computing, "ways to chain things together" would have remained a vague metaphor. But the computer can perform arbitrary symbol manipulations that we interpret as having logical import. It is easy to build a program to which we enter "Most birds can fly" and "Tweety is a bird" and that then produces "Twenty can fly" according to a regular (although logically questionable) rule. The artificial intelligence methodology does not demand a logically correct answer but one that works sufficiently often to be "heuristically adequate."
In a way, this approach is very attractive. Everyday human thought does not follow the rigid strictures of formal deduction. Perhaps we can devise some more flexible (and even fallible) system that operates according to mechanical principles but more accurately mirrors the mind.
But this appeal is subtly deceptive. Minsky places the blame for lack of success in explaining ordinary reasoning on the rigidity of logic and does not raise the more fundamental questions about the nature of all symbolic representations and of formal (though possibly "nonlogical") systems of rules for manipulating them. There are basic limits to what can be done with symbol amnipulation, regardless of how many "different, useful ways to chain things together" one invents. The reduction of mind to the interactive sum of decontextualized fragments is ultimately impossible and misleading. But before elaborating on the problems, let us first review some assumptions on which this work proceeds:
1) Intelligence is exhibited by "physical symbol systems."
2) These systems carry out symbol manipulations that correspond to some kind of "problem solving."
3) Intelligence is embodied as a large collection of fragments of "knowledge."
The Physical Symbol System Hypothesis
The fundamental principle is the identification of intelligence with the functioning of a rule-governed symbol-manipulating device. It has been most explicitly stated by Newell and Simon:
A physical symbol system has the necessary and sufficient means for general intelligent action. . . . By 'general intelligent action' we wish to indicate the same scope of intelligence we see in human action: that in any real situation behavior appropriate to the ends of the system and adaptive to the demands of the environment an occur, within some limits of speed and complexity.[11]
This "physical symbol system hypothesis" presupposes materialism—the claim that all of the observed properties of intelligent beings can ultimately be explained in terms of lawful physical processes. It adds the claim that these processes can be described at a level of abstraction in which all relevant aspects of a physical state can be understood as the encoding of symbol structures and that the activities can be adequately characterized as systematic application of symbol manipulation rules.
The essential link is representation —the encoding of the relevant aspects of the world. Newell lays this out explicitly:
An intelligent agent is embedded in a task environment; a task statement enters via a perceptual component and is encoded in an initial representation . Whence starts a cycle of activity in which a recognition occurs . . . of a method to use to attempt the problem. The method draws upon a memory of general world knowledge . . . . It is clear to us all what representation is in this picture. It is the data structures that hold the problem and will be processed into a form that makes the solution available. Additionally, it is the data structures that hold the world knowledge and will be processed to acquire parts of the solution or to obtain guidance in constructing it.[12] [emphasis in original]
Complete and systematic symbolic representation is crucial to the paradigm. The rules followed by the machine can deal only with the symbols, not their interpretation.
Problem Solving, Inference, and Search
Newell and Simon's physical symbol systems aspire not to an idealized rationality but to "behavior appropriate to the ends of the system and adaptive to the demands of the environment." This shift reflects the formulation that won Simon a Nobel Prize in economics. He supplanted decision theories based on optimization with a theory of "satisficing"—effectively using finite decision-making resources to come up with adequate, but not necessarily optimal, plans of action.
As artificial intellignece developed inthe 1950s and 1960s, this methodology was formalized in the techniques of "heuristic search."
The task that a symbol system is faced with, then, when it is presented with a problem and a problem space, is to use its limited processing resources to generate possible solutions, one after another, until it finds one that satisfies the problem-defining test.[13]
The "problem space" is a formal structure that can be thought of as enumerating the results of all possible sequences of actions that might be taken bythe program. In a program for playing chess, for example, the problem space is generated by the possible sequences of moves. The number of possiblities grows exponentially with the number of moves and is beyond practical reach after a small number. However, one can limit search in this space by following heuristics that operate on the basis of local cues ("If one of your pieces could be taken on the opponent's next move, try moving it . . .). There have been a number of variations on this basic theme, all of which are based on explicit representations of the problem space and the heuristics for operating within it.
Figure 10.1 illustrated some rules and facts from expert systems. These are not represented in the computer as sentences in English but as symbols intended to correspond to the natural language terms. As these examples indicate, the domains are naturally far richer and more complex than can be captured by such simple rules. A lawyer will have many questions about whether a plaintiff was "negligent," but for the program, it is a simple matter of whether a certain symbolic expression of the form "Negligent (x )" appears in the store of representations or whether there is a rule of the form "If . . . then Negligent (x )," whose conditions can be satisfied.
There has been a great deal of technical debate over the detailed form of rules, but two principles are taken for granted in essentially all of the work:
1) Each rule is true in a limited (situation-dependent), not absolute, sense.
2) The overall result derives from the synergistic combination of rules, in a pattern that need not (in fact, could not in general) be anticipated in writing them.
For example, there may be cases in which the "sulfate ion test is positive" even though the spill is not sulfuric acid. The overall architecture of the rule-manipulating system may lead to a conclusion being drawn that violates one of these rules (on the basis of other rules). The question is not whether each of the rules is true but whether the output
of the program as a whole is "appropriate." The knowledge engineers hope that by devising and tuning such rules, they can capture more than the deductive logic of the domain:
While conventional programs deal with facts, expert systems handle 'lore' . . . the rules of thumb, the hunches, the intuition and capacity for judgment that are seldom explicitly laid don but which form the basis of an expert's skill, acquired over a lifetime's experience.[14]
This ad hoc nature of the logic applies equally to the cognitive models of Newell and Simon, in which a large collection of separate "production rules" operate on a symbolic store, or "working memory." Each production rules specifies a step to be carried out on the symbols in the store, and the overall architecture determines which will be carried out in what order. The symbols do not stand for chemical spills and law but for hypothesized psychological features, such as the symbolic contents of short-term memory. Individual rules do things like moving an element to the front of the memory or erasing it. The cognitive modeler does not build an overall model of the system's performance on a task but designs the individual rules in the hope that appropriate behavior will emerge from their interaction.
Minsky makes explicit this assumption that intelligence will emerge from computational interactions among a plethora of small pieces.
I'll call "Society of Mind" this scheme in which eahc mind is made of many smaller processes. These we'll call agents. Each mental agent by itself can only do some simple thing that needs no mind or thought at all. Yet when we join these agents in societies—in certain very special ways—this leads to true intelligence.[15]
Minsky's theory is quite different from Newell's cognitive architecture. In place of finely tuned clockworks of precise production rules, we find an impressionistic pastiche of metaphors. Minsky illustrates his view in a simple "micro-world" of toy blocks, populated by agents such as BUILDER (which stacks up the blocks), ADD (which adds a single block to a stack), and the like:
For example, BUILDER 's agents require no sense of meaning to do their work; ADD merely has to turn on GET and PUT . Then GET and PUT do not need any subtle sense of what those turn-on signals "mean"—because they're wired up to do only what they're wired up to do.[16]
These agents seem like simple computer subroutines—program fragments that perform a single well-defined task. But a subsequent chapter describes an interaction between the BUILDER agent and the WRECKER agent, which are parts of a PLAY-WITH-BLOCKS agent:
Inside an actual child, the agencies responsible for BUILDING and WRECKING might indeed become versatile enough to negotiate by offering support for one another's goals. "Please, WRECKER, wait a moment more till BUILDER adds just one more block: it's worth it for a louder crash!"[17]
With a simple "might indeed become versatile," we have slipped from a technically feasible but limited notion of agents as subroutines to an impressionistic description of a society of homunculi, conversing with one another in ordinary language. This sleight of hand is at the center of the theory. It takes an almost childish leap of faith to assume that the modes of explanation that work for the details of block manipulation will be adequate for understanding conflict, consciousness, genius, and freedom of will.
One cannot dismiss this as an isolated fantasy. Minsky is one of the major figures in artificial intelligence, and he is only stating in a simplistic form a view that permeates the field. In looking at the development of computer technology, one cannot help but be struck by the successes at reducing complex and varied tasks to systematic combinations of elementary operations. Why not, then, make the jump of the mind. If we are no more than protoplasm-based physical symbol systems, the reduction must be possible, and only our current lack of knowledge prevents us from explicating it in detail, all the way from BUILDER 's clever ploy down to the logical circuitry.
Knowledge As a Commodity
All the approaches described above depend on interactions among large numbers of individual elements: rules, productions, or agents. No one of these elements can be taken as reprresenting a substantial understandable truth, but this does not matter since somehow the conglomeration will come out all right. But how can we have any confidence that it will? the proposed answer is a typical one of our modern society: "More is better!" "Knowledge is power, and more knowledge is more power."
A widely used expert systems text declares,
It wasn't until the late 1970s that AI scientists began to realize something quite important: The problem-solving power of a program comes from the knowledge it possesses, not just from the formalisms and inference schemes it employs. The conceptional breakthrough was made and can be quite simply stated. To make a program intelligent, provide it with lots of high-quality, specific knowledge about some problem areas.[18] [emphasis in original]
This statementis typical of much writing on expert systems, both in the parochial perspective than inflates a homily into a "conceptual break-
through" and in its use of slogans like "high-quality knowledge." MIchie (the death of artificial intelligence in Britain) predicts,
[Expert systems] . . . can actually help to codify and improve expert human knowledge, taking what was fragmentary, inconsistent and error-infested and turning it into knowledge that is more precise, reliable and comprehensive. This new process, with its enormous potential for the future, we call "knowledge refining."[19]
Feigenbaum proclaims,
The miracle product is knowledge, and the Japanese are planning to package and sell it the way other nations package and sell energy, food, or manufactured goods. . . . The essence of the computer revolution is that the burden of producing the future knowledge of the world will be transferred from human heads to machine artifacts.[20]
Knowledge is a kind of commodity—to be produced, refined, and packaged. The knowledge engineers are not concerned with the age-old epistemological problems of what constitutes knowledge or understanding. They are hard at work on techniques of "knowledge acquisition" and see it as just a matter of sufficient money and effort:
We have the opportunity at this moment to do a new version of Diderot's Encyclopedia , a gathering up of all knowledge—not just the academic kind, but the informal, experiential, heuristic kind—to be fused, amplified, and distributed, all at orders of magnitude difference in cost, speed, volume, and usefulness over what we have now.[21] [emphasis in original]
Lenat has embarked on this task of "encod[ing] all the world's knowledge down to some level of detail." The plan projects an initial entry of about 400 articles from a desk encyclopedia (leading to 10,000 paragraphs worth of material), followed by hiring a large number of "knowledge enterers" to add "the last 99 percent." There is little concern that foundational problems might get in the way. Lenat asserts that "AI has for many years understood enough about representation and inference to tackle this project, but no one has sat down and done it."[22]
The Fundamental Problems
The optimistic claims for artificial intelligence have far outstripped the achievements, both in the theoretical enterprise of cognitive modeling and in the practical application of expert systems.
Cognitive models seek experimental fit with measured human behavior, but the enterprise is fraught with methodological difficulty, as it straddles the wide chasm between the engineering bravado of computer science and the careful empiricism of experimental psychology. When a
computer program duplicates to some degree some carefully restricted aspect of human behavior, what have we learned? It is all too easy to write a program that would produce that particular behavior and all too hard to build one that covers a sufficiently general range to inspire confidence. As Pylyshyn (an enthusiastic participant in cognitive science) observes,
Most current computational models of cognition are vastly underconstrained and ad hoc; they are contrivances assembled to mimic arbitrary pieces of behavior, with insufficient concern for explicating the principles in virtue of which such behavior is exhibited and with little regard for a precise understanding.[23]
Newell and his colleagues' painstaking attention to detailed architecture of production systems is an attempt to better constrain the computational model, in the hope that experiments can then test detailed hypotheses. As with much of experimental psychology, a highly artificial experimental situation is required to get results that can be sensibly interpreted at all. Proponents argue that the methods and theoretical foundations that are being applied to microbehavior will eventually be extended and generalized to cover the full range of cognitive phenomena. As with Minsky, this leap from the microstructure to the whole human is one of faith.
In the case of expert systems, there is a more immediate concern. Applied AI is widely seen as a means of managing processes that have grown too complex or too rapid for unassisted humans. Major industrial and governmental organizations are mounting serious efforts to build expert systems for tasks such as air traffic control, nuclear power plant operation, and, most distressingly, the control of weapons systems. These projects are justified with claims of generality and flexibility for AI programs. They ignore or downplay the difficulties that will make the programs almost certain to fail in just those cases where their success is most critical.
It is a commonplace in the field to describe expert systems as "brittle"—able to operate only within a narrow range of situations. The problem here is not just one of insufficient engineering but is a direct consequence of the nature of rule-based systems. We will examine three manifestations of the problem: gaps of anticipation, blindness of representation, and restriction of the domain.
Gaps of Anticipation
In creating a program or knowledge base, one takes into account as many factors and connections as feasible. But in any realistically complex domain, this gives, at best, a spotty coverage. The person designing a
system for dealing with acid spills may not consider the possibility of rain leaking into the building, or of a power failure, or that a labeled bottle does not contain what it purports to. A human expert faced with a problem in such a circumstance falls back on common sense and a general background of knowledge.
The hope of patchwork rationalism is that with a sufficiently large body of rules, the thought-through spots will successfully interpolate to the wastelands in between. Having written Rule A with one circumstance in mind and Rule B with another, the two rules in combination will succeed in yet a third. This strategy is the justification for the claim that AI systems are more flexible than conventional programs. There is a grain of truth in the comparison, but it is deceptive. The program applies the rules blindly with erratic results. In many cases, the price of flexibility (the ability to operate in combinations of contingencies not considered by the programmer) is irreparable and inscrutable failure.
In attempting to overcome this brittleness, expert systems are built with many thousands of rules, trying to cover all of the relevant situations and to provide representations for all potentially relevant aspects of context. One system for medical diagnosis, called CADUCEUS (originally INTERNIST ), has 500 disease profiles, 350 disease variations, several thousand symptoms, and 6,500 rules describing relations among symptoms. After fifteen years of development, the system is still not on the market. According to one report, it gave a correct diagnosis in only 75 percent of its carefully selected test cases. Nevertheless, Myers, the medical expert who developed it, "believes that the addition of another 50 [diseases] will make the system workable and, more importantly, practical."[24]
Human experts develop their skills through observing and acting in many thousands of cases. AI researchers argue that this results in their remembering a huge repertoire of specialized "patterns" (complex symbolic rules) that allow them to discriminate situations with expert finesse and to recognize appropriate actions. But it is far from obvious whether the result of experience can be adequately formalized as a repertoire of discrete patterns.[25] To say that "all of the world's knowledge" could be explicitly articulated in any symbolic form (computational or not), we must assume the possibility of reducing all forms of tacit knowledge (skills, intuition, etc). to explicit facts and rules. Heidegger and other phenomenologists have challenged this, and many of the strongest criticisms of artificial intelligence are based on the phenomenological analysis of human understanding as a "readiness-to-hand" of action in the world, rather than as the manipulation of "present-to-hand" representations.[26]
Be that as it may, it is clear that the corresponding task in building expert systems is extremely difficult, if not theoretically impossible. The knowledge engineer attempts to provide the program with rules that
correspond to the expert's experienct. The rules are modified through analyzing examples in which the original rules break down. But the patchwork nature of the rules makes this extremely difficult. Failure in a particular case may not be attributahble to a particular rule but rather to a chance combination of rules that are in other circumstances quite useful. The breakdown may not even provide sharp criteria for knowing what to change, as with a chess program that is just failing to come up with good moves. The problem here is not simply one of scale or computational complexity. Computers are perfectly capable of operating on millions of elements. The problem is one of human understanding—the ability of a person to understand how a new situation experienced in the world is related to an existing set of representations and to possible modifications of those representations.
In trying to remove the potentially unreliable "human element," expert systems conceal it. The power plant will no longer fail because a reactor operator falls asleep but beccause a knowledge engineer did not think of putting in a rule specifying how to handle a particular failure when the emergency system is undergoing its periodic test and the backup system is uot of order. No amount of refinement and articulation can guarantee the absence of such breakdowns. The hope that a system based on patchwork rationalism will respond "appropriately" in such cases is just that—a hope, and one that can engender dangerous illusions of safety and security.
The Blindness of Representation
The second problem lies in the symbol system hypothesis itself. To characterize a situation in symbolic form, one uses a system of basic distinctions, or terms. Rules deal with the interrelations among the terms, not with their interpretations in the world.
Consider ordinary words as an analogy. Imagine that a doctor asks a nurse, "Is the patient eating?" If they are deciding whether to perform an examination, the request might be paraphrased, "is she eating at this moment?" If the patient is in the hospital for anorexia and the doctor is checking the efficacy of the treatment, it might be more like, "Has the patient eaten some minimal amount in the past day?" If the patient has recently undergone surgery, it might mean, "Has the patient taken any nutrition by mouth?" and so on. In responding, a person interprets the sentence as having relevance in the current situation and will typically respond appropriately without conscious choosing among meaning.
To build a successful symbol system, decontextualized meaning is necessary: terms must be stripped of open-ended ambiguities and shadings. A medical expert system might have a rule of the form, "IF EATING (x ) . . .," which is to be applied only if the patient is eating, along with
others of the form "IF . . . THEN EATING (x )," which determine when that condition holds. Unless everyone who writes or reads a rule interprets the primitive term "Eating" in the same way, the rules have no consistent interpretation and the results are unpredictable.
In response to this, one can try to refine the vocabulary. "Currently Dining" and "Taking Solids" could replace the more generic term, or we could add construal rules, such as, "in a context of immediate action, take 'Eating' to mean 'Currently Dining.'" Such approaches work for the cases that programmers anticipate but of course are subject to the infinite regress of trying to decontextualize context. The new terms or rules themselves depend on nterpretation that is not represented in the system.
Restriction of the Domain
A consequence of decontextualized representation is the difficulty of creating AI programs in any but the most carefully restricted domains, where almost all of the knowledge required to perform the task is special to that domain (i.e., little commonsense knowledge is required). One can find specialized tasks for which appropriate limitations can be achieved, but these do not include the majority of work in commerce, medicine, law, or the other professions demanding expertise.
Holt characterized the situation as follows:
A brilliant chess move while the room is filling with smoke because the house is burning down does not show intelligence. If the capacity for brilliant chess moves without regard to life circumstances deserves a name, I would naturally call it "artificial intelligence."[27]
The brilliance of a move is with respect to a well-defined domain: the rules of chess. But acting as an expert doctor, attorney, or engineer takes the other kind of intelligence: knowing what makes sense in a situation. The most successful artificial intelligence programs have operated in the detached puzzlelike domains of board games and technical analysis, not those demanding understanding of human lives, motivations, and social interaction. Attempts to cross into these difficult territories, such as a program said to "understand tales involving friendship and adultery,"[28] proceed by replacing the real situation with a cartoonlike caricature, governed by simplistic rules whose inadequacy is immediately obvious (even to the creators, who argue that they simply need further elaboration).
This reformulation of a domain to a narrower, more precise one can lead to systems that give correct answers to irrelevant problems. This is of concern not only when actions are based directly o nthe output of the computer system (as in one controlling weapons systems) but also when, for example, medical expert systems are used to evaluate the work of physicians.[29] Since the system is based on a reduced representation of
the situation, it systematically (if invisibly) values some aspects of care while remaining blind to others. Doctors whose salaries, promotions, or accreditation depend on the review of their actions by such a program will find their practice being subtly shaped to its mold.
The attempt to encode "the world's knowledge" inevitably leads to this kind of simplification. Every explicit representation of knowledge bears within it a background of cultural orientation that does not appear as explicit claims but is manifest in the very terms in which the "facts" are expressed and i the judgment of what constitutes a fact. An encyclopedia is not a compendium of "refined knowledge" but a statement within a tradition and a culture. By calling an electronic encyclopedia a "knowledge base," we mystify its source and its grounding in a tradition and background.
The Bureaucracy of Mind
Many observers have noted the natural affinity between computers and bureaucracy. Lee argues that "bureaucracies are the most ubiquitous form of artificial intelligence. . . . Just as scientific management found its idealization in automation and programmable production robots, one might consider an artificially intelligent knowledge-based system as the ideal bureaucrat."[30] Lee's stated goal is "improved bureaucratic software engineering," but his analogy suggeste more.
Stated simply, the techniques of artificial intelligence are to the mind what bureaucracy is to human social interaction .
In today's popular discussion, bureaucracy is seen as an evil—a pathology of large organizations and repressive governments. But in his classic work on bureaucracy, Weber argued its great advantages over earlier, less formalized systems, calling it the "unambiguous yardstick for the modernization of the state." He notes that "bureaucracy has a 'rational' character, with rules, means-ends calculus, and matter-of-factness predominating"[31] and that it succeeds in "eliminating from official business love, hatred, and all purely personal, irrational, and emotional elements which escape calculation."[32]
The decisive reason for the advance of bureaucratic organization has always been its purely technical superiority over any other form of organization. The fully developed bureaucratic apparatus compares with other organizations exactly as does the machine with the non-mechanical modes of production. Precision, speed, unambiguity, knowledge of the files, continuity, discretion, unity, strict subordination, reduction of friction and of material and personal costs—these are raised to the optimum point in the strictly bureaucratic administration.[33] [emphasis in original]
The benefits of bureaucracy follow from the reductio of judgment to the systematic application of explicitly articulated rules. Bureaucracy achieves a predictability and manageability that is missing in earlier forms of organization. There are striking similarities here with the arguments given for the benefits of expert systems and equally striking analogies with the shortcomings as pointed out, for example, by March and Simon:
The reduction in personalized relationships, the increased internalization of rules, and the decreased search for alternatives combine to make the behavior of members of the organization highly predictable; i.e., the result in an increase in the rigidity of behavior of participants [which] increases the amount of difficulty with clients of the organization and complicates the achievement of client satisfaction.[34] [emphasis in original]
Given Simon's role in artificial intelligence, it is ironic that he notes these weaknesses of human-embodied rule systems but sees the behavior of rule-based physical symbol systems as "adaptive to the demands of the environment." Indeed, systems based on symbol manipulation exhibit the rigidities of bureaucracies and are most problematic in dealing with "client satisfaction"—the mismatch between the decontextualized application of rules and the human interpretation of the symbols that appear in them. Bureaucracy is most successful in a world that is stable and repetitive, where the rules can be followed without interpretive judgments. Expert systems are best in just the same situations. Their successes have been in stable and precise technical areas, where exceptions are not the rule.
Michie's claim that expert systems can encode "the rules of thumb, the hunches, the intuition and capacity for judgment" is wrong in the same way that it is wrong to seek a full account of an organization in its formal rules and procedures. Modern sociologists have gone beyond Weber's analysis, pointing to the informal organization and tacit knowledge in individual expertise. Without it, we get rigidity and occasional but irreparable failure.
The depersonalization of knowledge in expert systems also has obvious parallels with bureaucracy. When a person views his or her job as the correct application of a set of rules (whether human invoked or computer based), there is a loss of personal responsibility or commitment. The "I just follow the rules" of the bureaucratic clerk has its direct analogue in "That's what the knowledge base says." The individual is not committed to appropriate results (as judged in some larger human context) but to faithful application of the procedures.
This forgetfulness of individual commitment is perhaps the most subtle and dangerous consequence of patchwork rationality. The person
who puts rules into a knowledge base cannot be committed to the consequences of applying them in a situation he or she cannot foresee. The person who applies them cannot be committed to their formulation or to the mechanics by which they produce an answer. The result belongs to no one. When we speak here of "commitment," we mean something more general than the kind of accountability that is argued in court. There is a deep sense in which every use of language is a reflection of commitment, as we will see below.
Alternatives
We began with the question of thinking machines—devices that mechanically reproduce human capacities of thought and language. We have seen how this question has been reformulated in the pursuit of artificial intelligence, to reflect a particular design based on patchwork rationalism. We have argued taht the current direction will be inadequate to explain or construct real intelligence.
But, one might ask, does that mean that no machine could exhibit intelligence? Is artificial intelligence inherently impossible, or is it just fiendishl difficult? To answer sensibly, we must first ask what we mean by "machine." There is a simple a priori prof that machines can be intelligent if we accept that our own brains are (in Minsky's provocative words) nothing but "meat machines." If we take "machine" to stand for any physically constituted device subject to the causal laws of nature, then the question reduces to one of materialism and is not to be resolved through computer research. If, however, we take machine to mean "physical symbol system," then there is ground for a strong skepticism. this skepticism has become visible among practitioners of artificial intellige nce as well as the critics.
Emergent Intelligence
The innovative ideas of cybernetics a few decades ago led to two contrasting research programs. One, which we have examined here, took the course of symbol processing. The other was based on modeling neural activity and led to the work on "perceptrons" a research line that was discounted for many years as fruitless and is now being rehabilitated in "connectionist" theories, based on "massively parallel distributed processing." In this work, each computing element (analogous to a neuron) operates on simple general principles, and intelligence emerges from the evolving patterns of interaction.[35]
Connectionism is one manifestation of what Turkle calls "emergent AI."[36] The fundamental intuition guiding this work is that cognitive structure in organisms emerges through learning and experience, not through
explicit representation and programming. The problems of blindness and domain limitation described above need not apply to a system that has developed through situated experience.
It is not yet clear whether we will see a turn back toward the heritage of cybernetics or simply a "massively parallel" variant of current cognitive theory and symbol-processing design. Although the new connectionism may brethe new life into cognitive modeling research, it suffers an uneasy balance between symbolic and physiological description. Its spirit harks back to the cybernetic concern with real biological systems, but the detailed models typically assume a simplistic representational base much closer to traditional artificial intelligence. Connectionism, like its parent cognitive theory, must be placed in the category of brash unproved hypotheses, which have not really begun to deal with the complexities of mind and whose current explanatory power is extremely limited.
In one of the earliest critiques of artificial intelligence. Dreyfus compared it to alchemy.[37] Seekers after the glitter of intelligence are misguided intrying to cast it from the base metal of computing.There is an amusing epilogue to this analogy: in fact, the alchemists were right. Lead can be converted into gold by a particle accelerator hurling appropriate beams at lead targets. The AI visionaries may be right in the same way, and they are likely to be wrong in the same way. There is no reason but hurbris to believe that we are any closer to understanding intelligence than the alchemists were to the secrets of nuclear physics. The ability to create a glistening simulacrum should not fool us into thinking the rest is "just a matter of encoding a sufficient part of the world's knowledge" or into a quest for the philosopher's stone of "massively parallel processing."
Hermeneutic Constructivism
Discussions of the problems and dangers of computers often leave the impression that on the whole we would be better off if we could return to the precomputer era. In a similar vein, one might decry the advent of written language, which created many new problems. For example, Weber attributes the emergence of bureaucracy to the spread of writing and literacy, which made it possible to create and maintain systems of rules. Indeed, the written word made bureacuracy possible, but that is far from a full account of its relevance to human society.
The computer is a physical embodiment of the symbolic calculations envisaged by Hobbes and Leibniz. As such, it is really not a thinking machine, but a language machine . The very notion of "symbol system" is inherently linguistic, and what we duplicate in our programs with their rules and propositions is really a form of verbal argument, not the workings of mind. It is tempting—but ultimately misleading—to project the
image of rational discourse (and its reflection in conscious introspection) onto the desisn of embodied intelligence. In taking inner discourse as a model for the activity of Minsky's tiny agents, or of productions that determine what token to process next, artificial intelligence has operated with the faith that mind is linguistic down to the microscopic level.
But the utility of the technology need not depend on this faith. The computer, like writing, is fundamentally a communication medium, one that is unique in its ability to perform complex manipulations on the linguistic objects it stores and transmits. We can reinterpret the technology of artificial intelligence in a new background, with new consequences. In doing so, we draw on an alternative philosophical grounding, which I will call "hermeneutic constructivism."
We begin with some fundamental questions about what language is and how it works. In this, we draw on work in hermeneutics (the study of interpretation) and phenomenology, as developed by Heidegger and Gadamer, along with the concepts of language action developed from the later works of Wittgenstein through the speech act philosophy of Austin, Searle, and Habermas.[38]
Two guiding principles emerge: (1) people create their world through language; and (2) language is always interpreted in a tacitly understood background .
Austin pointed out that "performative" sentences do not convey information about the world but act to change that world. "you're hired," when uttered in appropriate conditions, creates—not describes—a situation of employment. Searle applied this insight to do something. Habermas extended it further, showing how sentences we would naively consider statements of fact have force by virtue of an act of commitment by the speaker.
The essential presupposition for the success of [a language] act consists in the speaker's entering into a specific engagement, so that the hearer can rely on him. An utterance can count as a promise, assertion, request, question, or avowal, if and only if the speaker makes an offer that he is ready to make good insofar as it is accepted by the hearer. The speaker must engage himself, that is, indicate that in certain situations he will draw certain consequences for action.[39]
Descartes's descendants in the rationalistic tradition take the language of mathematics as their ideal. Terms are either primitive or can be fully defined; the grammar is unambiguous; and precise truth conditions can be established through formal techniques. But even in apparently simple and straightfoward situations, human language is metaphorical, ambiguous, and undefinable. What we can take as fundamental is the engagement—the commitment to make good what cannot be fully made precise.
This grounding is especially evident for statements of the kind that Roszak characterizes as "ideas" rather than "information."[40] "All men are created equal" cannot be judged as a true or false description of the objective world. Its force resides in the commitments it carries for further characterization and further action. But it is critical to recognize that this social grounding of language applies equally to the mundane statements of everyday life. "The patient is eating" cannot be held up to any specific set of truth conditions across situations in which it may be uttered. The speaker is not reporting an objectively delineated state of affairs but indicating the "engagement" to enter sincerely into a dialogue of articulation of the relevant background.
This unavoidable dependence of interpretation on unspoken background is the fundamental insight of the heremenutic phenomenologists, such as Gadamer. It applies not just to ordinary language but to every symbolic representation as well. We all recognize that in "reducing things to numbers," we lose the potential for interpretation in a background. But this is equally true of "reducing tem to symbol structures."
Whenever a computer program is intended to guide or take action in a human domain, it inevitably imports basic values and assumptions. The basic nature of patchwork rationalism obscures the underlying constitutive "ideas" with a mosaic of fragmentary bits of "information." The social and political agenda concealed behind these patches of decontextualized and depersonalized belief is dangerous in its invisibility.
Language Machines
Symbol structures are ultimately created by people and intepreted by people. The computer, as language machine, manipulates symbols without respect to their interpretation. To the extent that relations among the meanings can be adequately reflected in precise rules, The computational manipulations make sense. The error is in assuming that thes manipulations capture, rather than augment or reify, parts of the meaning. If an expert system prints out "Give the patient penicillin" or "fire the missiles now," room for interpretation is limited and meaning is lost. But instead, we can see the computer as a way of organizing, a searching, and manipulating texts that are created by people, in a context, and ultimately intended for human interpretation.
We are already beginning to see a movement away from the arly vision of computers replacing human experts. For example, the medical diagnostic system described above is being converted from "Internist" (a doctor specializing in internal medicine) to an "advisory system" called "QMR" (Quick Medical Reference).[41] The rules can be thought of as constituting an automated textbok, which can access and logically combine entries that are relevant to a particular case. The goal is to suggest
and justify possibilities a doctor might not otherwise have considered. The program need not respond with an evaluation or plan for action but is successful through providing relevant material for interpretation by an expert. Similarly, in areas of real-time control (like a nuclear power plant), an advisory system can monitor conditions and provide warnings, reports, and summaries for human review. In a similar vein, an interactive computer-based encyclopedia need not cover all of human knowledge or provide general purpose deduction in order to take advantage of the obvious computer capacities of speed, volume, and sophisticated inferential indexing.
Another opportunity for design is in the regularities of the structure of language use. As a simple example, a request is normally followed in coherent conversation by an acceptance, a rejection, or a request to modify the conditions. These, in turn, ar efollowed by other language acts in a logic of "conversation for action" oriented toward completion (a state in which neither party is awaiting further action by the other). The theory of such conversations has been developed as the basis for a computer program called The coordinator, which is used for facilitating and organizing computer-message conversations in an organization.[42] It emphasizes the role of commitment by the speaker in each speech act and provides the basis for timely and effective action.
Howard has studied the use of computer systems by professionals evaluating loan applications for the World Bank. He argues taht their use of computers while on field missions increases the "transpoarency" of their decision-making process, hence increasing their accountability and enhancing opportunities for meaningful negotiation. The computer serves as a medium of discourse in which different commitments and their consequences can be jointly explored.
As a result, the dialogue between them [the bankers and their clients] suddenly becomes less about the final results—"the numbers"—and more about the assumptions behind the numbers, the criteria on which decisions are themselves based. . . . [quotinga bank professional] "Instead of just saying, 'I don't believe you, my opinion is X,' we explore it. We say, 'let's see what the consequences of that are.' And, sometimes, we end up changing our assumptions."[43]
Current expert systems methodologies are not well suited to this kind of dialogue. They separate the construction of the knowledge base from the use of its "expertise." The experts (with the help of knowledge engineers) enter the knowledge in the laboratory, and the users apply it in the field to get results. But we might instead use the computer to support the discourse that creates the reality—as a tool for the cooeprative articulation of the characterizations and rules that will be applied. Rather than
seeing the computer as working with objectified refined knowledge, it can serve as a way of keeping track of how the representations emerge from interpretations: who created them in what context and where to look for clarification.
Conclusion
The question of our title demands interpretation in a context. As developed in this essay, it might be formulated more precisely, "Are we machines of the kind that researchers are building as 'thinking machines'?" In asking this kind of question, we engage in a kind of projection—understanding humanity by projecting an image of ourselves onto the machine and the iamge of the machine back onto ourselves. In the tradition of artificial intelligence, we project an image of our language activity onto the symbolic manipulations of the machine, then project that back onto the full human mind.
But these projections are like the geometric projection of a three-dimensional world onto a two-dimensional plane. We systematically eliminate dimensions, thereby both simplifying and distorting. The particular dimensions we eliminate or preserve in this exercise are not idiosyncratic accidents. They reflect a philosophy that precedes them and that they serve to amplify and extend.
In projecting language as a rule-governed manipulation of symbols, we all too easily dismiss the concerns of human meaning that make up the humanities and indeed of any socially grounded understanding of human language and action. In projecting language back as the model for thought, we lose sight of the tacit embodied understanding that undergirds our intelligence. Through a broader understanding, we can recapture our view of these lost dimensions and in the process better understand both ourselves and our machines.
References
Athanasiou, Tom. "High-tech Politics: The Case of Artificial Intelligence," Socialist Review (1987): 7-35.
Austin, J. L. How to Do Things with Words. Cambridge: Harvard University Press, 1962.
Bobrow, Daniel, ed. Special Issue on Nonmonotonic Logic, Artificial Intelligence 13:1 (January 1980).
Club of Rome, The Limits to Growth . New York: Universe books, 1972.
Davis, Philip J., and Reuben Hersh. Descartes' Dream: The World According to Mathematics . San Diego: Harcourt Brace Jovanovich, 1986.
Dreyfus, Hubert. "Alchemy and ARtificial Intelligence," Rand Corporation Paper, December 1965, 3244.
———. What Computers Can't Do: A Critique of Artificial Reason. New York: Harper and Row, 1972 (2d ed. with new preface, 1979).
———. "Making a Mind versus Modeling the Brain: Artificial Intelligence Back at a Branchpoint" Daedalus 117:1 (Winter 1988): 14-44.
Dreyfus, Hubert L., and Stuart E. Dreyfus. Mind Over Machine: The Power of Human Intuition and Expertise in the Era of the Computer. New York: Macmillan/The Free Press, 1985.
Feigenbaum, Edward A., and Pamela McCorduck. The Fifth Generation: Artificial Intellignece and Japan's Computer Challenge to the World. Reading, Mass.: Addison-Wesley, 1983.
Flores, C. Fernando. "Management and Communication in the Office of the Future," Ph.D. dissertation, University of California, Bereley, 1982.
Gardner, Howard. The Mind's New Science: A History of the Cognitive Resolution. New York: Basic Books, 1985.
Habermas, J"urgen. Communication and the Evolution of Society , trans. Thomas McCarthy. Boston: Beacon Press, 1979.
Haugeland, John. Mind Design. Cambridge: Bradford/MIT Press, 1981.
———. Artifical Intelligence: The Very Idea. Cambridge: Bradford/MIT Press, 1985.
Holt, Anatol. "Remarks Made at ARPA Principal Investigators' Conference," Los Angeles, February 6-8, 1974 (unpubl. ms.).
Howard, Robert. "Systems Design and Social responsibility: The Political Implications of 'Computer-Supported Cooperative Work,'" address delivered at the First Annual Conference on Computer-Supported Cooperative Work, Austin, Texas, December 1986.
Laird, John, Paul Rosenbloom, and Allen Newell. Universal Subgoaling and Chunking: The Automatic Generation and Learning of Goal Hierarchies. Hingham, Mass.: Klumer, 1986.
Lee, Ronald M. "Bureaucracy as Artifical Intelligence," in L. B. Methlie and R. H. Sprague, eds., Knowledge Representation for Decision Support Systems . New York: Elsevier (North-Holland), 1985. 125-132.
———. "Automating Red Tape: The Performative vs. Information Rolse of Bureaucratic Documents," Office: Technology and People 2 (1984): 187-204.
Lenat, Douglas. "CYC: Using Common Sense Knowledge to Overcome Brittleness and Knowledge Acquisition Bottlenecks," AI Magazine 6:4 (1986): 65-85.
March, James G., and Herbert A. Simon. Organizations. New York: Wiley, 1958.
Maturana, Humberto R., and Francisco Varela. The Tree of Knowledge. Boston: New Science Library, 1987.
Michie, Donald, and Rory Johnston. The Creative Computer. New York: Viking, 1984.
Minsky, Marvin. The Society of Mind. New York: Simon and Schuster, 1986.
Newell, Allen. "The Knowledge Level," Artificial Intelligence 18 (1982): 87-127.
Newell, Allen, and Herbert Simon. "Computer Science as Empirical Inquiry: Symbols and Search," Communications of the ACM 19 (March 1976): 113-126. Reprinted in J. Haugeland, ed., Mind Design , 35-66.
———. Human Problem Solving. Englewood Cliffs, N.J.: Prentice-Hall, 1972.
Newquist, Harvey P., III. "The Machinery of Medical Diagnosis," Ai Expert 2:5 (May 1987): 69-71.
Pylyshyn, Zenon. Computation and Cognition: Toward a Foundation for Cognitive Science. Cambridge: Bradford/MIT Press, 1984.
Roszak, Theodore. The Cult of Information: The Folklore of Computers and the True Art of Thinking. New York: Pantheon, 1986.
Rumelhart, David, and James MacLelland. Parallel Distributed Processing: Explorations in the Microstructures of Cognition. 2 vols. Cambridge: Bradford/MIT Press, 1986.
Russell, Bertrand. A History of Western Philosophy. New York: Simon and Schuster, 1952.
Simon, Herbert. Models of Thought. New Haven: Yale University Press, 1979.
Turkle, Sherry. "Romantic Reactions: Paradoxical Responses to the Computer Presence," chap. 11, this volume.
Waterman, Donald. A Guide to Expert Systems. Reading, Mass.: Addison-Wesley, 1986.
Weber, Max. Economy and Society: An Outline of Interpretive Sociology. Berkeley, Los Angeles, London: University of California Press, 1968.
Winograd, Terry. "A Language/Action Perspective on the Design of Cooperative Work," Human-Computer Interaction 3:1 (1987-88): 3-30.
Winograd, Terry, and Fernando Flores. Understanding Computers and Cognition: A New Foundation for Design. Norwood, N.J.: Ablex, 1986.
Eleven—
Romantic Reactions:
Paradoxical Responses to the Computer Presence
Sherry Turkle
Evocative Objects
In the eighteenth century, Newton's physics gave rise to a clockwork and rationalist view of human nature.[1] It also eventually gave rise to the opposite: a romantic reaction to considering reason as what made humans unique declared that sensibility was more important than logic, the heart more human than the mind.
Responses in our contemporary culture to the computer presence have a similar dialectical quality. Computers and computational ideas have entered popular thinking to support images of mind as mechanism, as program, as information processor. Yet even as these images have spread through the culture, they have provoked a new romantic reaction. When people use information-processing models to explain more and more of their behavior, they often feel compelled to isolate as "their core" something they can think of as privileged and undetermined, something fundamentally "beyond information." The very qualities of computers that foster mechanistic views of human nature also lead people to emphasize the importance of feelings, spontaneity, holism, sensuality, and spirituality in defining what is uniquely human. For some people, the appropriation and disavowal of computational images of the self take the form of a pendulum swing; for others, the competing views of the self exist simultaneously: there is no victory, only ambivalence.
I have been a student of these popular "romantic reactions" to the computer for over a decade.[2] I have reported that in the presence of the computer, there is a tendency for people to define themselves as what computers are not or cannot do. But defining people too starkly as what computers cannot do in terms of performance leaves one in a vulnerable
position, with definitions of what is unique in human nature trying to run ahead of what clever engineers might come up with next. This happened (to choose only one out of many examples) when in the early 1960s, philosopher Hubert Dreyfus insisted that a computer could not beat him at chess because chess required intuition and experience, a "knowing how" rather than a "knowing that." When a chess program was able to triumph over him (and other even more skilled players), Dreyfus had to retreat to the position that "real chess" was the kind of chess computers could not play.[3] With many such experiences of computer competency behind them, people have learned to look for a more modulated response. In my studies, I find that people begin by admitting that human minds are some kind of computer and then go on to find ways to think of people as something more as well.
Faced with "smart machines," professional philosophers respond by cataloging principles of human uniqueness. They find them in human intentionality, emotion, and biology. Faced with a computer that follows rules , philosophers see humans as unique because their knowledge is socially and physically situated, not rule based but embodied, concrete, and experiential.[4] These responses from a professional community are echoed in the popular culture. When confronted with a computer that in some ways seems to think like a person, people use everyday language to capture their sense of human uniqueness. They talk about feelings, flesh, intuition, spark, direct experience, or, as Joseph Weizenbaum summed it up, the things we know but cannot say, the wordless glance that a father and mother share over the bed of their sleeping child.[5]
People analogize mind to computer but then take care to undermine the analogy by attributing to people what one eighteen-year-old I interviewed called "a saving grace." In her mind, "that saving grace, the difference between people and computers, is emotion. And that's the line you can draw. That's where you say, this thing may be able to do something like thinking, but it can't love anybody." I have found this response widespread.
Since the mid-1970s, the media have touted the prowess of expect systems, the large computer programs that embody the information and rules that professionals use to make everyday decisions. With a general cultural understanding that rule-based, information-rich computers can do amazing things, many people have come to believe that there is such a thing as computer "cognition" and see information processing as a model for an important aspect of human rationality.[6] But often, at the same time, almost in defense, their sense of identity becomes increasingly focused on whatever they define as "not cognition," "beyond information." People concede to the rule-based computer some power of
reason and then turn their attention to the soul and the spirit in the human machine.
Most recently, however, the story of computers and "romantic reactions" has taken a dramatic new turn. Romantic reations to the computer are being met by a movement within the technical community which rejects programs and rules to focus on "emergent" intelligence. This movement puts forward new images of machines that bring together many of the characteristics the romantic reaction used to mark the boundary between people and mechanism. These images are of computation without programming, of machines that are unpredictable and undetermined. Such are the "romantic machines" of emergent artificial intelligence (AI). Emergent AI insists that it does not teach the computer but allows the machine to learn; it does not give the computer rules to follow but expects intelligence to emerge from the interaction of agents or entities within the computer system.
The guilding metaphors here are not logical but biological, not mechanistic but social. Theorists of a "connectionist" or "neural net" school of AI believe that if neuronlike entities in the machine are left to interact, they will organize themselves to produce intelligent behavior. They treat the computer as a black box that houses the emergent processes.[7] Their way of approaching computers is more like the one we use to approach people in everyday life. We do not need to know the specific rules of our human companions' behavior; we have a general feeling for how they do things and allow ourselves the pleasure of surprise. In a "society of mind" model, Marvin Minsky and Seymour Papert describe an inner world of "dumb" interacting agents. Complexity of behavior, emotion, and thought emerges from the cacophony of their opposing views, their conflicts and their compromises.[8]
A vision of emergent intelligence was present from the earliest days of AI, but for many years it went underground, leaving the field to a more rationalistic and "rule-driven" tradition, symbolic information processing.[9] But recently, the emergent tradition has resurfaced, with a vengeance. As Paul Smolensky put it, "In the past half-decade the connectionist approach to cognitive modeling has grown from an obscure cult claiming a few true believers to a movement so vigorous that recent meetings of the Cognitive Science Society have begun to look like connectionist pep rallies."[10] My studies show that in the popular culture, people are trying to describe human uniqueness in terms of what computers cannot do, while in the professional computer culture, scientists are starting to talk about machines in images ever closer to those we use to describe people. Now, both people and computers are presented as fundamentally "beyond information."
Thus, the scientists of emergent AI—a classification I use to include
connectionist (neural net) and society of mind models—are themselves part of the romantic reaction. Assimilating their research to a romantic reaction does not challenge the validity or importance of their mathematical methods. It is, however, an assertion about the appeal of the problem to which the mathematics is applied. While traditional AI represented throught as an essentially logical process, connectionism sees it as a natural phenomenon governed by mathematical laws in the same way that the rest of nature is. The influences that bear on this construction of the problem are not just technical but social, cultural, and psychological.
With the resurgence of emergent approaches to AI, the story of romantic reactions to the computer presence comes full circle. Images of the machine as unstructured and undetermined meet images of people as unstructured and undetermined, some of these put forth in response to computers in the first place. With this "effet de retour," the story of romantic reactions to the computer presence can no longer be told from one side as the story of people responding to their reflection in the mirror of the machine. There is movement through the looking glass.
The computer is a psychological machine, not only because it might be side to have its own very primitive psychology but also because it causes us to reflect on our own. Marginal objects play important roles in theory construction. On the line between categories, they draw attention to how we have drawn the lines and in the process call them into question.[11] Emerson said that "dreams and beasts are two keys by which we find out the secrets of our own nature. They are test objects."[12] Like dreams and beasts, the computer stands on the margins. It is mind and not yet mind; it is inanimate and yet interactive. It does not think, yet neither is it external to thought. It is poised between the thought and the thought of. As behaving systems, computers sit on the boundary between the physical and the psychological. In that position, they provoke thinking about the boundaries between matter, life, and mind.
From this perspective, which takes the computer as a test object, what I have called an "evocative object," the question is not whether we will ever build machines that think like people but whether people have always thought like machines.[13] And if this is the case, if we see ourselves as in some ways kin to the computer, are these the most important things about us? Is what we share with the computer what is most essential about being human?
Such questions have traditionally been the province of philosophers and psychologists. With the advent of personal computers in the 1970s, these questions moved out into the culture at large, carried by people's relationships with an object they could touch, use, and own. Computers became "objects to think with," carrier objects for thinking about the self, and this for two reasons.[14] First, as I have noted, computers are at the
margins. The machines do things—like interact and respond to queries—that provoke reflection on what it means to interact and respond. And if one of the things that makes you feel alive is "I think therefore I am," the computer's "almost" thinking is provocative. Second, although computers engage in complex behaviors, they present no simple window onto their inner structure. They are opaque. People use computers to reflect on the human. In this process of using machines to think about mind, what the machines can actually do is important, but how people think about them is more so. Thus, the story is being played out on the boundary, not between minds and machines but between ideas about minds and ideas about machines.
Transparency to Opacity/Physics to Psychology
When scientists speak about their childhood experiences, many refer to the intensity of their relationships with objects. For example, an MIT faculty member I shall call "Sandy" told of the many happy hours he had spent with a beloved broken radio.
I had no idea what any of the parts did: capacitors and resistors and parts I didn't know the names of, tubes that plugged in and things like that. What I did assimilate was that it was made of lots of pieces, and that the pieces were connected in special patterns, and that one can discover truth or something like that by looking through the patterns that connect this device. Obviously, some kind of influences flowed between these connections, and they sort of communicated. And you could see that the radio had lots of littler, simpler devices that communicated with each other so that the whole thing could work.
A lot of the parts came in little glass tubes. I could see through the glass. And some of them had different patterns than the others. So I could figure out that the parts came in different classes. And the different patterns could help you figure out the arrangements that they needed to communicate with each other. And it was a great thrill to have thought of that. I can remember that there was this tremendous charge I got from that, and I thought I would spend the rest of my life looking for charges just like that one.
Although Sandy never fixed the broken radio, several things happened to him in the course of working on it. He came to see himself as a special sort of person, the kind of person who is good with "things" and with "figuring things out." He came to understand the importance of interconnections and of breaking things down to simpler systems within a more complex device. And when he had such insights, Sandy felt an exhilaration to which he became addicted. Sandy's charges came not from external success but from his inner conviction that he had under-
stood something important. In other words, when we watch Sandy with his radio, we watch Sandy the child developing the intellectual personality of Sandy the scientist.
Scientists' recollections of their youth are highly subjective data. But there is another source of data for exploring the developmental role of objects as carriers of ideas: direct observation of children. I have observed many children who remind me of how Sandy describes himself at five.[15] Like Sandy, they puzzle about how things work; like him, they take things apart and theorize about their structure. The objects at the center of their play and theorizing include refrigerators (another of Sandy's childhood passions, the object to which he "graduated" after radios), air-conditioning machines, heaters, Legos, erector sets, model trains, motors, and bicycles. Notable for their absence are modern radios. The favored objects (let us call them "traditional objects") have a great deal in common with one another and with the four-tube radio that offered itself up to Sandy's fascinated observation.
The most significant thing they have in common is "transparency." An object is transparent if it lets the way it works be seen through its physical structure. The insides of a modern radio do not provide a window onto the patterns and interconnections of parts that so impressed Sandy. Sandy's portable four-tube radio was transparent. Its solid-state contemporary cousin is opaque.
With the words "solid-state," I have invoked the most dramatic example of the shift to opacity in the object world. Unlike Sandy's radio, integrated circuits have no easily visible "parts" from the point of view of the curious eye and the probing hand that are the only equipment children bring to such things. They hide their structure while performing miracles of complexity of function.
At five, Sandy could look inside his radio and make enough sense of what he saw to develop a fruitful theory about how it worked. But when you take off the back of an electronic toy or computer, all that the most persistently curious child finds is a chip or two, some batteries, and some wire. Sandy was able to use the radio to think with by thinking about its physical structure. He could make it his own not only because he could see it but because he could work it in his head, drawing on a coherent system of thought that was part of his child knowledge. This was knowledge of physical movement. Sandy spoke of some unknown "stuff" moving through the connections between the different parts of the radio. He says he thought of the stuff as influences, and as he got older, he learned to think of the influences as current.
Computers (taken as physical objects) present a scintillating surface, and the "behavior" they exhibit can be exciting and complex, but there is no simple, mechanical way to understand how they work. They are
opaque. If their "behavior" tempts one to anthropomorphize, there is no way to "open the hood," to "pull off the cover," and reassure oneself that mechanism stands behind. When children try to think about the physical working of the computer, they find themselves with little to say. ("It's the batteries," they tell me, frustrated at finding themselves at a dead end.) When this happens, children turn to a way of understanding in which they have more to say—a psychological way of understanding. In doing so, they draw on another kind of child knowledge than that which Sandy used, knowledge not of the body and its motion but of the mind and its thought and emotion. Sandy was able to use the radio to approach the ways of thinking of physical science through his knowledge of how people move (the influences and their connections). Contact with computational objects leads children to approach psychological ways of thinking through their intuitive knowledge about how people think.
In sum, children use computers as "objects to think with" by identifying with them as psychological machines. One nine-year-old, Tessa, made this point very succinctly in a comment about the chip she had seen when her computer was being serviced. "It's very small, but that does not matter. It doesn't matter how little it is, it only matters how much it can remember." The physical object is dismissed. The psychological object becomes the center of attention and elaboration. The development of this kind of discourse begins with much younger children. There, the computer enters into how they sort out the metaphysically charged questions to which childhood must give a response.
When, in the 1920s, Swiss psychologist Jean Piaget first studied what children thought was alive and not alive, he found that children home in on the concept of life by making finer and finer distinctions about the kind of physical activity that is evidence of life. For the very young child, said Piaget, everything active may be alive: the sun, the moon, a car, the clouds. Later, only things that seem to move on their own without anybody giving them a push or a pull can be seen as alive. And finally, the concept of "motion from within" is refined to include a notion of subtle life activity: grwoth, metabolism, and breathing become the new criteria.[16]
Children build their theories of what is alive and what is not alive as they build all other theories. They use the things around them: toys, people, technology, the natural environment. Children today are confronted with highly interactive computer objects that talk, teach, play, and win. Children are not always sure whether these should be called alive or not alive. But it is clear, even to the youngest children, that physical movement is not the key to the puzzle. The child perceives the relevant criteria not as physical or mechanical but as psychological. For example, in the case of a computer game that plays tic-tac-toe, is it aware, is it conscious, does it have feelings, even, does it cheat?[17]
Children use psychology to talk about the aliveness of inanimate things other than computers. One five-year-old told me that the sun is alive "because it has smiles." Another said that a cloud is alive "because it gets sad. It cries when it rains." But if an eight-year-old argues that clouds or the sun are alive, the reasons given are almost always related to their motion—their way of moving across the sky or the fact that they seem to do so of their own accord. In contrast, as children become older and as they become more sophisticated about computers, their arguments about the computer's aliveness or lack of aliveness become focused on increasingly refined psychological distinctions. The machines are "sort of alive" because they think but do not feel, because they learn but do not decide what to learn, because they cheat but do not know they are cheating.
Piaget told a relatively simple story that accounted for increasing sophistication about the question of aliveness through the development of notions of physical causality. My observations of children discussing the aliveness of computers agree with those of many investigators who stress the greater complexity of children's animistic judgments. For example, in Conceptual Change in Childhood , Susan Carey demonstrates that alongside the development Piaget traces, something else is going on: the development of biological knowledge.[18] Children's encounters with computers underscore the importance of a third area of relevant knowledge: psychological knowledge and the ability to make psychological distinctions. Carey discusses how children develop an intuitive psychology that is prebiological, a way of interpreting biological aspects of life as aspects of human behavior. (As when a child answers the question, "Why do animals eat?" by saying that "they want to" rather than they must "eat to live.") When I refer to children's responses about the nature of the computer's aliveness as psychological, I am talking about something else—aspects of psychology that will not be replaced by a biological discourse but will grow into mature psychological distinctions, most significantly, the distinction between thought and feeling.
The computer evokes an increasingly sophisticated psychological discourse just as Carey points out that involvement with and discussion about animals evokes a more developed biological discourse. Noncomputational machines—for example, cars and telephones—entered into children's thinking but in essence did not disturb the "traditional" pattern. Cognitive psychologists Rochel Gelman and Elizabeth Spelke say why. There is a great difference between "traditional machines" and computers. "A machine may undergo complex transformations of states that are internal and unseen. But it lacks the capacity for mental transformations and processes." In their work, "for purposes of exposition," they "disregard or set aside modern man-made machines that mimic in one
way or more the characteristics of man."[19] Their intuition that the computer would be disruptive to their expository categories is correct. The marginal computer does upset the child's constructed boundaries between thing and person.
A long tradition of Western science has drawn a line between the worlds of psychology and physics and has tried, at least programmatically, to take the laws of motion of matter as the fundamental handle for grasping the things it found in the physical world. Sandy's investigation of the radio and Piaget's reports of how children used motion to sort out the question of what is alive in the world of traditional objects showed them conforming to this way of thinking. Tessa's summary comment of what "matters" when you try to understand a chip ("that it remembers") does not conform. Nor do scenes of children discussing whether a computer is alive by cataloging its qualities of consciousness, its degree of intentionality, whether or not it "cheats."[20]
In the present context, what is most important is that the new psychologized language for talking about the machine world influences how children think about people. The discussion of the computer's cheating leads them into a discussion of intentions: people have them, computers do not. Discussion about the computer's origins leads them to distinctions between free will and programming: people think their own ideas, computer ideas are put in by their makers. Some children move from this distinction to another between rote thinking, which computers are said to do, and creative thinking, which they do not.
Among such distinctions, perhaps the most important is how children isolate the cognitive from the rest of psychology. While young children tend to throw together such undifferentiated observations as that a computer toy is happy, smart, or gets angry, by the ages of nine and ten, children comfortably manipulate such ideas as "the computer thinks like a person—does the math things you know—but it doesn't have feelings," or "the computer can't dream, only people can," or "the computer only does things that can come out in typing."
The position toward which children tend as they develop their thinking about people in relation to computers is to split psychology into the cognitive and the affective: the psychology of thought and of feeling. It is no longer that something has a psychology or it does not. While younger children often say that a computer or computer toy is alive "because it has feelings," older children tend to grant that the machine is intelligence and is thus "sort of alive" but then distinguish it from people because of its lack of feelings. Confronted by a personlike machine, a machine that plays on the boundary, people want to make the boundary firmer.
Romantic Selves
Traditionally, children came todefine what was special about people in contrast to what they saw as their nearest neighbors, the animals: their pet dogs, cats, and horses. Pets have desires, but what stands out dramatically about people is how intelligent they re, their gifts of speech and reason. Computers upset this distinction. The machine presents itself to the child as a thing that is not quite a thing, a mind that is not quite a mind. As such, it changes the way children think about who are their nearest neighbors. The Aristotelian definition of man as a "rational antimal" (powerful even for children when it defined people in contrast to their nearest neighbors, the animals) gives way to a different distinction.
Today's children appropriate computers through identification with them as psychological entities and come to see them as their new nearst neighbors. And they are neighbors that seem to share in or (from the child's point of view) even excel in our rationality. Children stress the machines' prowess at games, spelling, and math. People are still defined in contrast to their neighbors. But now, people are special because they feel. Many children grant that the computer is able to have a "kind of life," or a "sort of life," but what makes people unique is the kind of life that computers do not have, an emotional life. If people were once rational animals, now they are emotional machines. This is their romantic reaction.
As children tell it, we are distinguished from machine intelligence by love and affection, by spiritual urges and sensual ones, and by the warmth and familiarity of domesticity. In the words of twelve-year-old David, "When there are computers who are just as smart as people, the computers will do a lot of the jobs, but there will still be things for the people to do. They will run the restaurants, taste the food, and they will be the ones who will love each other, have families and love each other. I guess they'll still be the ones who go to church." Or we are distinguished from the machines by a "spark," a mysterious element of human genius.
Thirteen-year-old Alex plays daily with a chess computer named Boris which allows its human user to set its level of play. Although Alex always loses if he asks the computer to play its best game, Alex claims that "it doesn't feel like I'm really losing." Why? Because as Alex sees it, chess with Boris is like chess with a "cheater." Boris has "all the most famous, all the best chess games right there to look at. I mean, they are inside of him." alex knows he can study up on his game, but Boris will always have an unfair advantage. "It's like in between every move Boris could read all the chess books in the world." Here, Alex defines what is special about being a person not in terms of strengths but in terms of a certain frailty.
For this child, honest chess is chess played within the boundaries of human limitations. His heroes are the great chess masters whose skill depends not only on "memorizing games" but on "having a spark," a uniquely human creativity.
In my studies of adults in the computer culture, I have found that many follow essentially the same path as do children when they talk about human beings in relation to the new psychological machines. This path leads to allowing the possibility of unlimited rationality to computers while maintaining a sharp line between computers and people by taking the essence of human nature to be what computers cannot do. The child's version: the human is the emotional. The adult's version, already foreshadowed in Alex's formulation of the human "spark": the human is the unprogrammable.
Few adults find it sufficient to say, as did David, that machines are reason and people are sensuality, spirituality, and emotion. Most split the human capacity for reason. Then, the dichotomy that David used to separate computers and people becomes a way to separate aspects of human psychology. One student speaks of his "technology self" and his "feelings self," another of her "machine part" and her "animal part."
Or, people talk about those parts of the psyche that can be simulated versus those parts that are not subject to simulation, frequently coupled with the argument that simulated thinking is real thinking, but simulated feeling is not real feeling. Simulated love is never love. Again, this is a romantic response, with the computer making a new contribution to our formulas for describing the divided self. We have had reason and passion, ego and id. Now, on one side is placed what is simulable; on the other, that which cannot be simulated, that which is "beyond information."
The Romantic Machines of Emergent AI
This, of course, is where new directions in artificial intelligence become central to our story. Because emergent AI presents an image of the computer as fundamentally beyond information .
For years, AI was widely identified with the intellectual philsoophy and methodology of information processing. Information-processing AI has roots in mathematician George Boole's intellectual world, in logic.[21] It relies on the manipulation of propositions to obtain new propositions and the combination of concepts to obtain new concepts. But artificial intelligence is not a unitary enterprise. It is a stuff out of which many theories can be fashioned. And beyond information processing, there is emergent AI.
Emergent AI is indissociable from parallel computation. In a traditional, serial computer, millions of units of information sit in memory
doing nothing as they wait for the central processor to act on them, one at a time. Impatient with this limitation, the goal of emergent AI is "pure" computation. The whole system is dynamic, with no distinction between processors and the information they process. In some versions of emergent AI, the processors are neuronlike entities connected in networks; in others, they are anthropomorphized societies of subminds. In all cases, they are in simultaneous interaction. The goal, no less mythic in its proportions than the creation of a strand of DNA, is the generation of a fragment of mind. From the perspective of emergent AI, a rule is not something you give a computer but a pattern you infer when you observe the machine's behavior, much as you would observe a person's.
The two AIs have fueled very different fantasies of how to build mind out of machine. If information-processing AI is captured by the image of the knowledge engineer, hungry for rules, debriefing the human expert to embody that expert's methods in algorithms and hardware, emergent AI is captured in the image of the computer scientist, "his young features rebelling, slipping into a grin not unlike that of a father watching his child's first performance on the violin," running his computer system overnight so that the agent within the machine will create intelligence.[22]
The popular discourse about emergent intelligence tends to stress that the AI scientists who work in this paradigm set up experiments in the computer and let them run, not knowing in advance what the interactions of agents within the system will produce. They stress the drama and the suspense.
To train NETalk to read aloud, Sejnowski had given his machine a thousandword transcription of a child's conversation to practice on. The machine was reading the text over and over, experimenting with different ways of matching the written text to the sound of spoken word. If it got a syllable right, NETalk would remember that. If it was wrong. NETalk would adjust the connections between its artificial neurons, trying new combinations to make a better fit. . . . NETalk rambles on, talking nonsense. Its voice is still incoherent, but now the rhythm is somewhat familiar: short and long bursts of vowels packed inside consonants. It's not English, but it sounds something like it, a crude version of the nonsense poem "The Jabberwocky." Sejnowski stops the tape. NETalk was a good student. Learning more and more with each pass through the training text, the voice evolved from a wailing banshee to a mechanical Lewis Carroll.[23]
Such descriptions of neural net "experiments" reflect the AI scientists' excitement in doing what feels like "real" laboratory science rather than running simulations. But at the same time that they are excited by the idea of AI as an experimental science, they are drawn to the mystery and unpredictability of what is going on inside of the machine. It makes their material seem more lifelike.
We are far from the language of data and rules that was used to describe the large expert systems of the 1970s. The agents and actors of emergent AI programs are most easily described through anthropomorphization. Take, for example, a very simple case of emergent intelligence, the perceptron, a pattern-recognition machine designed in the late 1950s. In the perceptron, inner agents, each of whom has a very narrow decision rule and access to a very small amount of data, essentially "vote." The perceptron weights their voices according to each agent's past record of success. It is able to take advantage of signals saying whether it has guessed right or wrong to create a voting system where agents who have guessed right get more weight. Perceptrons are not programmed but learn from their own "experiences." In an information-processing system, behavior follows from fixed rules. The perceptron has none. What is important is not what an agent knows but who it knows, its place in a network, its interactions and connections. While information processing begins with formal symbols, perceptrons operate on a subsymbolic and subformal level.
In the brain, damage seldom leads to complete breakdown. It usually leads to a degradation of performance proportional to its extent. Perceptrons show the graceful degradation of performance that characterizes the brain. With disabled "voter-agents," the system still works, although not as well as before. This connection with the brain is decisive for the theorists of the most successful of the trends in emergent AI: connectionism or neural nets.
In the early 1960s, the atmosphere in AI laboratories was heady. Researchers were thinking about the ultimate nature of intelligence. The goal ahead was almost mythic: mind creating mind. Perceptrons and perceptronlike systems had their successes and their adherents as did information-processing approaches with their attmepts to specify the rules behind intelligence, or in Boole's language, the "laws of thought."
But for almost a quarter of a century, the pendulum swung away from one computational aesthetic and toward another, toward rules and away from emergence. In its influence on spcyhology, AI became almost symonymous with information processing. Allen Newell and Herbert Simon posited that the human brain and the digital computer shared a level of common functional description. "At this level, both the humna brain and the appropriately programmed digital computer could be seen as two different instantiations of a single species of device—a device that generated intelling behavior by manipulating symbols by means of formal rules."[24] Newell and Simon developed rule-based systems in their purest form, systems that simulated the behavior of people working on a variety
of logical problems. The method nd its promise were spelled out in the Newell and Simon physical symbol system hypothesis:
A physical symbol system has the necessary and sufficient means for general intelligent action. By necessary we meant that any system that exhibits general intelligence will prove upon analysis to be a physical symbol system. By sufficient we meant that any physical symbol system of sufficient size can be organized further to exhibit general intelligence.[25]
Thus, simulations of what came to be called "toy problems" promised more: that mind could be built out of rules. But the ideas of information processing were most successful in an area where they fell far short of building mind. This was in the domain of expert systems. With the worldly success of expert systems in the 1970s, the emphasis was taken off what had been most mythic about the AI of the 1950s and early 1960s and placed on what computer scientists had learned how to do with craftsman's confidence—gather rules from experts and code them in computer programs.
However, I have noted that in the late 1970s and early 1980s, the pendulum swung again. There was new, powerful, parallel hardware and new ideas about how to program it. The metaphors behind programming languages shifted. They were no longer about lists and variables but about actors and objects. You could think about traditional programming by analogies to the step-by-step instruction of a recipe in a cook-book. To think about the new object-oriented programming, the analogie had to be more dynamic: actors on a stage. With these changes came a rebirth of interest in the concept of neural nets, reborn with a new capturing mnemonic, connectionism.
More than anyone else, Douglas Hofstadter captured the aesthetic of the new movement when he spoke about computation "waking up from the Boolean dream."[26] For connectionists, that dream had been more like a nightmare. Like the romantics, connectionists sought to liberate themselves from a constraining rationalism of program and rules. They take pride in the idea that the artificial minds they are trying to build have an aspect that, if not mystical, is at the very least presented as mysterious. From the point of view of the connectionists, a certain amount of mystery fits the facts of the case.
We cannot teach an information-processing computer the rules for most aspects of human intelligence that people take for granted because we simply do not know them. There is no algorithm for recognizing a face in a crowd. The connectionists approach this state of affairs with a strategy made possible by the new availability of massively parallel computing: build a computer that at least in some way looks like a brain and
make it learn by itself. Unlike symbolic information processing, which looked to programs and specified locations for information storage, the connectionists do not see information as being stored "anywhere" in particular. Rather, it is stored everywhere. Information is better thought of as "evoked" than "found."[27] The computer is treated as a black box that houses emergent processes.
There is an irony here. The computer presence was an important influence toward ending the behaviorist hegemony in American psychology in the late 1970s. Behaviorism forbade the discussion of inner states or entities. One could not talk about memory, only the behavior of "remembering." But the fact that computers had memory and inner states provided legitimation for discussing people as having them as well. Behaviorism presented mind as a black box. Information processing opened the box and filled it with rules, trying to ally itself as closely as possible with commonsense understandings. But this was a vulnerability from the point of view of nonprofessionals who were then exposed to these understandings. They seemed too commonsense. People had to be more than information and rules. Now connectionism closes the box again. What is inside these more opaque systems can once again be thought of as mysterious and indeterminate.
Philosopher John Searle exploited the vulnerability of information-processing models when he pursued a thought experiment that took as its starting point the question of what might be going on in a computer that could "speak Chinese." Searle, who assures us that he does not know the Chinese language, asks us to imagine that he is locked in a room with stacks and stacks of paper, say, index cards. He is given a story written in Chinese and then is passed slips of paper on which are written questions about the story, also in Chinese. Of course, he does not know he has a story, and he does not know that the slips of paper contain questions about the story. What he does know is that "clever programers" have given him a set of rules for what to do with the little pieces of paper he is passed. The rules tell him how to match them up with other little pieces of paper that have Chinese characters on them, which he passes out of the room. The rules say such things as "The squiggle-squiggle sign is to be followed by the squoggle-squoggle sign."[28] He becomes extraordinarily skillful at following these rules, at manipulating the cards in his collection. We are to suppose that his instructions are sufficiently complete to enable him to "output" chinese characters that are in fact the correct answers to the questions about the story.
All of this is set up for the sake of argument in order to ask one rhetorical question in plain English: Does the fact that he sends out the correct answers prove that he understands Chinese? For Searle, it is clear that the answer is no.
. . . I can pass the Turing test for understanding Chinese. But all the same I still don't understand a word of Chinese and neither does any other digital computer because all the computer has is what I have: a formal program that attaches no meaning, interpretation, or content to any of the symbols.[29]
In the end, for Searle, the system is only a "paper shuffler." He described the innards of the machine in terms so deeply alien to the ways most people experience the inner workings of their minds that they felt a shock of "nonrecognition." For may people, it fueled a sense that Searle had captured what always seemed wrong with AI. The disparity between the description of the paper shuffler and one's sense of self supported the view that such a systme could not possibly understand the meaning of chinese in the same sense that a person does.
Connectionism is less vulnerable to the Searlean argument. Its models postulate emergence of thought from "fuzzy" process, so opening up the box does not reveal a crisply defined mechanism that a critic can isolate and make to seem psychologically implausible. Connectionsits only admit just enough of a view onto the inside of their system to create a general feeling for its shape. And for many people, that shape feels right—in the way that Searle made rule-driven systems feel wrong. That shape is resonant with brainlike processes: associations and networks. Perhaps for these grown-up AI scientists, neural nets have something of the feel that the four-tube radio had for Sandy the child. The theoretical objects "feel right," and the theory does not require that they be pinned down to a high degree of specificity. And similar to Sandy, these scientists' "charges," their sense of excitement and achievement, depends not only on instrumental success but on the sense of being in touch with fundamental truths. In this, the connectionists, like Sandy, are romantics-in-practice.
Winograd worked as a young scientist in a very different intellectual culture, the culture of symbolic AI. This is the culure of which Dreyfus could say, "any domain must be formalizable." And the way to do AI is to "find the context-free elements and principles and to base a formal, symbolic representation on this theoretical analysis."[30] In other words, the goal is a limpid science, modeled on the physical sciences, or as Winograd put it, "We are concerned with developing a formalism, or 'representation,' with which to describe . . . knoweldge. We seek the 'atoms' and 'particles' of which it is built, and the 'forces' that act on it."[31] From the point of view of this intellectual aesthetic of transparency, the connectionist black box is alien and unacceptable. In other terms, the classic confronts the romantic.
Indeed, referring to the connectionist's opaque systems, Winograd has said that people are drawn to connectionism because it has a high percentage of "wishful thinking."[32] Perhaps one could go further. For
connectionism, wishful thinking is a point of method. They assert that progress does not depend on the ability to specify process. In other words, for connectionists, computers can have the same "opacity" as the brain. Like the brain, they are boxes that can remain closed; this does not interfere with their functioning as models of mind.
Societies of Mind
Winograd has made a similar point about the wishful thinking in another branch of emergent AI that has come to be known as the "society theory" of mind.[33] Marvin Minsky and Seymour Papert have built a computational model that sees the mind as a society of interacting agents that are highly anthropomorphized, discussed in the terms one usually reserves for a whole person. But their agents do not have the complexity of people. Instead, the society theory is based (as is the idea of perceptrons) on these agents being "dumb." Each agent has a severely limited point of view. Like the voting agents in a perceptron model, this narrowness of vision leads them to different opinions. Intelligence emerges from their interactions and negotiations. In its construction of a highly anthropomorphized inner world, society theory uses a language about mind that is resonant with the object-relations tradition in psychoanalytic thinking.[34]
In classical psychoanalytic theory, a few powerful inner structures (e.g, the superego) act on memories, thoughts, and wishes. Object relations theory posits a dynamic system in which the distinction between processed and processor breaks down.[35] The parallel with computation is clear: in both cases, there is movement away from a situation in which a few inner structures act on more passive stuff. Psychoanalyst W. R. D. Fairbairn replaced the Freudian dichotomy of ego and id, structure and drive energy with independent agencies within the mind who think, wish, and generate meaning in interaction with one another.
The Minsky-Papert computational model evokes the world of Fairbairn. In the case of both psychoanalysis and society theory, a static model (on the one hand, based on drive and on the other, based on information) is replaced by an active one. A model that seems mechanistic and overly specified as something that could encompass the human psyche is replaced by a model with a certain mystery, a model of almost chaotic interactions. The most elaborated presentation of the society model is Minksy's The Society of Mind .[36] There, instead of what Winograd describes as the "finely tuned clockwork" of information-processing AI, we get an "impressionistic pastiche of metaphors." In a microworld of toy blocks, Minsky describes how agents that at first blush seem like simple computational subroutines work together to perform well-defined tasks like building
towers and tearing them down. But then comes the wishful thinking, what Wingorad here calls the "sleight of hand."[37] Minsky speculates how, within an actual child, the agents responsible for BUILDING and WRECKING might become versatile enough to offer support for one another's goals. In Minsky's imaginative text, they utter sentences like, "Please WRECKER, wait a moment more till BUILDER adds just one more block: it's worth it for a louder crash."[38] winograd points out that Minsky has moved from describing the agents as subroutines to speaking of them as entities with consciousness and intention. And his reaction is sharp:
With a simple "might indeed become versatile," we have slipped from a technically feasible but limited notion of agents as subroutines, to an impressionistic description of a society of homunculi, conversing with one another in ordinary language. This sleight of hand is at the center of the theory. It takes an almost childish leap of faith to assume the modes of explanation that work for the details of block manipulation will be adequate for understanding conflict consciousness, genius, and freedom of will.
One cannot dismiss this as an isolated fantasy. Minsky is one of the major figures in artificial intelligence and he is only stating in a simplistic form a view that permeates the field.[39]
Winograd attributes the sleight of hand o Minsky's faith that since we are no more than "protoplasm-based physical symbol systems, the reduction must be possible and only our current lack of knowledge prevents us from explicating it in detail, all way from BUILDER's clever play down to the logical circuitry." In other words, Winograd attributes the sleight of hand to Minsky's belief in the physical symbol system hypothesis. In interpret it somewhat differently. Minsky's leap of faith, his confidence in the power of the agents, is based on his belief—one that I see at the core of the romantic reaction in artificial intelligence—that the interaction of agents causes something to emerge beyond what is possible through the manipulation of symbols alone.
Winograd's critique reflects his model of what Minsky's audience wants to hear, of the culture Minsky is writing to. Winograd bleieves that they want to hear a vindication of physical symbols, that they want to hear that symbols and rules will ultimately tell the story. The way I see it, Minsky's audience, like theaudience for connctionism, wants to hear precisely the opposite. In the 1970s, the culture of AI enthusiasts was committed to a rule-based model, but now things have changed. Both professionals and the lay public are drawn to soceity theory and to connectionism not because they promise specificity but because they like
their lack of specificity. We. Daniel Hills, designer of the "Connection Machine," a massively parallel computer, puts the point well:
It would be very convenient if intelligence were an emergent behavior of randomly connected neurons in the same sense that snowflakes and whirlpools are emergent behaviors of water molecules. It might then be possible to build a thinkign machine by simply hooking together a sufficiently large network of artificial neurons. The notion of emergence would suggest that such a network, once it reached some critical mass, would spontaneously begin to think.
This is a seductive idea because it allows for the possibility of constructing intelligence without first understanding it . Understanding intelligence is difficult and probably a long way off, so the possibility that it might spontaneously emerge from the interactions of a large collection of simple parts has considerable appeal to the would-be builder of thinking machines.
. . . Ironically, the apparent inscriutibility of the idea of intelligence as an emergent behavior accounts for much of its continuing popularity.[40]
The strength of object theories in both psychoanalysis and artificial intelligence is a conceptual framework that offers rich possibilities for models of interactive process; the weakness, which is of course what Wingorad is pointing to, is that the framework may be too rich. The postulated objects may be too powerful when they epxlain the mind by postulating many minds within it.[41] Object theory confronts both fields with the problem of infinite regress, but Minsky is writing to a professional audience that has sensitized itself to its heady possibilities. Computer scientists are used to relying on recurison, a controlled form of circular reasoning; it would not be going too far to say that some have turned it into a transcendent value. For douglas Hofstadter, computation and the power of self-reference weave together art, music, and mathematics into an eternal golden braid that brings coherency and a shared aesthetic to the sciences and the arts. His vision is, to say the least, rmantic. In Gödel, Escher, Bach , he speaks of it as a kind of religion.[42] Hofstadter's brilliant and accessible writing has done a great deal to legitimate the circularity implicit in accounting for the entities that are then to acocunt for thought in the wider culture.
In the 1970s, Minsky said, "The mind is a meant machine." the phrase became famous. During my research program in the late 1970s and early 1980s, I interviewed hundreds of people in both the technical and lay cultures about their beliefs about computers, about how smart the machines were then and how smart they might someday be. In these conversations, Minsky's words were often cited as an example of "what was wrong" with AI. They provoked irritaiton, even disgust. I believe they seemed unacceptable not so much because of their substance but because of then-prevalent images of what kind of machine the mind's meat ma-
chine might be. These images were mechanistic. But now, a source of connectionism's appeal is precisely that it proposes an artificial meat machine, a computational machine made of biological resonant components. And with a changed image of the machine, the idea that the mind is one becomes far less problematic.
Even those who were most critical of rule-driven AI are somewhat disarmed by emergent models that turn away from rules and toward biology. Dreyfus, for example, had criticized smybolic information-processing AI in terms of a broader critique of a purely syntactic, representational view of the relation of mind to reality. Pointing to the work of Martin Heidegger and the later Ludwig Wittgenstein, he made the point that "Both these thinkers had called into question the very tradition on which symbolic information processing was based. Both were holists, both were struck by the importance of everyday practices, and both held that one could not have a theory of the everyday world."[43] Now, he sees neural networks in a position to prove them right and him as well:
If multilayered networks succeed in fulfilling their promise, researchers will have to give up the conviction of Descartes, Husserl, and early Wittgenstein that the only way to produce intelligent behavior is to mirror the world with a formal theory in mind. . . . Neural networks may show that Heidegger, later Wittgenstein and Rosenblatt [an early neural net theorist] were right in thinking that we behave intelligently in the world without having a theory of that world.[44]
While cautionus, Dreyfus is receptive to the technical possibilities and philosophical importance of connectionist research in a way that he never was toward traditional AI. Similarly, cultural critics such as Leo Marx, long skeptical about the impact of technology on liberal and humanistic values, have found the "contextual, gestaltist, or holistic theory of knowledge implicit in the connectinist research program" to be "particularly conductive to acquiring complex cultural understanding, a vital form of liberal knowledge."[45] Although the sympathetic response here is less to connectionism than to its metaphors, his warm feelings, like those of Dreyfus, show how connectionism has new possibilities for intellectual imperialism. Connectionism has received good press as a more "humanistic" form of AI endeavor, with the stress on its respect for the complexity and mystery of mind.
Thinking of Yoursel As an Anti-Lovelace Machine
In a memoir she wrote in 1842, Lady Ada Lovelace, a friend and patroness of Charles Babbage, inventor of the "analytical engine," was the first person to go on record with a variant of the often-quoted phrase, "The
computer only does what you tell it to do, nothing more, nothing less."[46] Most people are used to thinking of computers with the "Lovelace" model. It caputres the aesthetic of information processing.[47] But it does not speak to emergent AI. For emergent AI, the point is precisely to make computers do more than they were programmed to do, indeed, in a certain sense, not to have to program them at all. It has become commonplace to quote Lovelace to object to the possibility of AI. "People are not computers. The don't follow rules. They learn. They grow." But to the degree that emergent AI is characterized by "anti-Lovelace" representations of the computer, it breaks down resistance to seeing continuity between computers and people by describing computers that learn and grow. And it describes computers whose working are mysterious.
In emergent AI, the effort is to have surpring global complexity emerge from local simplicity. Even in the small neural net programs that can run on standard personal computers, watching such phenomena emerge can have the "feel" of watching life. Indeed, one of the first computer demonstrations that played on this idea was a program called the "GAME OF LIFE." Patterns grow, change, and evolve before the eye of the beholder. It is not surprising that AI scientists use such system to study evolutionary phenomena. This kind of evolving, "unfolding" machine does not seem to "offend" in the same way as did its programmed cousin. One MIT hacker I interviwed told me that if you make the system complicated enough, for example, the simultaneous operation of a society of millions of interacting programs, you might be able to create in a machine "that sense of surprising onself . . . that makes most people feel that they have free will, perhaps even . . . a soul." Another told me that "with the idea of mind as society, Minsky is trying to create a computer complex enough, indeed beautiful enough, that a soul might want to live in it." If you romanticize the machine in this way, you have a new chance to see the machine as like the human and the human as like the machine.
If you see computers as Lovelace machines, people are the opposite of computers, or if they are to be analogized with computers, the human psychology that emerges is resolutely mechanistic. But connectionists insist that computation should not be reduced to specifications, and although the adherents of society models are more committed to one day being able to specify what is within the black box, that day is far off and the theory need not wait. Emergence offers an appealing retort to the Lovelace objection. For Hills, "emergence offers a way to believe in physical causality while simultaneously maintaining the impossibility of a reductionist explanation of thought. For those who fear mechanistic explanations of the human mind, our ignorance of how local interactions produce emergent behavior offers a reassuring fog in which to hide the sould."[48]
Connectionist machines, romantic machines whose emergent properties can "hide the soul," have a cultural resonance in our time of wide-spread disaffection with instrumental reason. For Papert, speculating on the identity of the "Prince Charming" who awoke the sleeping connectionism, a shift of cultural values has created a more welcoming milieu for connectionist ideas. The new resonance depends on a "generalized turn away from the hard-edged rationalism of the time connectionism last went into eclipse and a resurgent attraction to more holistic ways of thinking."[49]
For years I have documented how people construct romantic images of mind when confronted with the image of the computer as an information-processing machine. Today there is a more complex picture as people also confront increasingly romantic images of the machine.
When sociobiology presents an image of the human as a "bundle of genes," people rebel. They listen to the theory, and they say that is "not me." When AI presents an image of the human as a "bundle of programs," there is the same "not me" response, the same reaction that was exploited by Searle with powerful rhetorical effect. When programs became refined as "heuristic" (defined by rules of thumb rather than rules), the response softens, but the idea of people as a "bundle of heuristics" does not go far enough for people to accept such systems as a reflection of who they are. But the idea that "I" am a bundle of neuronlike agents that communicate with one another and whose intelligence emerges from their interactions sounds enough like how we have always thought about the brain to start to feel comfortable, like "me."
The mathematical theory of connectionism might not be any more accessible to lay thinking than technical papers on information processing; indeed, their advanced mathematics usually makes them less so. But when object- and agent-oriented theories are popularized and move out into the general culture, they have special appeal, the direct appeal of actors on a stage. At one time or another, we all have the sense of not feeling "at one" with ourselves: inner voices offer conflicting advice, reassurance, and chastisements. These experiences are easily and satisfyingly translated into a drama of inner objects. The objects and agents of emergent AI are engaging because they are concrete; it is easy to "play" with them. They are resonant with popular understanding of Freud that represent ego, superego, and id as competing inner actors. Like Freud's trio, the agents provide a way to interpret our common experiences of inner conflict. The rational bias of our culture presents consistency and coherency as natural, but, of course, feelings of fragmentation abound. Indeed, it has been argued that they characterize contemporary psychological life.[50] And so, theories that speak to the experience of a divided self have particular power.
Thus, emergent AI is a seductive theory for many reasons. It presents itself as escaping the narrow determinism of information processing. Its images are biologically resonant. The language of "neural nets" is inexact but metaphorically effective, presenting mind processes as the kinds of things that could be going on in a brain. Its constituent agents offer a theory for the felt experience of multiple inner voices. Like "fuzzy logic" or "chaos," two other ideas that have captured the popular and professional imagination, emergent AI speaks to our technological society's disappointments with the cold, sharp edges of formal logic. It is resonant with a general turn to "softer" epistemologies that emphasize concrete methodologies over algorithmic ones in the sciences as well as the arts.[51] It is resonant with a criticism of traditional Western philosophy, which, for Heidegger, is defined from the start by its focusing on facts in the world while "passing over" the world as such.[52]
And finally, to come full circle, the constituent agents of emergent AI offer almost tangible "objects to think with." In a paradoxical but comforting way, they get one back to a version of Sandy's experience with the four-tube radio. They try to explain the world to us in terms of familiar interactive systems, in terms of "parts" that do this or that to one another, and in terms of physics. The connection strengths that are posited between the neuronlike entities can be translated into the simple physics of moving things closer together and farther apart.
Freudian ideas about slips of the tongue became well known and gained acceptance for reasons that had little to do with assessments of their scientific validity. Slips are almost tangible ideas. They are manipulable. Slips are appealing as objects to think with. You can analyze your own slips and those of your friends. As we look for slips and start to manipulate them, both seriously and playfully, psychoanalytic ideas, at the very least the idea that there is an unconscious, start to feel more natural. The theory of slips allowed psychoanalytic ideas to weave themselves into the fabric of everyday life. They made the theory more concrete and appropriable. Similarly, people like to think about the ideas behind mind as connection and mind as society. To take the simplest case (which is the only case that matters when you look for the "whys" behind a theory's cultural popularity), you can imagine and play with Minsky's ideas of agents by taking on their personae. As in the case of psychoanalytic models, you can imagine yourself in the role of the psychic entities, and acting out these roles feels enough like an acting out of the theory to give a sense of understanding it. One may forget the details of each theory, but they offer experiences of theoretical play that break down resistance to seeing mind as irrational or seeing mind as machine. Romantic machines pave the way for the idea of mind as machine to become an acceptable part of everyday thinking.
In their reaction to new science and technology, the romantics split passion from reason, the sensuous from the analytic. T. S. Eliot, reflecting on the loss of the ability to integrate "states of mind and states of feeling," called it the "dissociation of sensibility."[53] Information processing led to the position that if computers closely reseble people in their thinking, they differ from them in their lack of feeling. It, too, set the stage for a dissociation of sensibility. The information-processing paradigm and the consequent reaction to it deepened the gulf between affect and cognition. Within the academic world, this movement was both reflected and reinforced in the emergence of a new, computationally inspired, branch of psychology: cognitive science.
The Freudian experience has long ago taught us that resistance to a theory is part of its cultural impact. Resistance to psychoanalysis with its emphasis on the unconscious led to an emphasis on the rational aspect of human nature, to an emphasis on people as logical beings. Resistance to a computational model of people as programmed information systems led to a new articulation of the position that what is essential in people is what cannot be captured in language, rules, and formalism. This resistance, in a form that I have called "romantic," put positive emphasis on the importance of aspects of human nature that are not simulable—on love, human connection, and sensuality—and on the importance of the embodiment of human knowledge within individuals and social groups. But it tended to reinforce a too-radical split between affect and cognition, between the psychology of thought and the psychology of feeling. When thought and feeling are torn from their complex relationship with each other, the cognitive can become reduced to mere logical process and the affective to the visceral. What was most powerful about Freud's psychological vision was its aspiration to look at thought and feeling as always existing together, at the same time and in interaction with each other. In psychoanalytic work, the effort is to explore the passion in the mathematician's theorem and the reason behind the most primitive fantasy. The unconscious has its own, highly structured language that can be deciphered and analyzed. Logic has an effective side, and affect has a logic. The too-easy acceptance of the idea that computers closely resemble people in their thinking and differ only in their lack of feeling supports a dichotomized and oversimplified view of human psychology.
We might hope that the theorists of emergent machine intelligence will further our appreciation and understanding of the human integration of thought and feeling. We must fear that they are not. Indeed, while we feared before that the human would be reduced to the analytic through neglect of feelings, the inclusion of feelings in AI models raises its own problems. The point is dramatized by the way Minsky appropriates Oedipus. In The Society of Mind , Minsky looks at the Oedipus com-
plex through its computational prism: "If a developing identity is based upon that of another person, it must become confusing to be attached to two dissimilar adult 'models.'"[54] Thus, "Oedipus" intervenes, a mechanism designed to facilitate the construction of a simple-enough agent by removing "one [of the models] from the scene."[55]
With this scenario, Minsky has entered a domain of human experience where pure information processing did not often care to wander. The tenor of his theory—romantic, loose, emergent—makes him confident that he can build agents that represent complex human attributes. But when he does so, he turns the Oedipal moment (traditionally thought of in terms of jealousy, sexuality, and murderous emotion) into a problem closer to cognitive dissonance. A discussion of primitive feelings is turned into a discussion of a kind of thinking. Information processing left affect dissociated; emergent AI may begin to integrate it only to leave it reduced.
We must acknowledge a profound change in our cultural images of the machine. The question remains: What will its effect be on our images of people?
Through the mid-1980s, many who criticized the computer as the embodiment of rationality feared that it would lead people to a view of themselves as cold mechanism. In fact, we have seen that resistance to images of computation as information processing led to a resurgence of romantic ideas about people: what made them special was all that the analytical engine was not. Now we face an increasingly complex situation.
For the foreseeable future, emergent machine intelligence will exist in only the most limited form. But already, there is widespread confidence in its intellectual and economic importance and familiarity with elements of its language, its way of talking about the problem of mind. This language provides a way to talk about machines which makes them seem more like people. It serves as a mediator between the computer culture and the culture of mind in a way that the language of information processing never could. The language of neurons, connections, associations, agents, and actors makes it easier to talk about oneself as "that kind" of a machine. The similarities in the language of computational "society" models and object-relations psychoanalysis makes the new models seem resonant with the concerns of a professional culture widely perceived as least hidebound by narrow logicism. And finally, I have noted that with the move away from rules and toward biology, philosophical critics seem disarmed in their traditional objections. In its simplest form, arguing that people do not think "linearly" is no longer an argument for why parallel machines are not "really" thinking.
Through such intellectual detours, romantic machines may have the
effect that critics feared from rational ones. Resistance to the rational machines fostered a romantic view of people. The romantic machines offer a way for people to accept the idea of the human as a meat machine. But this acceptance comes at a high cost. John Searle has argued that thought is a product of our specific biology, the product of a human brain.[56] When Searle talks about human biology, he means neurons and the chemistry of the synapse, as when he speculates that "as far as we know, the functional aspect of the neuron is the nondigital variation in ]its] rate of firing."[57] But our specific biology means more than having a brain just as it means more than being made of meat. Our specific biology places us in the human life cycle: we are born, nurtured by parents, grow, develop sexually, become parents in our turn. And we die. This cycle brings us the knowledge that comes from understanding the certainty of loss, that those we love will die and so will we. In these ways, we are alien to a being that is not born of a mother, that does not feel the vulnerability of childhood, that does not know sexuality or anticipate death. We may be machines, but these experienced aspects of our biology (the consequences of there being meat in our meat machines) compel a search for transcendence. People look for it in religion, history, art, the relationships through which we hope to live on.
Emergent AI does not offer us minds that are born of mothers, grow up in families, or know the fear of death. When AI offered a rational and rule-driven machine, it led to a romantic reaction. Current romanticizations of the machine may lead to a rationalistic one: a too-easy acceptance of the idea that what is essential about human beings can be captured in what makes us kin to mechanism.
Twelve—
Biology, Machines, and Humanity
Stuart Hampshire
In addressing the subjects of biology and humanity as well as machines and humanity, I feel the necessity of mentioning human bodies, which often get lfet out although they are among the rather conspicuous facts about human beings, that they possess them. The philosophy in which I was largely brought up, Anglo-American empiricist philosophy, sometimes seems a prolonged conspiracy to overlook the fact that human beings have bodies and that we live in them and, ost important of all, that we exist because of them. We often know what our bodies are doing and what is going on inside them from sources other than the five senses that we use to observe objects other than our own bodies. These sources of knowledge about bodily movement and action are more intimate, because internal, sources. If we did not know without observation that we were moving our eyes and our hands, we would not know by observation about external things. I said all this some years ago in Thought and Action[1] but not sufficiently aggressively, I now think.
The overwhelming and obvious lack of any significant similarity between machines of any kind, as far as we know, and human beings, is due to the fact that machines do not have bodies. I believe they have frames or mainframes. This limits their minds. The more the intellect's calculative abilities and the memories of machines are developed and increased, the less, in certain respects, they become like human beings. That is why they are sometimes spoken of, not unreasonably, as inhuman. When I hear that they are regularly defeating the human grand masters at chess (and we have been waiting for some years for this to happen), I shall know that they have become even less human. You may remember a very brilliant novel by Nabokov about chess masters that brought up the human aspects of successful chess playing. An increase in machines' intellectual powers
and their powrs of coherent memory might correctly be described, in summary fashion, as an increase in their powers of mind. There is nothing incorrect about that. But these words might be misleading if they were taken to imply that their minds as a whole had been developed and their total powers of mind increased.
The fact is that just two commonly distinguished powers of mind, distinguished for practical and social purposes, would have been abstracted from the huge amalgam that constitutes the mind and then been further developed and increased. As was stressed, both by Terry Winograd and Sherry Turkle, all sorts of humanly interesting mental powers and dispositions would remain at zero. I am thinking of the dispositions and powes associated with sexuality, with all that sexuality entails in family relationships and emotional attachments, the enjoyment of sports and of games of skill that are played outside and are less abstract than chess, of sculpture, of dancing, of mimicry (as opposed to reproduction), of dramatic performance, of inappropriate and sudden laughter, or groans and scowls out of season and not on cue, of tones of voice in speech and in singing. Obviously, I could continue this list indefinitely. I have not mentioned the pleasures of thirst, cultivated thirst and controlled intoxication, of conviviality and of old-fashioned greed, which was once mentioned charmingly by one of Sherry Turkle's twelve-year-old subjects. One could go on. Yet the competition between man and machines remains.
The important thing about machines is that they have remarkably copious and definite memories—efficient, clear, and unconfused—which we do not. Together, this makes up a fairly small part of what we should properly include under the title of mind and not necessarily the most interesting or productive part. If we take a long historical view of culture, and of a person's individuality, it is a matter of the expression in an eye or the way a person walks or stands or speaks; it is half physical. No one who has seen it will forget the way that a certain dancer's neck, a Russian dancer, Ulanova, fitted on to her head when she danced. It was quite unforgettable. The style and expression (I do not mean feeling) were unique. Think of the tainted history of the word reason, pictured as master to slave, as in Plato, or upside down, as in Hume, where it is subject to another total and hopeless abstraction called feeling, reason being the slave. The history of the word reason is tainted because Aristotle began by associating powers of reason with the doctrine of the Scala Universi, the great Chain of Being, which puts reason at the top, near to God the Father, or God the Holy Ghost in the sky, our animal nature lower down the scale of being, and the "trancelike life of plants" just above the sticks and stones. This picture of the great Chain of Being is just a pleasing and rather self-glorifying fiction, which models God's creation, as has been several times remarked, on a properly stratified social order, and the social order is
indeed a human creation. This picture is a denial of complexity of living things and of the many different powers of development that can be traced in nature, all in the interest of some form of social tidiness.
If there is no clear view of God at the top of the ladder, or perhaps a little above it, it is not clear what is higher and lower in the order of human powers and dispositions. Nor is it clear why we should think in terms of scales at all. There is no valid reason, it seems to me, to place the noncomputational, or nonstorage, or nonlogical, aspects of the mind lower than so-called pure reason, apart from the dreams of the disembodied survival of the soul, which the myth of pure reason preserves. If we forget this recurring, haunting dream of an ideal Manichaeanism, we can insist on the equal priority in the human mind of imagination, of physical play, of poetry, painting, and the performing arts, in the definition of humanity. We can think of individuality in handwriting and script. We ought not, it seems to me, to have this academic competition of higher or lower. Maybe, for some ulterior moral argument, one picks out distinctive human powers and dispositions where we could probably look better, compared with other animal species, or we may think of the suprprisingly variable and unstructured nature of human sexuality, which leads to various family structures. Second, since we learn an enormous variety of natural languages, variety within the species is important and distinctive, as is the peculiarly confused store of our memories.
Let me also address memories, because they came up in Roger Hahn's essay and elsewhere. Ours are confused in comparison with the machine's. In an interesting and productive way, human beings, compared with other animals, are obsessed with their past, as Bernard Williams has remarked. Not merely their cwn past but their family's past, the tribe's and nation's past. These various pasts are lodged in their minds both as individuals and as tribes or nations, as a mixture of history and myth, truth and falsity. Memories are blurred (at any rate, mine is), smudged with the vast complicated network of uplanned, uniwue, and largely unconscious associations in the process of condensation of memory.
Assuming an individual's memories are an inextricable and innovative muddle, an interfusion of elements, forming and reforming as experience goes on in a person's life. When the muddle becomes sufficiently rich and many layered, like a compost heap, and when reason has been kept in its place, we call the muddled memory "imagination." However, when machines have impaired memories, they become "defective," and we are stuck with them, and they do not breed as compost does.
Hahn proposed a certain revision of standard histories of science in relation to the scientific revolution. He drew a contrast between the role of mechanical devices, particularly in the sixteenth and seventeenth centuries, which are plentifully illustrated in the books of the time (the illus-
trated new concepts of physical understanding), and, equally important, a new confidence in human abilities. If you understand it, you can make it, and often you can convert that proposition and say you can make it if you can understand it. This principle is referred to as the verum factum principle. it introduced a notion of causality connected with experimental action, with doing something in contrast to mere observation: the recording in theeye, of what the eye sees or receives. Now the Platonic and hence Peripatetic traditional had put the recording eye, not the ingenious manipulator, on top of the scale of value, and Plato, as is often remarked, had always used metaphors of vision to explain what the acquisition of knowledge is. The ingenious manipulator was a craftsman, lower on the social scale. As Keller points out, the terms that we use, such as competition, are derived from new social realities. There is an interesting difference that Hahn brought out, because the eye is, according to Hegel, the most ideal of the human senses, which means it is the least bodily part of the body, the least, as it were, closely material, the most expressive, the nearest to the mind through being nearest to the brain. We have this interesting view of true empiricism as being the doctrine that we can reconstruct natural creatues, and to reconstruct is to understand. It is not to have a map of a possible reconstruction but an actual reconstruction that is most enlightening about the nature of understanding.